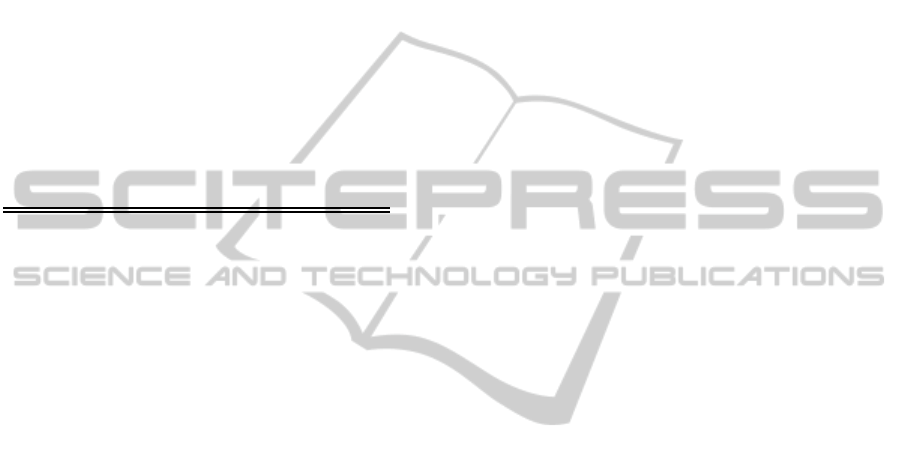
sequences of S
ts
before and after the reduction phase
described in Section 3.3. We can note that the over-
all number of selected sequences after the reduction
phase is higher for single stocks than for the stock
market indexes. Considering that the error rate be-
tween the stock market indexes and the single stocks
(apart from CAC-40) is very similar, we can say that
this is a very encouraging result because in principle,
if we perform this method on a big set of different
stocks, we could obtain a gain on average. From this
point of view, we consider very promising the per-
formances obtained on real stocks prices in this first
work.
Table 1: Results on Real Data Sets: Lukoil (L), Gazprom
(G), MICEX (M), CAC-40 (C). Classification Error on Test
Set, number of sequences in the Test Set and number of
selected sequences after the reduction procedure.
Err. S
ts
(%) # seq. S
ts
# sel. seq. S
ts
L 52.8571 202 63
G 54.2334 202 126
M 55.5556 202 13
C 100 208 1
5 CONCLUSION AND FUTURE
WORK
In this paper we introduce a new classification system
aiming to perform trend prediction on financial time
series. We prove, through synthetic benchmarking
data sets, that if there are some regularities inside data
our method is able to detect them, showing good clas-
sification performances also in the presence of noise
and with errors in the labeling procedure. The results
on real data show us the path to follow for the future
extension of the proposed method. We consider as en-
couraging the result obtained on real data, suggesting
further developments. To this aim, among other pos-
sible improvements, we are currently working on an
agent based mining algorithm, as the core procedure
for the alphabet synthesis.
REFERENCES
Bagheri, A., Mohammadi Peyhani, H., and Akbari, M.
(2014). Financial forecasting using anfis networks
with quantum-behaved particle swarm optimization.
41:6235–6250.
Bargiela, A. and Pedrycz, W. (2003). Granular computing:
an introduction. Springer.
Berndt, D. J. and Clifford, J. (1994). Using dynamic time
warping to find patterns in time series. In KDD work-
shop, volume 10, pages 359–370. Seattle, WA.
Chang, P.-C., Fan, C.-Y., and Liu, C.-H. (2009). Integrating
a piecewise linear representation method and a neu-
ral network model for stock trading points prediction.
Systems, Man, and Cybernetics, Part C: Applications
and Reviews, IEEE Transactions on, 39(1):80–92.
Cheng, C.-H., Su, C.-H., Chen, T.-L., and Chiang, H.-H.
(2010). Forecasting stock market based on price trend
and variation pattern. 5990:455–464.
Haugen, R. A. (1999). The new finance: the case against ef-
ficient markets, volume 2. Prentice Hall Upper Saddle
River.
Kendall, G. and Su, Y. (2005). A particle swarm optimi-
sation approach in the construction of optimal risky
portfolios. In Artificial Intelligence and Applications,
pages 140–145. Citeseer.
Livi, L., Del Vescovo, G., and Rizzi, A. (2012). Graph
recognition by seriation and frequent substructures
mining. In ICPRAM, pages 186–191.
Los, C. A. (2000). Nonparametric efficiency testing of asian
stock markets using weekly data. Advances in Econo-
metrics, 14:329–363.
Malkiel, B. G. and Fama, E. F. (1970). Efficient capital
markets: A review of theory and empirical work. The
journal of Finance, 25(2):383–417.
Muggeo, V. M. (2003). Estimating regression models
with unknown break-points. Statistics in medicine,
22(19):3055–3071.
Nanni, L. (2006). Multi-resolution subspace for financial
trading. Pattern recognition letters, 27(2):109–115.
Possemato, F. and Rizzi, A. (2013). Automatic text cate-
gorization by a granular computing approach: Facing
unbalanced data sets. In Neural Networks (IJCNN),
The 2013 International Joint Conference on, pages 1–
8. IEEE.
Radeerom, M., Wongsuwarn, H., and Kasemsan, M. L. K.
(2012). Intelligence decision trading systems for stock
index. 7198:366–375.
Rizzi, A., Del Vescovo, G., Livi, L., and Mascioli, F. M. F.
(2012). A new granular computing approach for se-
quences representation and classification. In Neural
Networks (IJCNN), The 2012 International Joint Con-
ference on, pages 1–8. IEEE.
Rizzi, A., Possemato, F., Livi, L., Sebastiani, A., Giuliani,
A., and Mascioli, F. M. F. (2013). A dissimilarity-
based classifier for generalized sequences by a gran-
ular computing approach. In Neural Networks
(IJCNN), The 2013 International Joint Conference on,
pages 1–8. IEEE.
Sai, Y., Yuan, Z., and Gao, K. (2007). Mining stock mar-
ket tendency by rs-based support vector machines. In
Granular Computing, 2007. GRC 2007. IEEE Inter-
national Conference on, pages 659–659. IEEE.
Vanstone, B. and Tan, C. (2003). A survey of the application
of soft computing to investment and financial trading.
Information Technology papers, page 13.
ECTA2014-InternationalConferenceonEvolutionaryComputationTheoryandApplications
288