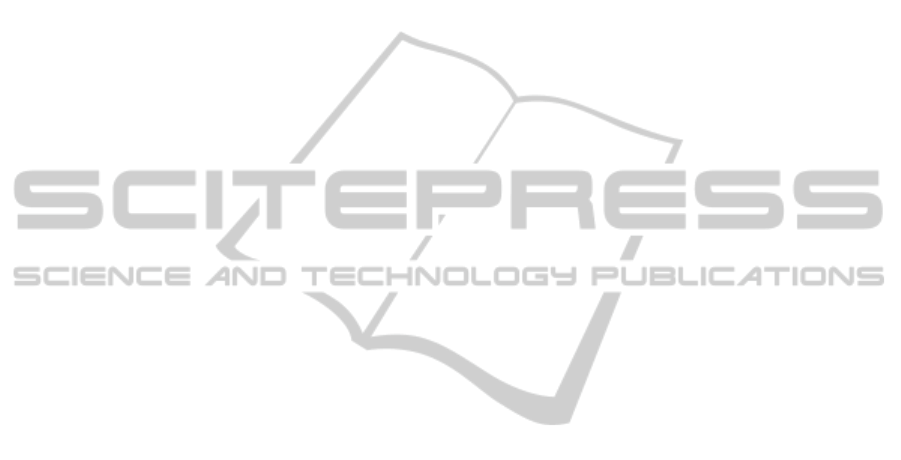
Hoard, M., Homer, J., Manley, W., Furbee, P., Haque, A.
& Helmkamp, J. 2005, "Systems modeling in support
of evidence-based disaster planning for rural areas",
International journal of hygiene and environmental
health, vol. 208, no. 1–2, pp. 117-125.
Homer, J., Hirsch, G., Minniti, M. & Pierson, M. 2004,
"Models for collaboration: how system dynamics
helped a community organize cost-effective care for
chronic illness", System Dynamics Review (Wiley),
vol. 20, no. 3, pp. 199-222.
Jacobson, S.H., Sewell, E.C. & Weniger, B.G. 2001,
"Using Monte Carlo simulation to assess the value of
combination vaccines for pediatric immunization" in
Proceedings of the 33nd Winter Simulation
Conference IEEE Computer Society, Washington, DC,
USA, pp. 1421-1428.
Kasaie, P., Kelton, W.D., Vaghefi, A. & Naini, S.G.R.J.
2010, "Toward optimal resource-allocation for control
of epidemics: an agent-based-simulation approach",
Proceedings of the 2010 Winter Simulation
Conference, pp. 2237-2248.
Krejci, I., Kvasnicka, R. & Svasta, J. 2011, "Dynamic
aging chain of the Czech Republic population", Acta
Universitatis et Silviculturae Mendelianae Brunensis,
vol. LIX (7), pp. 209-216.
Lagergren, M. 1998, "What is the role and contribution of
models to management and research in the health
services? A view from Europe", European Journal of
Operational Research, vol. 105, no. 2, pp. 257-266.
Lane, D.C., Monefeldt, C. & Rosenhead, J.V. 2000,
"Looking in the wrong place for healthcare
improvements: A system dynamics study of an
accident and emergency department", The Journal of
the Operational Research Society, vol. 51, no. 5, pp.
518-531.
Luke, D.A. & Stamatakis, K.A. 2012, "Systems science
methods in public health: dynamics, networks, and
agents", Annual Review of Public Health, vol. 33, no.
1, pp. 357-376.
Martin, S., Rice, N., Jacobs, R. & Smith, P. 2007, "The
market for elective surgery: Joint estimation of supply
and demand", Journal of health economics, vol. 26,
no. 2, pp. 263-285.
Mielczarek, B. & Uziałko-Mydlikowska, J. 2012,
"Application of computer simulation modeling in the
health care sector: a survey", Simulation, vol. 88, no.
2, pp. 197-216.
Mielczarek, B. 2013, "Using discrete-event simulation to
forecast the volume of hospital emergency services to
be delivered at the regional level", SIMULTECH 2013
- Proceedings of the 3rd International Conference on
Simulation and Modeling Methodologies,
Technologies and Applications, pp. 197-203.
Mielczarek, B. 2014, "Simulation modelling for
contracting hospital emergency services at the regional
level", European Journal of Operational Research,
vol. 235, no. 1, pp. 287-299.
Mustafee, N., Katsaliaki, K. & Taylor, S.J.E. 2010,
"Profiling literature in healthcare simulation",
Simulation, vol. 86, no. 8-9, pp. 543-558.
Sterman, J.D. 2000, Business dynamics. System thinking
and modeling for a complex world, McGraw-Hill
Higher Education, USA.
Taylor, K. & Dangerfield, B. 2005, "Modelling the
feedback effects of reconfiguring health services", The
Journal of the Operational Research Society, vol. 56,
no. 6, pp. 659-675.
Vanderby, S. & Carter, M.W. 2010, "An evaluation of the
applicability of system dynamics to patient flow
modelling", The Journal of the Operational Research
Society, vol. 61, no. 11, pp. 1572-1581.
Whitworth, M.H. 2006, "Designing the response to an
anthrax attack", Interfaces, vol. 36, no. 6, pp. 562-568.
Wong, H.J., Morra, D., Wu, R.C., Caesar, M. & Abrams,
H. 2012, "Using system dynamics principles for
conceptual modelling of publicly funded hospitals",
The Journal of the Operational Research Society, vol.
63, no. 1, pp. 79-88.
SIMULTECH2014-4thInternationalConferenceonSimulationandModelingMethodologies,Technologiesand
Applications
888