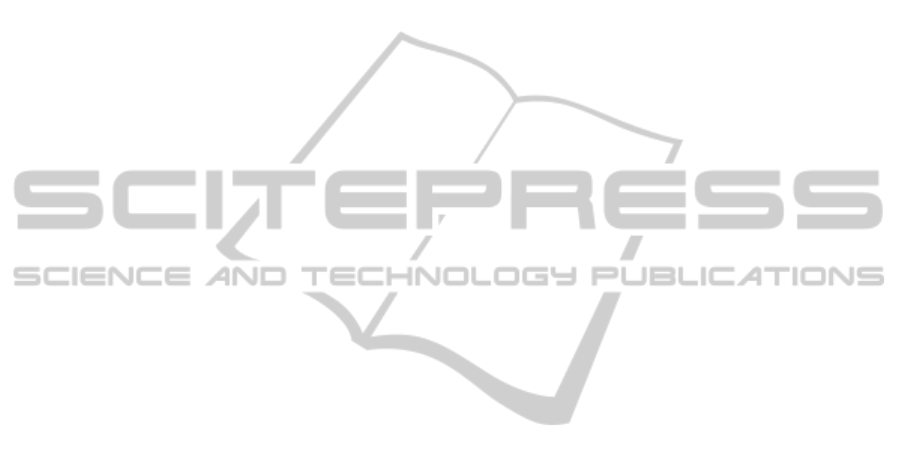
adaptive control and for nonadaptive control with sys-
tem parameters taken as their final estimates. The cost
function values for η = 0 are J
0
= 0.1848, 0.1828, and
J
0
= 0.2998, 0.2665 for mph and nmph nominal sys-
tems, respectively. Again, some jumps of cost func-
tion are observed in adaptive case for η > 0.
The norm condition of robust stability (20), for
both mph and nmph systems with known parameters
is also illustrated in Fig.6 for η = [0,2]. It can be ob-
served that robust stability margin for nmph system is
even larger than for mph system, i.e. the value of η
at which the norm attains 1 is greater in the case of
nmph system.
The RLS algorithm was applied for parameter
identification of a considered ARX model and as al-
ready has been shown in Figures 1, 3 the estimates
converge to the true nominal values for both mph and
nmph systems.
For general ARMAX models, the recursive pseu-
dolinear regression (RPLR) or recursiveprediction er-
ror (RPEM) algorithms should be applied. The results
shown in (Nilsson and Egardt, 2010), confirm that
RPEM is then more suitable in the considered under-
modelled situation taking into account the asymptotic
properties of the algorithms.
6 CONCLUSIONS
Simple adaptive discrete-time LQG control in the
context of LTR is presented. Parameter estimation of
ARMAX model is used for tuning the discrete-time
compensator. The interplay between robustness, per-
formance and estimation convergence with respect to
the modeling error is underlined. Examples of third-
order actual systems described by a second-order mph
and nmph nominal models are taken for simulation.
Simulation results show an effectivness of the adap-
tive LQG control with possible LTR effect as a way
for robustifying the adaptive control especially for
mph systems. On the other hand, problems with dis-
continuous solution of Riccati equation may occur for
nmph systems.
REFERENCES
A. Saberi, B. C. and Sannuti, P. Theory of ltr for non-
minimum systems, recoverable target loops, and re-
covery in a subspace. Int. J. Control.
Bitmead, R., Gevers, M., and Wertz, V. (1990). Adaptive
Optimal Control. Prentice Hall International.
Duncan, T. and B.Pasik-Duncan (1999). Adaptive
continuous-time linear quadratic gaussian control.
IEEE Trans. Automat. Contr., 44(9):1653–1665.
Krolikowski, A. (1995). Amplitude constrained
adaptive LQG control of first order systems.
Int.J.Adapt.Contr.Sign.Proc., 9(3):285–299.
Kumar, P. (1983). Optimal adaptive control of linear-
quadratic-gaussian systems. SIAM J. Control and Op-
timization, 21(2):163–178.
Maciejowski, J. (1985). Asymptotic recovery for discrete-
time systems. IEEE Trans. Automat. Contr.,
30(6):602–605.
M¨akil¨a, P., Westerlund, T., and Toivonen, H. (1984). Con-
strained linear quadratic gaussian control with process
applications. Automatica, 20(1):15–29.
Nilsson, M. and Egardt, B. (2010). Comparing recursive
estimators in the presence of unmodeled dynamics. In
Proc. IFAC Symp. ALCOSP, pages 135–149, Antalya,
Turkey.
Saberi, A. and Stoorvogel, A. A. (1996). Continuity prop-
erties of solutions to h
2
and h
∞
riccati equations. Sys-
tems and Control Letters., 27(4):209–222.
Tadjine, M., M’Saad, M., and Dugard, L. (1994). Discrete-
time compensators with loop transfer recovery. IEEE
Trans. Automat. Contr., 39(6):1259–1262.
Tay, T. and Moore, J. (1989). Loop recovery via h
∞
/h
2
sensitivity recovery. Int.J.Control, 49(4):1249–1271.
Tay, T. and Moore, J. (1991). Adaptive LQG controller with
loop transfer recovery. Int.J.Adapt.Contr.Sign.Proc.,
5(2):135–149.
AdaptiveLQG/LTRControl;DiscontinuityIssue
807