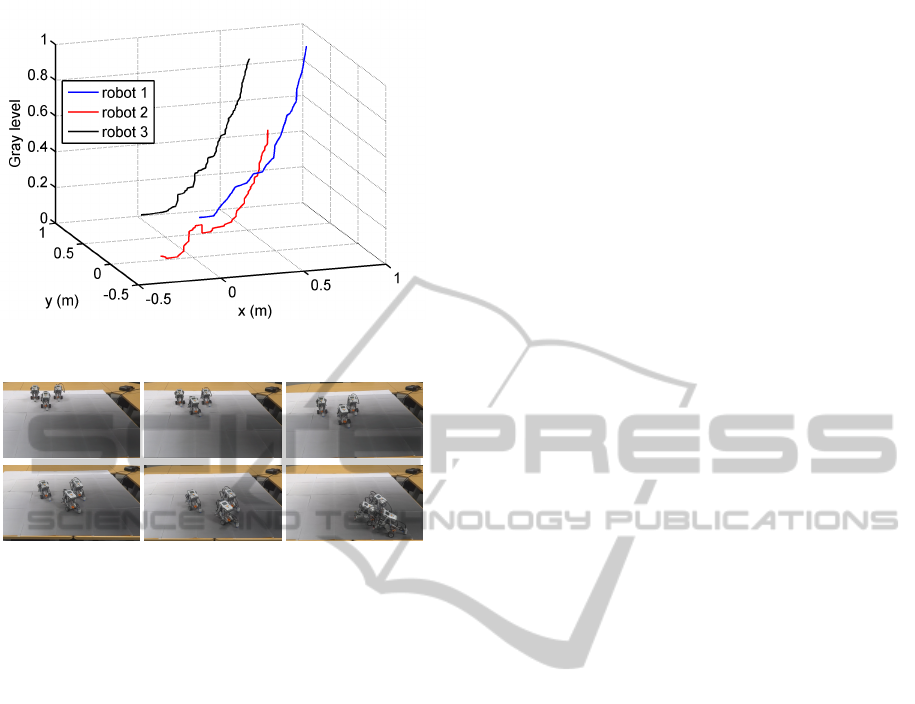
Figure 5: Fleet trajectory toward maximum, with measured
potential level.
Figure 6: Sequence of fleet convergence to the maximum.
5 CONCLUSIONS AND
PERSPECTIVES
The experiments reported in this paper have high-
lighted the possibility to control a fleet of Lego Mind-
storms NXT to fulfill two types of missions with these
autonomous vehicles, notwithstanding their compu-
tational capabilities. A first scenario has shown that
MPC can be a flexible solution to deal with fleet
management and collision avoidance between vehi-
cles and with obstacles. An efficient discretization
strategy allows the MPC to find an efficient control
sequence within constrained time. In a second sce-
nario, a decentralized estimation and control scheme
to find the maximum of a potential field has been
presented. It involved linear parameter estimation to
obtain the gradient of the field and a gradient-ascent
control proven to converge to the actual maximum lo-
cation. Implementation of the two strategies on the
fleet of Lego Mindstorms NXT was successful, which
shows the interest of these platforms as a practical
testbed for cooperative estimation and control under
strict implementation constraints.
ACKNOWLEDGEMENTS
The authors would like to thank Guillaume Broussin
and Mathieu Touchard, who contributed to these ex-
periments during their internship at ONERA.
REFERENCES
Benedettelli, D., Casini, M., Garulli, A., Giannitrapani, A.,
and Vicino, A. (2009). A Lego Mindstorms experi-
mental setup for multi-agent systems. In Proceedings
of the IEEE Multi-conference on Systems and Control,
Saint Petersburg, Russia, pages 1230–1235.
Canale, M. and Brunet, S. C. (2013). A Lego Mindstorms
NXT experiment for model predictive control educa-
tion. In Proceedings of the European Control Confer-
ence, Zurich, Switzerland, pages 2549–2554.
Costa, P., Moreira, A., Gonçalves, J., and Lima, J. (2011).
Proposal of a new real-time cooperative challenge in
mobile robotics. In Proceedings of the 18th IFAC
World Congress, Milan, Italy.
Dunbar, W. and Murray, R. (2006). Distributed receding
horizon control for multi-vehicle formation stabiliza-
tion. Automatica, 42:549–558.
Maze, N., Wan, Y., Namuduri, K., and Varanasi, M. (2012).
A Lego Mindstorms NXT-based test bench for cohe-
sive distributed multi-agent exploratory systems: Mo-
bility and coordination. In Proceedings of the AIAA
Infotech@Aerospace, Garden Crove, California.
Murray, R. (2007). Recent research in cooperative control
of multivehicle systems. Journal of Dynamic Systems,
Measurement, and Control, 129(5):571–583.
Pinto, M., Moreira, A. P., and Matos, A. (2012). Localiza-
tion of mobile robots using an extended Kalman fil-
ter in a Lego NXT. IEEE Transactions on Education,
55(1):135–144.
Rochefort, Y., Bertrand, S., Piet-Lahanier, H., Beauvois, D.,
and Dumur, D. (2012). Cooperative nonlinear model
predictive control for flocks of vehicules. In Proceed-
ings of the IFAC Workshop on Embedded Guidance,
Navigation and Control in Aerospace, Bangalore, In-
dia.
Scattolini, R. (2009). Architectures for distributed and hi-
erarchical model predictive control–a review. Journal
of Process Control, 19(5):723–731.
Scokaert, P. O. M., Mayne, D. Q., and Rawlings, J. B.
(1999). Suboptimal model predictive control (feasibil-
ity implies stability). IEEE Transactions on Automatic
Control,, 44(3):648–654.
Valera, Á., Vallés, M., Marín, L., and Albertos, P. (2011).
Design and implementation of Kalman filters applied
to Lego NXT based robots. In Proceedings of the 18th
IFAC World Congress, Milan, Italy.
ICINCO2014-11thInternationalConferenceonInformaticsinControl,AutomationandRobotics
610