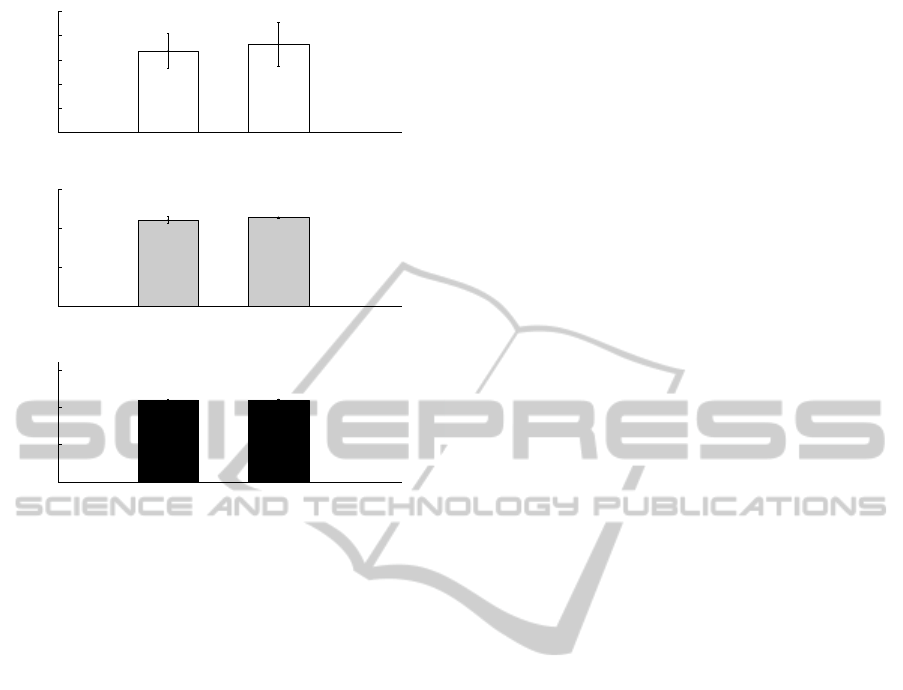
Not Ord. Config. Ord. Config.
0
20
40
60
80
100
Generation
(a)
Not Ord. Config. Ord. Config.
0
0.01
0.02
0.03
Fitness reduction (%)
(b)
Not Ord. Config. Ord. Config.
0
500
1000
1500
AP Loss Reduction [W]
(c)
Figure 4: Mean value of the number of generations (a), the
mean percentage reduction fitness value (b) and the mean
reduction of active power loss (c) with the respective stan-
dard deviation for ordered and unordered list of configura-
tions.
becomes considerable.
6 CONCLUSIONS
In this paper an improvement of the control system
described in (Storti et al., 2013a), (Possemato et al.,
2013), (Storti et al., 2013b) and (Storti et al., 2014) is
presented. We propose an heuristic method to com-
pare admissible network topologies and a criteria to
order the list of such topologies aiming to improve the
continuity of the objective function to the variation of
the configuration parameter. We execute some tests
on the SG sited in the west area of Rome realized by
ACEA Distribuzione S.p.A.. Results show that, for the
network under analysis, the proposed ordering pro-
cedure makes the joint PFC and DFR optimization
problem simpler to cope with a plain genetic algo-
rithm. In future works we intend to verify the criteria
in a more complex network and at different time inter-
vals. Moreover, by exploiting the property described
by Equations 13 and 14, it is possible to redefine more
suitable mutation and crossover operators to furtherly
improve the convergence of the GA during the evolu-
tionary process.
REFERENCES
Carreno, E., Romero, R., and Padilha-Feltrin, A. (2008). An
efficient codification to solve distribution network re-
configuration for loss reduction problem. IEEE Trans-
action on Power Systems, 23(4):15421551.
Chandramohan, S., Atturulu, N., Kumudini, R., and Ven-
takesh, B. (2010). Operating cost minimization of
a radial distribution system in a deregulated electric-
ity market through reconfiguration using nsga method.
International Journal of Electric Power Energy Sys-
tems, 32(2):126132.
Civanlar, S., Grainger, J., Yin, H., and Lee, S. (1988).
Distribution feeder reconfiguration for loss reduc-
tion. IEEE Transactions on Power Delivery,
3(3):12171223.
Dahu, L. (2011). Electric power communication system of
the future smart grid. Telecommunication for Electric
Power System, 32(222).
Merlin, A. and Back, H. (1975). Search for minimum-
loss operational spanning tree configuration for urban
power distribution systems. In Proceeding of Fifth
power system conference (PSCC), page 118.
Nara, K., Shiose, A., Kitagawa, M., and Ishibara, T. (1992).
Implementation of genetic algorithm for distribution
systems loss minimum reconfiguration. IEEE Trans-
action on Power Systems, 7(3):10441051.
Possemato, F., Storti, G., Paschero, M., Rizzi, A., and Frat-
tale Mascioli, F. (2013). Two evolutionary computa-
tion approaches for active power losses minimization
in smart grids. In Proceedings of North America Fuzzy
Information Processing Society.
Singh, M., Khadkikar, V., Chandra, A., and Varma, R.
(2011). Grid interconnection of renewable energy
sources at the distribution level with power-quality
improvement features. IEEE Transactions on Power
Delivery, 26(1):307–315.
Storti, G., Possemato, F., Paschero, M., Alessandroni, S.,
Rizzi, A., and Mascioli, F. F. (2013a). Neural Nets
and Surroundings 22nd Italian Workshop on Neu-
ral Nets, WIRN 2012, May 17-19, Vietri sul Mare,
Salerno, Italy, volume 19 of Smart Innovation, Sys-
tems and Technologies, chapter Active power losses
constrained optimization in Smart Grids by gentic al-
gorithms, pages 279–288. Springer Berlin Heidelberg.
Storti, G., Possemato, F., Paschero, M., and nad F.M. Frat-
tale Mascioli, A. R. (2014). A radial configurations
search algorithm for joint pfc and dfr optimization in
smart grids. In Proceeding of 23th international con-
ference on industrial electrincs (ISIE).
Storti, G., Possemato, F., Paschero, M., Rizzi, A., and Frat-
tale Mascioli, F. M. (2013b). Optimal distribution
feeders configuration for active power losses mini-
mization by genetic algorithms. In Proceedings of
North America Fuzzy Information Processing Society.
ECTA2014-InternationalConferenceonEvolutionaryComputationTheoryandApplications
280