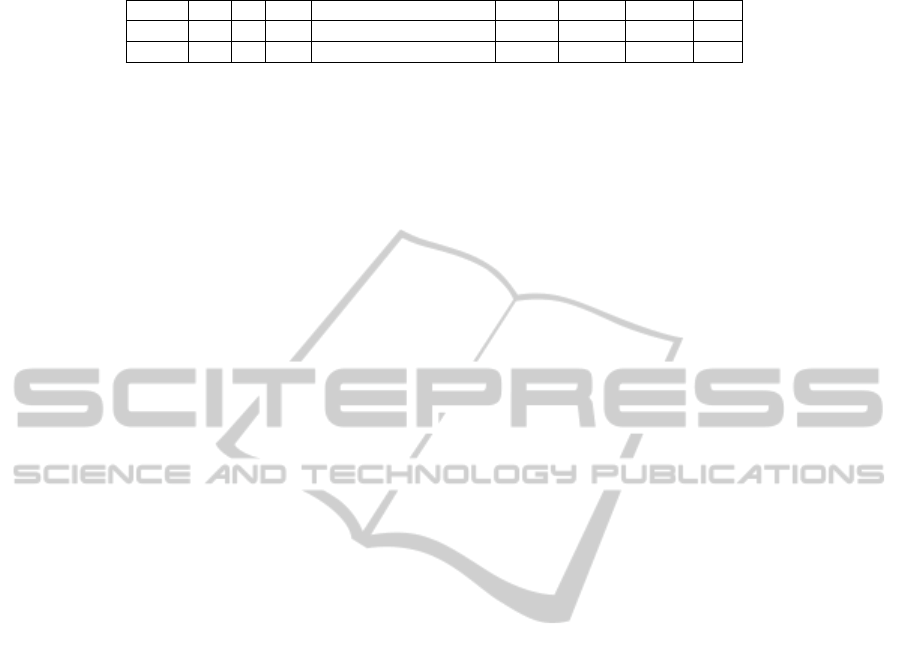
Table 2: Result of the best simulation obtained with the SD and SM.
Model α
f
k
i
k
opt
Categorical distance γ % Ts1 % Ts2 DB
A 0.3 7 7 Semantic Dissimilarity (SD) 0.4033 91.46% 23,12% 8.86
B 0.3 7 7 Simple Matching (SM) 0.4181 98,78% 35.76% 15.4
Maintenance procedure to be employed in the elec-
tric energy distribution network of Rome, Italy, man-
aged by ACEA Distribuzione S.p.A. By relying on
the OCC approach, the faults decision region is syn-
thetized by partitioning the available samples of the
training set. A suited pattern dissimilarity measure
has been defined in order to deal with different fea-
tures data types. The adopted clustering procedure
is a modifed version of k-means, with a novel proce-
dure for centroids initialization. A genetic algorithm
is in charge to find the optimal value of the dissimilar-
ity measure weights, as well as two parameters con-
trolling the initial centroids positioning and the fault
decision region extent, respectively. According to our
tests, the new proposed method for k-means initializa-
tion shows a good reliability in finding automatically
the best number of clusters and the best positions of
the centroids. Furthermore, the proposed SD for cat-
egorical features subspaces performs better than the
plain SM distance when used to define a pattern to
cluster dissimilarity measure. Since faults decision
region is synthetized starting from each cluster de-
cision region, this measure has a key role in defin-
ing a proper inductive inference engine, and thus in
improving the generalization capability of the recog-
nition system. Future works will be focused on the
definition of a suitable reliability classification mea-
sure, computed as the membership of incoming mea-
sures (patterns) to the fault decision region. Lastly,
tests results performed on real data make us confi-
dent about further systems developments possibility,
towards a final commissioning into the Rome electric
energy distribution network.
ACKNOWLEDGEMENTS
The authors wish to thank Acea Distribuzione S.p.A.
for providing the faults data and for their useful sup-
port during the OCC system design and test phases.
Special thanks to Ing. Stefano Liotta, Chief Network
Operation Division, and to Ing. Silvio Alessandroni,
Chief Electric Power Distribution Remote Control Di-
vision.
REFERENCES
Afzal, M. and Pothamsetty, V. (2012). Analytics for dis-
tributed smart grid sensing. In Innovative Smart Grid
Technologies (ISGT), 2012 IEEE PES, pages 1–7.
Barakbah, A. and Kiyoki, Y. (2009). A pillar algorithm for
k-means optimization by distance maximization for
initial centroid designation. pages 61–68.
Cai, Y. and Chow, M.-Y. (2009). Exploratory analysis of
massive data for distribution fault diagnosis in smart
grids. In Power Energy Society General Meeting,
2009. PES ’09. IEEE, pages 1–6.
Cheng, V., Li, C.-H., Kwok, J. T., and Li, C.-K. (2004). Dis-
similarity learning for nominal data. Pattern Recogni-
tion, 37(7):1471 – 1477.
Dan Pelleg, A. M. (2000). X-means: Extending k-means
with efficient estimation of the number of clusters. In
Proceedings of the Seventeenth International Confer-
ence on Machine Learning, pages 727–734, San Fran-
cisco. Morgan Kaufmann.
Davies, D. L. and Bouldin, D. W. (1979). A cluster separa-
tion measure. IEEE Transactions on Pattern Analysis
and Machine Intelligence, PAMI-1(2):224–227.
De Santis, E., Rizzi, A., Livi, L., Sadeghian, A., and Frat-
tale Mascioli, F. M. (2014). Fault recognition in smart
grids by a one-class classification approach. In 2014
IEEE World Congress on Computational Intelligence.
IEEE.
De Santis, E., Rizzi, A., Sadeghian, A., and Frattale Mas-
cioli, F. M. (2013). Genetic optimization of a fuzzy
control system for energy flow management in micro-
grids. In 2013 Joint IFSA World Congress and
NAFIPS Annual Meeting, pages 418–423. IEEE.
Del Vescovo, G., Livi, L., Frattale Mascioli, F. M., and
Rizzi, A. (2014). On the Problem of Modeling Struc-
tured Data with the MinSOD Representative. Interna-
tional Journal of Computer Theory and Engineering,
6(1):9–14.
Energy Information Admin. (2013). International Energy
Outlook 2011 - Energy Information Administration,
note=http://www.eia.gov/forecasts/ieo/index.cfm.
European Technology Plat. (2013). The Smart-
Grids European Technology Platform,
note=http://www.smartgrids.eu/ETPSmartGrids.
Guikema, S. D., Davidson, R. A., and Haibin, L. (2006).
Statistical models of the effects of tree trimming on
power system outages. IEEE Transactions on Power
Delivery, 21(3):1549–1557.
He, Z., Xu, X., and Deng, S. (2011). Attribute value weight-
ing in k-modes clustering. Expert Systems with Appli-
cations, 38(12):15365 – 15369.
Huang, Z. (1998). Extensions to the k-means algorithm for
clustering large data sets with categorical values.
ECTA2014-InternationalConferenceonEvolutionaryComputationTheoryandApplications
102