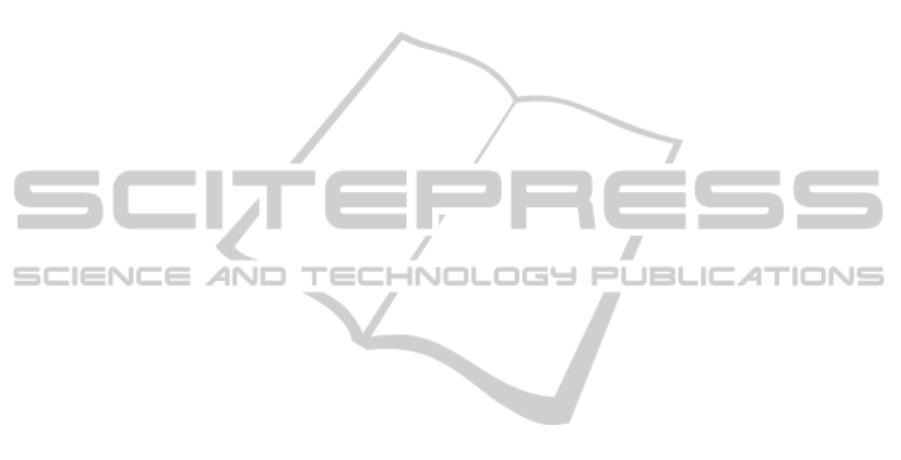
However, considering hidden node 4, each class (brand, object, and person) is
discriminated. It is possible to conclude that this node is able to successfully
segregate among classes, which is also supported by the data in Table 1 and Table 2.
In fact, the cells with correct hits (grey background) concentrate the majority of the
assessments and have the lowest probability values.
Nonetheless, discriminating between preferred and indifferent brands it is not so
good. The values of the four cells that involve preferred and indifferent brands in
Table 1 are approximate, and in the case of indifferent brands, the network has more
tendency to classify as preferred brands. The analysis of the same four cells in Table
2 confirms this observation. The reason for such has to be explored. The problem
may be intrinsic to the stimulus, because of its low salience, or the method has to be
improved in order to attain such refinement.
IC2 and IC7 (depicted in Fig. 2) are two important sources of data for successful
classification. Interestingly these two brain networks encompass brain regions from
visual and visual associative areas. This is in line with the findings of Hanson,
Matsuka and Haxby [4], which also found in fusiform gyri sources of cognitive data
for accurate classification.
References
1. Pereira, F., Mitchell, T.M., Botvinick, M.: Machine learning classifiers and fMRI: a tutorial
overview. Neuroimage 45, S199-S209 (2009)
2. Mitchell, T. M., Hutchinson, R., Niculescu, R. S., Pereira, F., Wang, X., Just, M., Newman,
S.: Learning to Decode Cognitive States from brain images. Machine Learning 57, 145-175
(2004)
3. Haynes, J.-D., Rees, G.: Decoding mental states from brain activity in humans. Nature
Reviews Neuroscience 7, 523-534 (2006)
4. Hanson, S. J., Matsuka, T., Haxby, J. V.: Combinatorial Codes in Ventral Temporal lobe
for Object Recognition: Haxby (2001) revisited: is there a "face" area? Neuroimage 23,
156-166 2004
5. Misaki, M., Miyauchi, S.: Application of artificial neural network to fMRI regression
analysis. Neuroimage 29, 396-408 (2006)
6. Sona, D., Veeramachaneni, S., Olivetti, E., Avesani, P.: Inferring Cognition from fMRI
Brain Images. In: Marques de Sá, J., Alexandre, L., Duch, W., Mandic, D. (eds.) Artificial
Neural Networks – ICANN 2007, vol.4669, pp.869-878. Springer Berlin/ Heidelberg, 2007
7. Fox, M.D., Snyder, A.Z., Vincent, J.L., Corbetta, M., Van Essen, D.C., Raichle, M.E.: The
human brain is intrinsically organized into dynamic, anticorrelated functional networks.
Proceedings of the National Academy of Sciences of the United States of America 102,
9673-9678 (2005)
8. Pereira, F., Botvinick, M.: Information mapping with pattern classifiers: a comparative
study. Neuroimage 56, 476-496 (2011)
9. Misaki, M., Kim, Y., Bandettini, P.A., Kriegeskorte, N.: Comparison of multivariate
classifiers and Response Normalizations for Pattern-information fMRI. Neuroimage 53,
103-118 (2010)
10. Hacker, C.D., Laumann, T.O., Szrama, N.P., Baldassarre, A., Snyder, A.Z., Leuthardt,
E.C., Corbetta, M.: Resting state network estimation in individual subjects. NeuroImage
82, 616-633 (2013)
11. Raichle, M. E., MacLeod, A. M., Snyder, A. Z., Powers, W. J., Gusnard, D.A., Shulman,
51