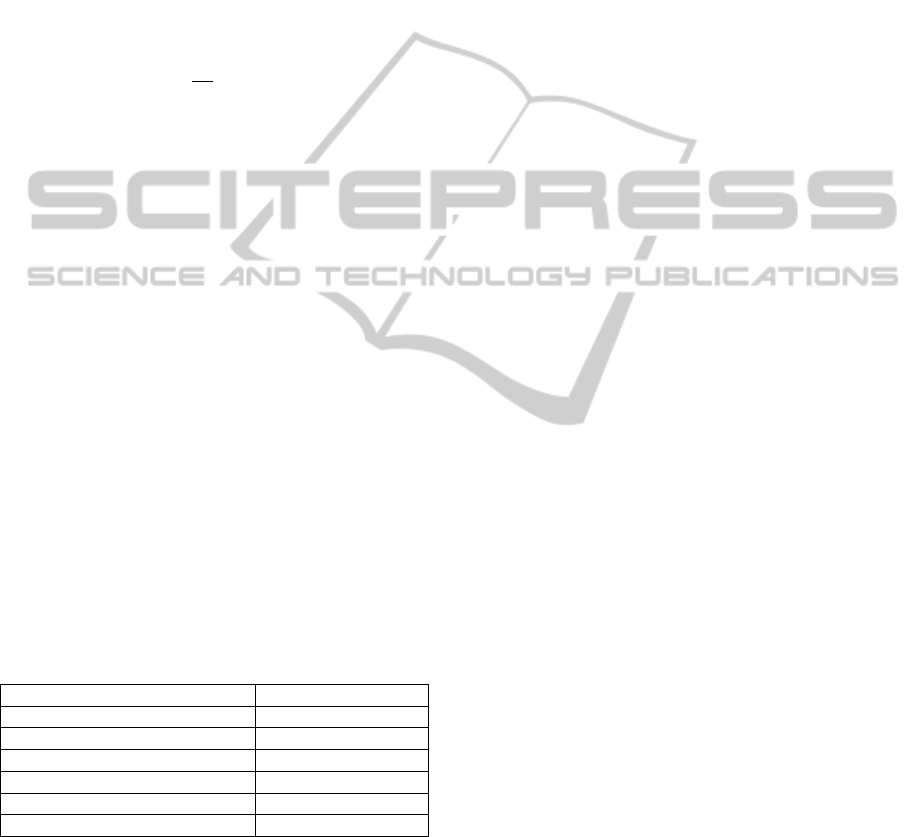
position because the influence function in LMS
criterion is linearly with the size of its error.
Among several methods, which deal with the
outlier problem, M-estimator techniques (Huber,
1984) are the most robust and have been applied in
many applications. M-estimators use some cost
functions which increase less rapidly than that of
least square estimators as the residual departs from
zero. When the residual error increases over a
threshold, M-estimators suppress the response
instead. This work employs Welsch M-estimator
function as the error function, given by
(12)
where
is a scale parameter. The cost function of
RBF network
Eq. (7) can be rewritten as
(13)
where
is one of the parameter sets of the network.
According to the gradient descent method, the
update equation for the network parameters (11) also
can be derived according to (13).
According to the M-estimator behaviour, the
modified RBF networks are able to eliminate the
influence of outliers. In this way, the classification
performance can be improved.
3 EXPERIMENTAL RESULTS
This research uses the Facial Recognition
Technology (FERET) (Phillips, 1998) database to
evaluate the performance. We select 600 frontal face
images from the FERET database. There are 300
images for training and other images for testing.
Table 1: Comparison of other methods.
Methods Accuracy (%)
Shan, C. [14] 94.81
Yuchun, Fang [15] 92.16
Qiu, Huining [17] 92.45
Mehmood, Y. [18] 94
Our method (M-estimator RBF) 94.7
Our method (Traditional RBF) 91.02
To investigate the performance of the PCA
dimensionality reduction, different dimensionalities
are performed which are ranged from 10 to 130
dimensions. The best accuracy rate of the proposed
method achieves 94.7% while the dimensionality is
60, and the number of neurons in RBF network is set
to 12. A comparison of other methods is listed in
Table 1. On the other hand, the table also shows that
the result of our method using traditional RBF
network is only 91.02 % accuracy. It demonstrates
the tolerance to outliers of M-estimator.
4 CONCLUSIONS
This research proposes three types of effective
features, including facial texture features, hair
geometry features, and mustache features, to
perform the gender classification. These features
cover the global, local, geometry, and texture
properties. We also design an M-estimator based
RBF neural network to classify the gender. The
experimental results show that the proposed method
produces a good performance.
ACKNOWLEDGEMENTS
We thank the National Science Council (Grant
number: NSC 102-2221-E-155 -070) for funding
this work.
REFERENCES
Alexandre, L. A., 2010. Gender recognition: A multiscale
decision fusion approach. Pattern Recognition Letters,
31, 1422-1427.
Moghaddam, B. and Ming-Hsuan, Y., 2000. Gender
classification with support vector machines. In
Proceedings of the Fourth IEEE International
Conference on Automatic Face and Gesture
Recognition, 306-311.
Len, B. et al, 2011. Classification of gender and face based
on gradient faces. In Proceedings of the 2011 3rd
European Workshop on Visual Information Processing
(EUVIP), 269-272.
Ueki, K. et al., 2004. A method of gender classification by
integrating facial, hairstyle, and clothing images. In
Proceedings of the 17th International Conference on
Pattern Recognition, 446-449.
Viola, P. and Jones, M., 2001. Rapid object detection
using a boosted cascade of simple features. In
Proceedings of the 2001 IEEE Computer Society
Conference on Computer Vision and Pattern
Recognition, I-511-I-518.
Stegmann, M. B. et al., 2003. FAME-a flexible appearance
modeling environment. IEEE Transactions on Medical
Imaging, 22, 1319-1331.
Chng, E. S. et al., 1996. Gradient radial basis function
networks for nonlinear and nonstationary time series
prediction. IEEE Trans. Neural Networks, 7(1), 190-
194.
2
2
/exp1
2
)(
nnW
rr
)()(
nW
rEJ
GenderClassificationUsingM-EstimatorBasedRadialBasisFunctionNeuralNetwork
305