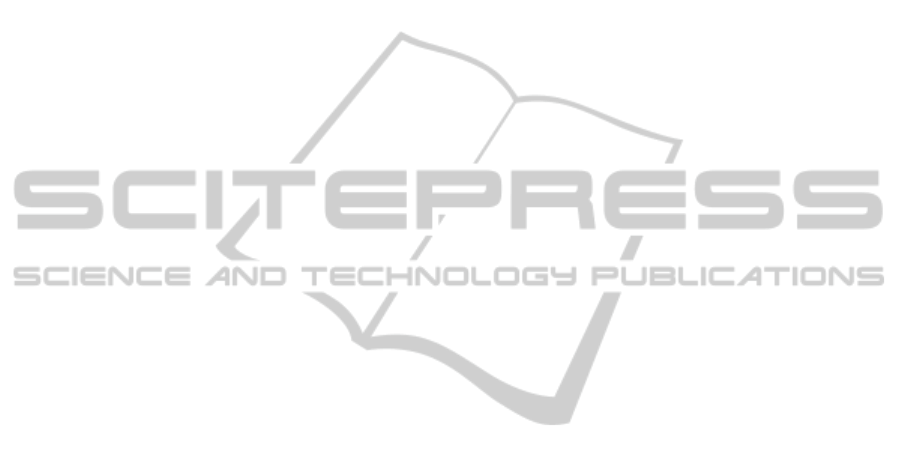
functions (0.575 against 0.766, 0.520 against 0.736,
and 0.545 against 0.736, respectively).
4 CONCLUSIONS
In our previous studies, we have investigated the use
of smartphone accelerometers to estimate road
surface roughness condition (IRI) in which
promising results are observed. This study continues
to explore the use of more smartphone sensors,
including the accelerometers and gyroscopes, to
estimate IRI with the final goal of obtaining an
improved estimation model that is acceptably
accurate, simple and easy to implement. An
experiment is carried out to obtain data from the
smartphone relevant sensors. After the data is
processed, FFT is used to calculate the magnitudes
of the vibration. Similar to the findings in our
previous studies, IRI can also be modelled as a
linear function of the average speed and the
magnitudes, calculated from both accelerometers
and gyroscopes. The function can be used to
estimate IRI with an improved accuracy in
comparison to the function that only considers the
average speed and the magnitudes from the
accelerometers alone, which is presented in the
previous studies. The new estimation function is
potentially useful for the development of a
smartphone app, which may contribute to improve
the efficiency of road authorities and government in
obtaining needed data, and monitoring as well as
maintenance planning of the road infrastructure.
In our ongoing studies, more focus is being put
into the formulation, piloting, and improvement of
the final and practicable estimation model.
Additionally, in our future work, great emphasis will
also be directed to the integration of the model into a
smartphone app.
REFERENCES
AndroSensor, 2012. [Smartphone App]. Google Play
Store. Available at: https:// play.google.com/store/
apps/details?id=com.fivasim.androsensor&hl=en.
BumpRecorder, 2013. [Smartphone App]. http://
www.bumprecorder.com/ [Accessed 15 July 2013]
Douangphachanh, V., Oneyama, H., 2013a. A Study on
the Use of Smartphones for Road Roughness
Condition Estimation, Proc. Of the Eastern Asia
Society for Transportation Studies, P297, Vol.9, 2013;
and J. Eastern Asia Society for Transport Studies, Vol.
10, 1551-1564.
Douangphachanh, V., Oneyama, H., 2013b. Using
Smartphones to Estimate Road Pavement Condition,
Paper presented at the 1
st
International Symposium for
Next Generation Infrastructure, Wollongong, NSW,
Australia, October 1-4.
Douangphachanh V., Oneyama, H., 2013c. Estimation of
Road Roughness Condition from Smartphones under
Realistic Settings, Proc. Of the 13
th
International
Conference on ITS Telecommunication (ITST), pp.
433-439.
Eriksson, J., Girod, L., Hull, B., Newton, R., Madden, S.,
Balakrishnan, H., 2008. The pothole patrol: using a
mobile sensor network for road surface monitoring,
Paper presented at the Sixth International Conference
on Mobile System, Applications and Services,
Breckenridge, Colorado, United States, June 17-20.
González, A., O’brien, E. J., Li ,Y. Y., Cashell, K., 2008.
The use of vehicle acceleration measurements to
estimate road roughness. Vehicle System Dynamics,
46(6), 483–499.
Mednis, A., Strazdins, G., Zviedris, R., Kanonirs, G.,
Selavo, L., 2011. Real time pothole detection using
Android smartphones with accelerometers, Paper
presented at the 2011 International Conference on
Distributed Computing in Sensor Systems, Barcelona,
Spain, June 27-29.
Mohan, P., Padmanabhan, V.N., Ramjee, R., 2008.
Nericell: Rich Monitoring of Road and Traffic
Condition using Mobile Smartphones. Proceedings of
the 6
th
ACM Conference on Embedded Network Sensor
Systems, 323-336.
Perttunen, M., Mazhelis, O., Cong, F., Kauppila, M.,
Leppänen, T., Kantola, J., Collin J., Pirttikangas, S.,
Haverinen, J., Ristaniemi, T., 2011. Distributed road
surface condition monitoring using mobile phones.
Ubiquitous Intelligence and Computing, 64–78.
Roadroid. 2014. [Smartphone App]. http://
www.roadroid.se/ [Accessed 9 December 2013]
Sayer, M. W., Gillespie, T. D., Queiros, C. A. V., 1986.
International Road Roughness Experiment. The World
Bank. Available at: http://deepblue.lib.umich.edu/
bitstream/handle/2027.42/3134/72773.pdf;jsessionid=2D5
5BF78AABCA31452E6A59CD28D17C1?sequence=2
[Accessed 12 September 2012]
Strazdins, G., Mednis, A., Kanonirs, G., Zviedris, R.,
Selavo, L., 2011. Towards Vehicular Sensor Networks
with Android Smartphones for Road Surface
Monitoring, Paper presented at the 2
nd
International
Workshop on Networks of Cooperating Objects,
Chicago, USA, April 11.
Tai, Y., Chan, C., Hsu, J. Y., 2010. Automatic road
anomaly detection using smart mobile device, Paper
presented at the 2010 Conference on Technologies and
Applications of Artificial Intelligence, Hsinchu,
Taiwan, November 18-20.
VIMS., 2012a. Vehicle Intelligent Monitoring System,
Bridge and Structure Laboratory, the University of
Tokyo, Japan.
VIMS., 2012b. VIMS Manual, VIMS Consortium,
Nagasaki University, Japan.
ExploringtheUseofSmartphoneAccelerometerandGyroscopetoStudyontheEstimationofRoadSurfaceRoughness
Condition
787