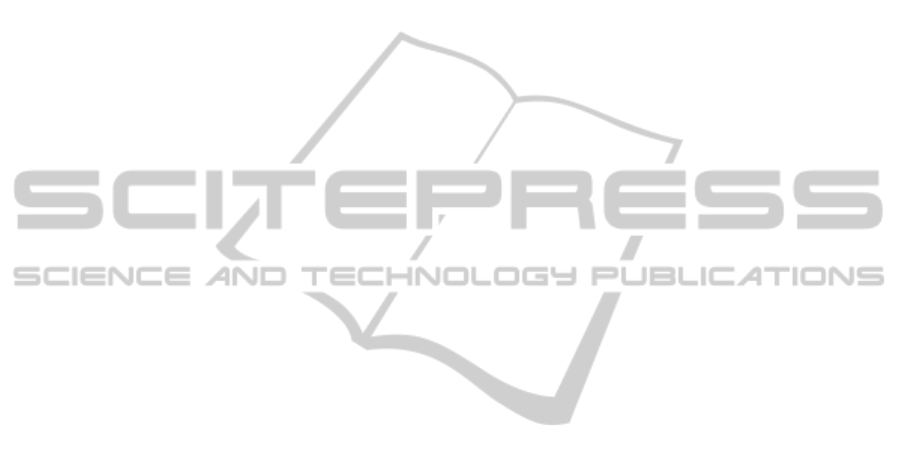
5 CONCLUSIONS
Forecasting non-linear financial time series has
received increased attention in recent years. This
paper proposed a novel EMD-filter in combination
with a neural network in order to forecast exchange
rates with the purpose of profitable trading. The
proposed model is compared to an unfiltered neural
network and a random walk model for out-of-sample
prediction of the EUR/USD and USD/JPY rates of
2013.
The proposed EMD-filtered neural network was
the best performing model based on the criteria of
directional symmetry, correlation and simulated
returns. This can be attributed to the EMD-filter’s
ability to increase the signal-to-noise ratio for the
applicable forecast horizon. The results are in
accordance with previous studies on EMD-based
prediction models where the use of EMD has
improved the prediction accuracy.
The two sample t-test rejects the similarity
between the returns generated by the proposed
EMD-filtered ANN model and the random walk
model at a significance level of 99% in all cases
except for 20 hour prediction horizons, where the
significance level if 95%. This is an indication that
the proposed model can consistently deliver higher
returns than a random walk at all the forecast
horizons for both exchange rates.
In conclusion, the out-of-sample test results
reveal that EMD-filtered ANN forecasting can be an
effective tool for investors in predicting exchange
rates.
REFERENCES
Cheng, C.-H. & Wei, L.-Y., 2014. A novel time-series
model based on empirical mode decomposition for
forecasting TAIEX. Economic Modelling, 36, pp.136-
141.
Flandrin, P., 2004. Empirical mode decomposition as a
filter bank. IEEE signal processing letters, 11(2),
pp.112-114.
Fu, C., 2010. Forecasting exchange rate with EMD-based
support vector regression. IEEE submissions, pp.0-3.
Hsieh, D., 1989. Testing for nonlinear dependence in daily
foreign exchange rates. Journal of Business, 62(3),
pp.339-368.
Huang, N.E. et al., 1996. The empirical mode
decomposition and the Hilbert spectrum for nonlinear
and non-stationary time series analysis. In
Proceedings of the Royal Society London. pp. 904-
993.
Huang, N.E. et al., 2003. Applications of Hilbert-Huang
transform to non-stationary financial time series
analysis. Applied Stochastic Models in Business and
Industry, 19(4), pp.361-361.
Imam, T., 2012. Intelligent Computing and Foreign
Exchange Rate Prediction : What We Know and We
Don ’ t. Progress in Intelligent Computing and
Applications, 1(1), pp.1-15.
Laurene Fausett, 1994. Fundamentals of Neural Networks
1st ed., Upper Saddle River: Prentice Hall.
Lin, C., Chiu, S.-hsiung & Lin, T., 2012. Empirical mode
decomposition–based least squares support vector
regression for foreign exchange rate forecasting.
Economic Modelling, 29(6), pp.2583-2590.
Lu, C.-J., Lee, T.-S. & Chiu, C.-C., 2009. Financial time
series forecasting using independent component
analysis and support vector regression. Decision
Support Systems, 47(2), pp.115-125.
Nusair, S.A., 2013. Real exchange rate dynamics in
transition economies: a nonlinear analysis.
International Journal of Finance and Economics,
18(March 2012), pp.188-204.
Pavlidis, E.G., Paya, I. & Peel, D.A., 2012. Forecast
Evaluation of Nonlinear Models : The Case of Long-
Span Real Exchange Rates. Journal of Forecasting,
595(September 2011), pp.580-595.
Tay, F. & Cao, L., 2001. Application of support vector
machines in financial time series forecasting. Omega,
29, pp.309-317.
Wang, W. et al., 2009. A Novel Hybrid Intelligent Model
for Financial Time Series Forecasting and Its
Application. 2009 International Conference on
Business Intelligence and Financial Engineering,
pp.279-282.
Xiong, T., Bao, Y. & Hu, Z., 2013. Beyond one-step-
ahead forecasting: Evaluation of alternative multi-
step-ahead forecasting models for crude oil prices.
Energy Economics, 40, pp.405-415.
Yang, H. & Lin, H., 2012. Combining Artificial
Intelligence with Non-linear Data Processing
Techniques for Forecasting Exchange Rate Time
Series. International Journal of Digital Content
Technology and its Applications
, 6(April), pp.276-284.
Yu, L., Wang, S. & Lai, K.K., 2008. Forecasting crude oil
price with an EMD-based neural network ensemble
learning paradigm. Energy Economics, 30(5),
pp.2623-2635.
Yu, L. et al., 2010. A multiscale neural network learning
paradigm for financial crisis forecasting.
Neurocomputing, 73(4-6), pp.716-725.
CombiningEmpiricalModeDecompositionwithNeuralNetworksforthePredictionofExchangeRates
249