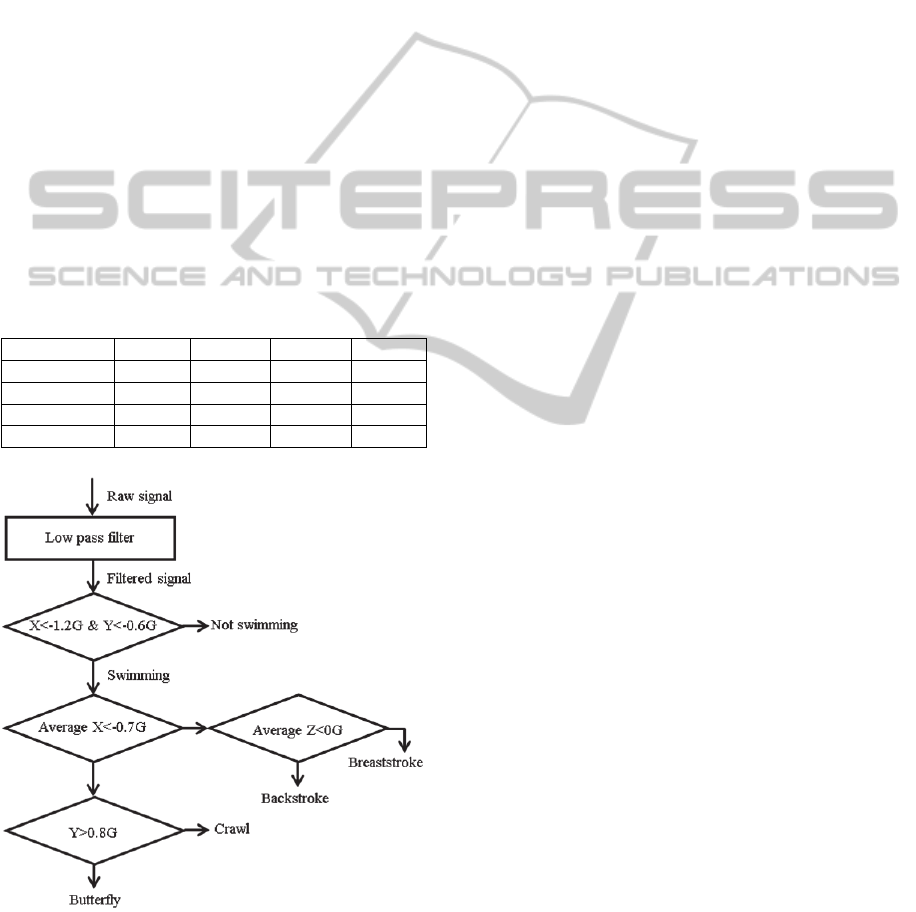
besides X axis threshold average on Y axis as
positive/negative was observed for style recognition.
To validate the algorithm, simulation of online
monitoring was performed. New data were released
to run through the algorithm continuously. The first
window was 20s long which was sliding over the
data.
3 RESULTS
For the 7 validation subjects, we had in total 42 laps
of swimming, each was 50m long. Our algorithm
showed highest performance by identifying all 42
swimming intervals and all 42 turning points.
Moreover, we achieved high accuracy when it
comes to detecting different swimming styles (Table
2). The algorithm detected all crawl, breaststroke
and backstroke laps, however identifying butterfly
failed in 2 out of 42 laps. Taken together, true
positive rate reaches 99%.
Table 2: Stroke recognition (TP = True positive; FP =
False positive, TN = True negative; FN = False negative).
TP FP TN FN
Crawl 42/42 1/126 125/126 0/42
Breaststroke 42/42 1/126 125/126 0/42
Backstroke 42/42 0/126 126/126 0/42
Butterfly 40/42 0/126 126/126 2/42
Figure 3: The flow chart of the stroke recognition process,
together with used cut-off’s.
4 CONCLUSIONS
In this study we are demonstrating that swimming
events together with swimming styles may be
registered with high certainty using wireless 3D
accelerometers. Moreover, we show that the whole
process could be performed in online mode, which
could be beneficial for coaches when overviewing
training performance.
More outputs, such as stroke frequency, stroke
length, stroke duration etc., are to be developed.
Identifying those variables would complete the
online monitoring tool, providing the coach all the
necessary information to analyse and improve
swimmers training and performance. In practice,
whether such a system could be useful to the
coaches is not yet explored. However, having a
constant feedback on a swimming effort and
quantification of each motion can only provide
sufficient measurements for more efficient
performance (Smith et al. 2002). As addition,
suchlike monitoring tool should not be limited to
one swimmer, but secure a multi-swimmers
monitoring feature.
Substantial improvement of the developed
algorithm could be achieved by applying device and
algorithm on larger number of subjects. This would
either provide stronger validation of the monitoring
tool, or result in fine tuning of the used cut-off’s
which should again confirm high accuracy of the
algorithm for monitoring swimming performance.
ACKNOWLEDGEMENTS
We thank Brigitte Becque and swimmers from sport
school Leonadro Lyceum in Antwerp for the help
with the data collection.
REFERENCES
Auvinet, B., Gloria, E., Renault, G., & Barrey, E. 2002.
Runner's stride analysis: comparison of kinematic and
kinetic analyses under field conditions. Science &
Sports, 17, (2) 92-94
Bachlin, M. & Troster, G. 2012. Swimming performance
and technique evaluation with wearable acceleration
sensors. Pervasive and Mobile Computing, 8, (1) 68-
81
Jensen, U., Prade, F., & Eskofier, B. M. Classification of
kinematic swimming data with emphasis on resource
consumption, In Body Sensor Networks, IEEE, pp. 1-
5.
icSPORTS2014-InternationalCongressonSportSciencesResearchandTechnologySupport
114