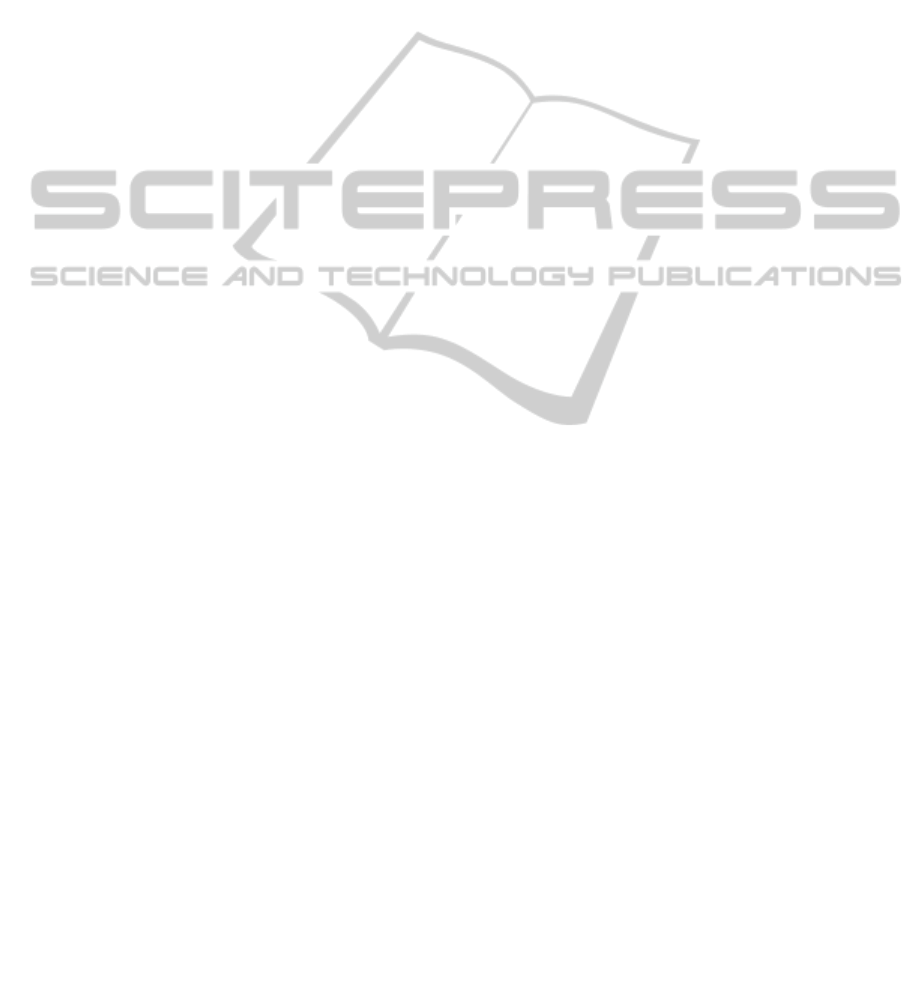
accelerometer at the trunk, it is known that the
greater they are, the greater is the risk and trend of
fall (Doheny et al. 2012). In Table 1, it is shown that
the crutches present the higher values, so these
devices are of higher risk to the user and the RFS
produced the lower values, except for RMS AP.
Considering the AP signal, it corresponds to the
forward and backward movement of the trunk. One
can see in Figure 5 a) that for the three devices the
signal is positive, meaning that the trunk is leaning
forward when the patient walks. However, the signal
is much higher for the RFS than the others ADs.
This happens because the RFS has to be pushed and
by observation of the authors, users had to lean
forward while pushing this device. This factor can
be due to an incorrect walker height adjustment.
Thus, the AP signal can be an important indicative
for posture correction as well as walker height
adjustment. Therefore, further studies will be
conducted to evaluate this potential clinical
indicator. In terms of ML signal in Figure 5 b), the
RFS presented the lower values, relatively to the
other ADs. The ML movement is a little attenuated
by the RFS and this happens because the user is
supported by the forearm support of RFS, preventing
the trunk oscillation in this direction.
Finally, relatively to the variability of the signals
of the trunk, it is shown in Table 1, that crutches
present a higher standard deviation for all of the
parameters, except for the sway range ML and RMS
AP. The first is higher for the standard walker. The
latter is higher for the RFS as expected, because of
the leaning of the trunk to the front.
To conclude, the authors see the RFS as the best
device for these patients since it provides higher
stability to the users, less risk of fall, a more natural
gait and a continuous movement.
5 CONCLUSION
This work used accelerometers located at the injured
leg’s ankle and trunk to verify and validate the
association between the accelerations signals and the
gait events, detect gait parameters and assess the fall
risk in assisted-gait with crutches, standard walker
and RFs. Further, it was possible to determine
efficiently all the proposed gait parameters in all
devices with patients diagnosed with KOA.
Additionnaly, it can be verified that the RFS
provides a greater stability, reducing the risk of fall
and inducing a more natural gai performance.
ACKNOWLEDGEMENTS
This work has been supported by FCT – Fundação
para a Ciência e Tecnologia in the scope of the
project: PEst-OE/EEI/UI0319/2014.
REFERENCES
Debi, R. et al., 2011. Correlation between single limb
support phase and self-evaluation questionnaires in
knee osteoarthritis populations. Disability and
rehabilitation, 33(13-14), pp.1103–9.
Doheny, E. P. et al., 2012. Displacement of centre of mass
during quiet standing assessed using accelerometry in
older fallers and non-fallers. Annual International
Conference of the IEEE Engineering in Medicine and
Biology Society. 2012, pp.3300–3.
Elbaz, A. et al., 2012. Can single limb support objectively
assess the functional severity of knee osteoarthritis?
The Knee, 19(1), pp.32–5.
Henriksen, M. et al., 2004. Test – retest reliability of trunk
accelerometric gait analysis. Gait & Posture, 19,
pp.288–297.
Kaufman, K. R. et al., 2001. Gait characteristics of
patients with knee osteoarthritis. Journal of
biomechanics, 34(7), pp.907–15.
Kloos, A. et al., 2012. The impact of different types of
assistive devices on gait measures and safety in
Huntington’s disease. PloS one, 7(2), p.e30903.
Lee, J.-A. et al., 2010. Portable activity monitoring system
for temporal parameters of gait cycles. Journal of
medical systems, 34(5), pp.959–66.
Martins, M. et al., 2013. Assessment of walker-assisted
human interaction from LRF and wearable wireless
inertial sensors. In Internation Congress on
Neurotechnology, Electronics and Informatics.
Neurotechnix. pp. 1–8.
Mathie, M. J. et al., 2004. Accelerometry: providing an
integrated, practical method for long-term, ambulatory
monitoring of human movement. Physiological
Measurement, 25(2), pp.R1–R20..
Sabatini, A. M. et al., 2005. Assessment of Walking
Features From Foot Inertial Sensing. IEEE transactions
on bio medical engineering, 52(3), pp.486–494.
Vaughan, C., Davis, B. & Connor, J. C. O., 1999.
Dynamics of Human Gait 2nd ed. C. Vaughan, ed.,
Cape Town, South Africa: Kiboho Publishers.
Watanabe, T. et al., 2011. A preliminary test of
measurement of joint angles and stride length with
wireless inertial sensors for wearable gait evaluation
system. Computational intelligence and neuroscience,
2011, p.975193.
DetectionofGaitEventsandAssessmentofFallRiskUsingAccelerometersinAssistedGait
793