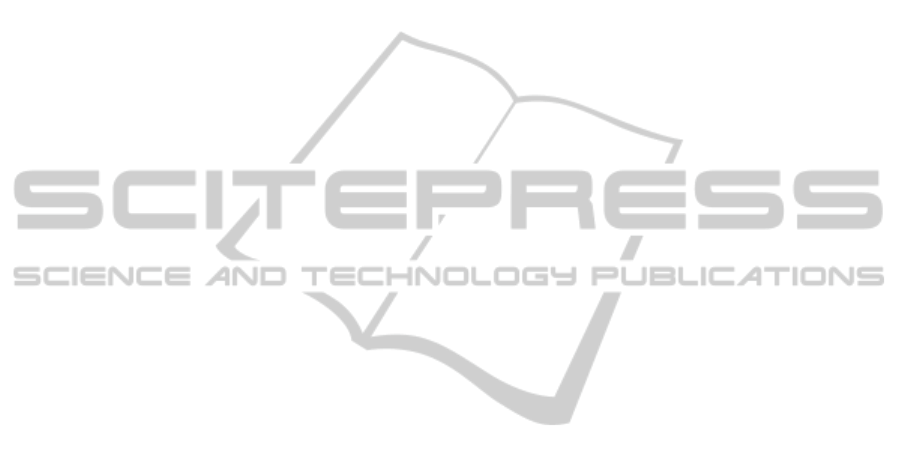
form of < opinion target expression, sentiment word,
contextual modifiers of sentiment word>.
1) Influence of Opinion Target Expression on
Polarity of Sentiment Phrase
Opinion targets that are noun or noun phrase
may exhibit oddity, and an odd opinion target will
change the polarity of the sentiment word that
modifies it. For example, the word '减少(decrease)'
generally exhibits negative polarity, and the phrase
'营业收入减少(the operating income decreases)'
exhibits negative polarity, while the phrase '损失减
少(the loss decreases)' exhibits positive polarity.
This is because the word ‘loss’ is an odd target.
For a opinion target composed of noun and verb,
sometimes this verb may also be a sentiment word.
For example, in sentences '股价上涨得很快(the
stock price rises rapidly)' and '股价下跌得很快(the
stock price drops rapidly)', the sentiment word
'rapidly' modifies 'stock price rises' and 'stock price
drops', respectively. At this time, the sentimental
polarity and intensity of the entire opinion target
expression need to be determined first.
2) Influence of Sentiment Word’s Contextual
Modifiers on Polarity of Sentiment Phrase
The contextual modifiers of sentiment words are
mainly negative adverbs and adverbs of degree, and
their influences on the polarity of sentiment phrases
include:
(1) Influence of negative adverbs on polarity of
sentiment words;
(2) Influence of adverbs of degree on polarity of
sentiment words;
(3) The distance between negative adverbs or
adverbs of degree and sentiment words is called edit
distance. When a negative adverb and an adverb of
degree modify the same sentiment word
simultaneously, different combinations of their edit
distances from the sentiment word result in different
influences on the polarity and intensity of the
sentiment word.
4 CONCLUSIONS
The beginning of the era of big data brings us both
opportunities and challenges. Applying data mining
to Web financial reviews, which contain abundant
information, could help with investors’ investment
decision-making, enterprise operators’ management
decision-making, as well as credit rating in the
finance and insurance industry.
However, the mining of Web financial reviews
faces many challenges, for example the diversity of
sentiment words’ parts of speech, the diversity of the
opinion targets expressions, and the complexity of
the construction of Web financial indexes, as well as
the sentimental quantification of Web financial
indexes caused by these three features. In the
meantime, this challenging task is very meaningful.
REFERENCES
Andrzejewski, D., Zhu, X. , and Craven M.(2009).
Incorporating domain knowledge into topic modeling
via Dirichlet forest priors. In ICML’09: Proceedings of
the International Conference on Machine Learning,
Montreal, Quebec, Canade, 14-18 June 2009. ACM,
25-32.
Bian, H., Wan, C., Liu, D., Jiang, T. (2013). A study of
financial crisis prediction model for listed companies
taking into account Web financial information. Computer
Science, 40(11), 295-298, 315. (in Chinese)
Bloom, K., Argamon, S. (2009). Automated learning of
appraisal extraction patterns. Language and
Computers, 71(1), 249-260.
Bloom, K., Garg, N., Argamon, S. (2007). Extracting
appraisal expressions. In EMNLP’07: Proceedings of
the Human Language Technology Conference and the
Conference on Empirical Methods in Natural
Language Processing, Prague, Czech Republic, 28-30
June 2007. ACL, 308-315.
Das, S., Chen, M. (2007). Yahoo! For Amazon: Sentiment
Extraction from Small Talk on the Web. Management
Science, 53(9),1375-1388.
Fang, L., Huang, M. (2012). Fine granular aspect analysis
using latent structural models. In ACL 2012:
Proceedings of the 50th Annual Meeting of the
Association for Computational Linguistics, Jeju,
Korea,8-14 July 2012. ACL, 333-337.
Feng, S., Fu, Y., Yang, F., et al., 2012. Blog sentiment
orientation analysis based on dependency parsing.
Journal of Computer Research and Development,
49(11), 2395-2406. (in Chinese)
Hu, M., Liu, B. (2004). Mining and summarizing
customer reviews. In KDD’04: Proceedings of the 10th
ACM International Conference on Knowledge
Discovery and Data Mining, Seattle, Washington,
USA, 22-25 August 2004. New York: ACM, 168-177.
Jakob, N., Gurevych, I. (2010). Extracting opinion targets
in a single and cross-domain setting with conditional
random fields. In EMNLP’10: Proceedings of
Conference on Empirical Methods in Natural
Language Processing. Cambridge, MA, 12-14
October 2010. ACL,1035-1045.
Jin, W., Ho, H., Srihari, R. (2009). OpinionMiner: a novel
machine learning system for Web opinion mining and
extraction. In KDD’09: Proceedings of ACM
International Conference on Knowledge Discovery and
Data Mining (SIGKDD), Paris, 19-21 September 2009.
New York: ACM, 1195-1204.
Kamal, A., Abulaish, M., Anwar, T. (2012). Mining
feature-opinion pairs and their reliability scores from
KDIR2014-InternationalConferenceonKnowledgeDiscoveryandInformationRetrieval
372