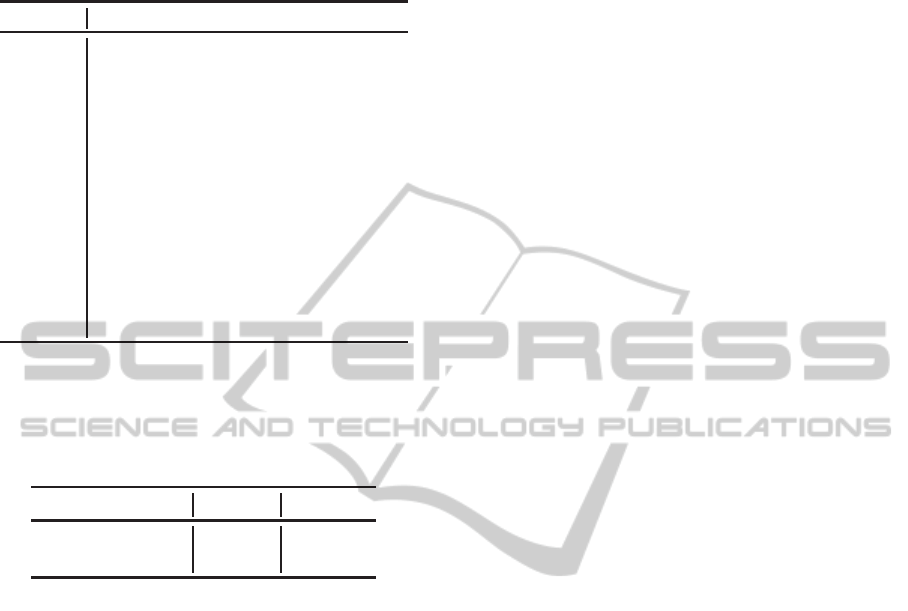
Table 2: Anatomical NEs that are missing in the original
text but are discovered in the transformed text by dictionary
look-up.
FMA ID Anatomical Term Name
50087 Anterior choroidal artery
50655
Calcarine artery
52573
Inferior branch of oculomotor nerve
59669
Roof of internal nose
61944
Anterior forceps of corpus callosum
62418
Lateral orbital gyrus
67956
Medial longitudinal stria
83759
Anterior ascending limb of lateral sulcus
83760
Anterior horizontal limb of lateral sulcus
83761
Posterior ascending limb of lateral sulcus
84114
Apical part of cell
256305
Lateral surface of cerebral hemisphere
256312
Basal surface of cerebral hemisphere
256318
Medial surface of cerebral hemisphere
256335
Tentorial surface of cerebral hemisphere
Table 3: The number of relations (subject–predicate–object
triples that contain anatomical NEs in both subject and ob-
ject) extracted from 787 test sentences, and the number of
those that were anatomically correct, before (= original text)
and after (= transformed text) decomposing cohesion.
# triples # correct
Original text 70 45
Transformed text 366 310
can extract the relations shown in Figure 3(b) from
the transformed texts, but not from the original ones.
5 CONCLUSION
In this paper, we proposed to transform the prose style
of anatomical textbooks by annotating reference ex-
pressions and coordinating conjunctions. We then
validated the utility of the transformed text for the
identification of anatomical NEs and their relations,
and verified that the cohesiveness of the text is one
of the major obstacles preventing us from accessing
knowledge in textbooks by methods other than read-
ing.
Since the transformed text is human readable as
well, the proposed style has potential to serve as a
new-model language resource that is accessible by
both human and machine, promising to improve the
computational reusability of anatomy textbooks. We
also believe the proposed method to be applicable to
texts in domains other than anatomy, as long as they
mainly consist of factual explanation of structures, for
instance natural or artificial geographical and geolog-
ical features.
REFERENCES
Abe, S., Inui, K., Hara, K., Morita, H., Sao, C., Eguchi,
M., Sumida, A., Murakami, K., and Matsuyoshi, S.
(2011). Mining personal experiences and opinions
from web documents. Web Intelligence and Agent Sys-
tems, 9(2):109–121.
Aramaki, E., Maskawa, S., and Morita, M. (2011). Twitter
catches the flu: Detecting influenza epidemics using
twitter. In EMNLP, pages 1568–1576.
Augenstein, I., Pad´o, S., and Rudolph, S. (2012). Lodi-
fier: Generating linked data from unstructured text. In
Proceedings of the 9th Extended Semantic Web Con-
ference (ESWC), pages 210–224.
Etzioni, O., Banko, M., Soderland, S., and Weld, D. S.
(2008). Open information extraction from the web.
Commun. ACM, 51(12):68–74.
Gray, H. (1918). Anatomy of the Human Body. Philadel-
phia: Lea & Febiger, 20 edition.
Hara, K., Shimbo, M., Okuma, H., and Matsumoto, Y.
(2009). Coordinate structure analysis with global
structural constraints and alignment-based local fea-
tures. In ACL-IJCNLP, pages 967–975, Suntec, Sin-
gapore. Association for Computational Linguistics.
Kim, J.-D., Ohta, T., Tateisi, Y., and Tsujii, J. (2003). Ge-
nia corpus - a semantically annotated corpus for bio-
textmining. In ISMB (Supplement of Bioinformatics),
pages 180–182.
Marcus, M. P., Marcinkiewicz, M. A., and Santorini, B.
(1993). Building a large annotated corpus of en-
glish: The penn treebank. Computational Linguistics,
19(2):313–330.
Ng, V. (2010). Supervised noun phrase coreference re-
search: The first fifteen years. In Proceedings of the
48th Annual Meeting of the Association for Compu-
tational Linguistics, pages 1396–1411, Uppsala, Swe-
den. Association for Computational Linguistics.
Riedel, S., Yao, L., McCallum, A., and Marlin, B. M.
(2013). Relation extraction with matrix factorization
and universal schemas. In NAACL-HLT, pages 74–84,
Atlanta, Georgia. Association for Computational Lin-
guistics.
Rosse, C. and Mejino, J. L. V. (2008). The Foundational
Model of Anatomy Ontology Anatomy Ontologies for
Bioinformatics. In Burger, A., Davidson, D., and Bal-
dock, R., editors, Anatomy Ontologies for Bioinfor-
matics, volume 6 of Computational Biology, chap-
ter 4, pages 59–117. Springer London, London.
Sch¨afer, U., Spurk, C., and Steffen, J. (2012). A
fully coreference-annotated corpus of scholarly pa-
pers from the acl anthology. In COLING (Posters),
pages 1059–1070.
Witte, S. P. and Faigley, L. (1981). Coherence, cohesion,
and writing quality. College Composition and Com-
munication, 32:189–204.
AnnotatingCohesiveStatementsofAnatomicalKnowledgeTowardSemi-automatedInformationExtraction
347