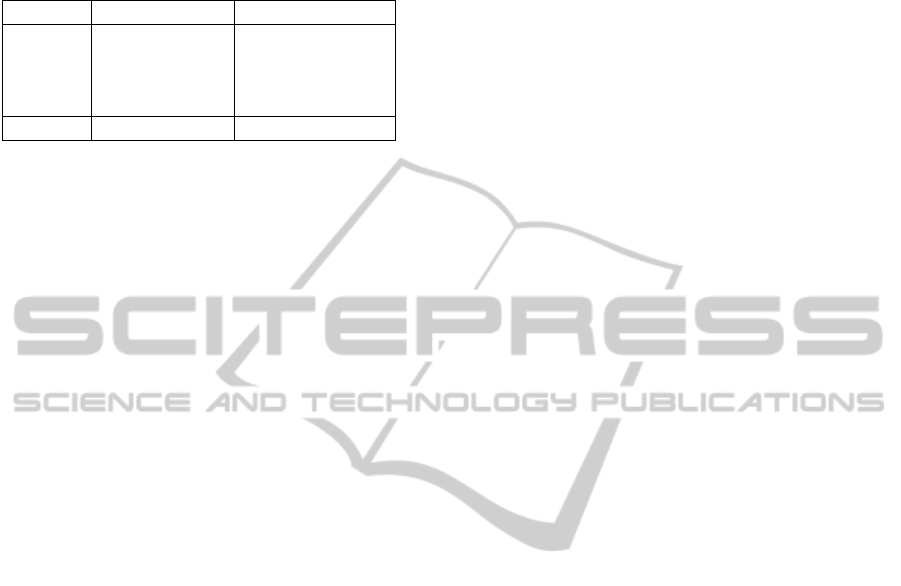
Table 1: Classification results of EMG-based prediction for
all subjects. The accuracy of the classification and the mean
time of EMG-based prediction inside the EMG-window
with the standard deviation.
Subject Accuracy (%) Time (ms)
1 70.00 −91.60 ± 91.30
2 46.67 −90.90 ± 130.70
3 93.33 −72.30 ± 46.20
4 57.67 −52.25 ± 51.40
Average 66.92 −76.74 ± 79.90
one and, mainly, for the subject three, is reached a
high level of accuracy. According to (Kirchner et al.,
2014), activations before the EMG-window were con-
sidered false positives. Taking into account all the
classes of classification, regardless of whether the de-
tection anticipates or not the movement onset or the
detection is classified before the EMG-window, 100%
of the trials were detected by the EMG classifier for
all subjects.
The difference of accuracy between the subjects is
directly related to the EMG time of activation, since
it defines the position of the EMG classification be-
tween the classes. The EMG time of activation de-
pends on many factors and vary between subjects and
trials, as it can be seen in Figure 9 and according to
the results achieved by (Kirchner et al., 2014).
The execution of the movement depends on many
motor unit-related parameters, e.g. twitch force, con-
tractile speed, axonal conduction velocity, fatigue re-
sistance, among others (Pons, 2008). Furthermore,
the surface electromyographic also depends on the
skin-electrode coupling which contributes to differ-
ent signals between subjects. Based on the study of
these characteristics, the length of the EMG-window
can be adapted for each subject. This can decrease the
number of wrong classifications of false positives. For
subjects whose accuracy is lower, the movement pre-
diction can be complemented by an EEG-based pre-
diction.
Depending on the type of neuromuscular disorder
and state of therapy, both, single EEG or single EMG-
based movement prediction, might no longer result in
good performance. In this case, the combination of
multimodal data should be even more relevant (Kirch-
ner et al., 2014).
5 CONCLUSIONS
A multimodal platform for lower limb studies in re-
habilitation based on EEG, EMG and IMU signals
was developed. An experimental protocol, applied
in healthy subjects to acquire data from the platform,
was also proposed. An offline analysis of the data
generated by this system in a lower limb movement
task was showed.
The analysis estimates the subject’s movement in-
tention evaluating the ERD characteristic in the EEG
signal and the muscle activation in the EMG signal.
The beginning of the movement was evaluated by
IMUs data, which also measure the angles between
the thigh and the shank of the subject during the tasks
performed.
The accuracy and the mean time showed the fea-
sibility of the interface proposed. Under a qualita-
tive analysis and based on the Figures 7, 8 and 9, the
system proposed was capable to acquire, synchronize
and process the combined signals. Thus, the platform
proposed can be used in the study and development of
multimodal interfaces. Prosthesis or orthoses whose
purpose is motor rehabilitation, adapting the control
of devices with respect to the patient’s intention, can
be developed with the platform proposed.
Results presented here were achieved in an offline
processing. In future work, a single trial algorithm
whose purpose is to analyze the EEG signal in order
to find MRPs will be applied. A platform which uses
online detection of EEG and EMG data will be ap-
plied to an exoskeleton, which is currently under de-
velopment.
REFERENCES
Den
`
eve, A., Moughamir, S., Afilal, L., and Zaytoon, J.
(2008). Control system design of a 3-DOF upper
limbs rehabilitation robot. Computer methods and
programs in biomedicine, 89(2):202–14.
Favre, J., Aissaoui, R., Jolles, B. M., de Guise, J. a., and
Aminian, K. (2009). Functional calibration procedure
for 3D knee joint angle description using inertial sen-
sors. Journal of biomechanics, 42(14):2330–5.
Gallego, J. A., Ib
´
a
˜
nez, J., Dideriksen, J. L., Serrano, J. I.,
del Castillo, M. D., Farina, D., and Rocon, E. (2012).
A multimodal human–robot interface to drive a neu-
roprosthesis for tremor management. IEEE Transac-
tions on Systems, Man, and Cybernetics, 42(6):1159–
1168.
Hermens, H. J., Freriks, B., Merletti, R., Stegeman, D.,
Blok, J., Rau, G., Disselhorst-Klug, C., and H
¨
agg, G.
(1999). European recommendations for surface elec-
tromyography.
Ib
´
a
˜
nez, J., Serrano, J., del Castillo, M., Gallego, J., and Ro-
con, E. (2013). Online detector of movement inten-
tion based on EEG—Application in tremor patients.
Biomedical Signal Processing and Control, 8(6):822–
829.
Ju, M.-S., Lin, C.-C. K., Lin, D.-H., Hwang, I.-S., and
Chen, S.-M. (2005). A rehabilitation robot with force-
position hybrid fuzzy controller: hybrid fuzzy control
NEUROTECHNIX2014-InternationalCongressonNeurotechnology,ElectronicsandInformatics
54