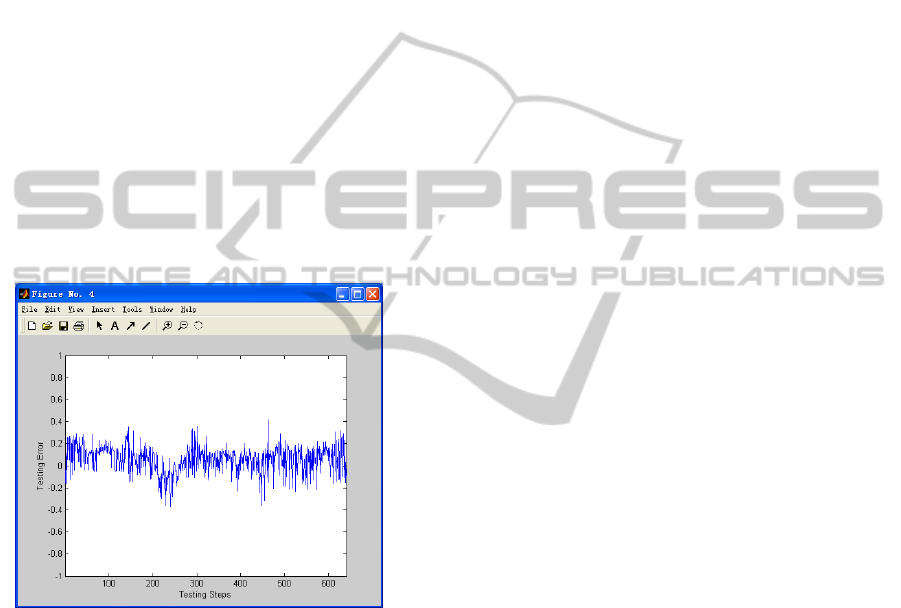
Where, η is the learning step, d
i
is the desired
output for node i, x
i
is the real output for node i, x
j
is
the input for node i, X is a Polynomial, which is:
1
(9)
5 EXPERIMENTS
In order to verify the validity of Choquet integral-
OWA operator based Adaptive Universal Fuzzy
Inference System (Agg-AUFIS) presented in this
paper, we established Agg-AUFIS for evaluation of
traffic level of service, which are trained and tested
by historical sample data (1429 pairs for training and
640 pairs for testing).
Testing errors are shown in Figure 5. Average
test error is 0.057391. The worst test error is 0.4154
while the best test error is 1.6785e-005. The results
indicated that Agg-AUFIS could be well adapted to
sample data and it is a kind of universal
approximator.
Figure 5: Testing error.
6 CONCLUSIONS
Based on FIS capability of simulating human
reasoning process and dealing with nonlinear system
problems, this paper presents a Choquet integral-
OWA operator based fuzzy inference system
(AggFIS) that is universal in reasoning operator
selection, inference model structure and importance
factor expression, and its adaptive model known as
Agg-AUFIS. The experiments results showed that
Agg-AUFIS has great non-linear mapping function
and complex system modeling capacity. The
comparative experiments will be made between
Agg-AUFIS and existing similar systems, which
could verify the advantages and effectiveness of
proposed model in future work.
REFERENCES
Esragh, F., Mamdani, E. H., 1981. A general approach to
linguistic approximation. Fuzzy Reasoning and Its
Applications, Academic Press. London.
Furuhashi, T., 2001. Fusion of fuzzy/neuro/evolutionary
computing for knowledge acquisition. Proceedings of
IEEE, 89(9), 1266-1274.
Grabisch, M., 2000. A Graphical Interpretation of the
Choquet Integral. IEEE transactions on Fuzzy
Systems, 8(5), 627-631.
Kelman, A., Yager, R. R., 1995. On the Application of a
Class of MICA Operators. International Journal of
Uncertainty, Fuzziness and Knowledge-Based
Systems, 3,113-125.
Mamdani, E. H., 1977. Application of Fuzzy Logic to
Approximate Reasoning Using Linguistic Synthesis.
IEEE transactions on computer, 26(12), 1182-1191.
Pedrycz, W., Lam, P., Rocha, A. F., 1995. Distributed
fuzzy system modeling. IEEE transactions on fuzzy
systems, man, and cybernetics, 25(5), 769-780.
Pedrycz, W., Oliveira, J., 1996. Optimization of fuzzy
models. IEEE transactions on fuzzy systems, man, and
cybernetics -PART B: cybernetics, 26(4), 627-636.
Rumelhart, D. E., Hinton, G. E., Williams, R. J., 1986.
Learning internal representations by error
propagation. Parallel distributed processing:
explorations in the microstructure of cognition, MIT
Press. Cambridge.
Rumelhart, D. E., 1994. The Basic Ideas in Neural
Networks. Communications of the ACM, 37(3), 87-92.
Yager, R. R., 1988. On Ordered Weighted Averaging
Aggregation Operators in Multicriteria
Decisionmaking. IEEE transactions on fuzzy systems,
man, and cybernetics, 18(1),183-190.
Yager, R. R., Filev, D. P., 1992. SLIDE:A simple adaptive
defuzzification method. IEEE transactions on Fuzzy
Systems, 1(1), 69-78.
Yager, R. R., 1993a. On a Hierarchical Structure for Fuzzy
Modeling and Control. IEEE transactions on fuzzy
systems, man, and cybernetics, 23(4), 1189-1197.
Yager, R. R., 1993b. Families of OWA operators. Fuzzy
Sets and Systems, 59, 125-148.
Yager, R. R., 1996. On Mean Type Aggregation. IEEE
transactions on fuzzy systems, man, and cybernetics -
PART B: cybernetics, 26(2), 209-221.
Zadeh, L. A., 1965. Fuzzy Set. Information and Control, 8,
338-353.
Zadeh, L. A., 1973. Outline of a New Approach to the
Analysis of Complex Systems and Decision Processes.
IEEE Transactions on Systems, Man, and Cybernetics,
3(1), 28-44.
Zadeh, L. A., 1988. Fuzzy Logic. Computer, 21(4), 83-93.
Zadeh, L. A., 1994. Soft Computing and Fuzzy Logic.
IEEE Software, 11(6), 48-56.
FCTA2014-InternationalConferenceonFuzzyComputationTheoryandApplications
168