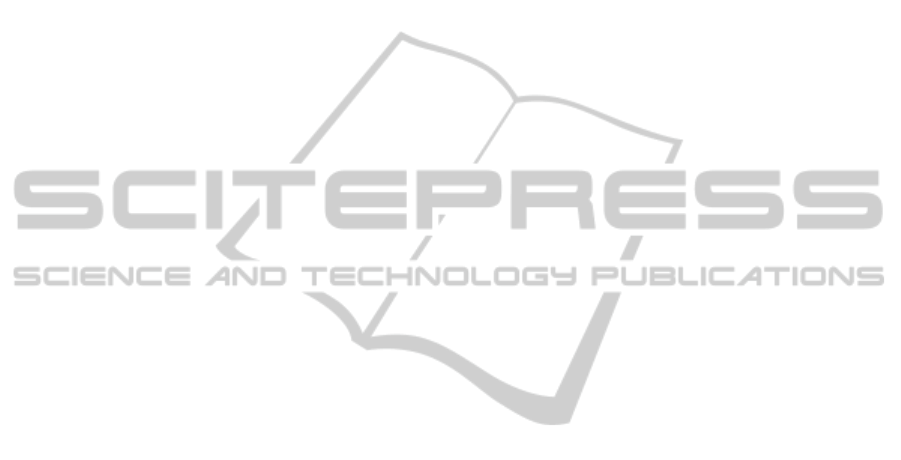
Gay, C., Sánchez, O., Martínez-López, B., Nébot, Á.,
Estrada, F. 2012. Simple Fuzzy Logic Models to
Estimate the Global Temperature Change due to GHG
Emissions. 2nd International Conference on
Simulation and Modeling Methodologies,
Technologies and Applications (SIMULTECH).
Special Session on Applications of Modeling and
Simulation to Climatic Change and Environmental
Sciences - MSCCEC 2012. July 28-31. Rome, Italy.
Thomson Reuters Conference Proceedings Citation
Index (ISI), INSPEC, DBLP and EI (Elsevier Index)
http://www.informatik.uni-
trier.de/~ley/db/conf/simultech/simultech2012.html.
Gay García, C., O. Sánchez Meneses. 2013. Natural
Handling of Uncertainties in Fuzzy Climate Models.
3rd International Conference on Simulation and
Modeling Methodologies, Technologies and
Applications (SIMULTECH). Special Session on
Applications of Modeling and Simulation to Climatic
Change and Environmental Sciences - MSCCEC
2013. July 29-31. Reykjavík, Iceland. Thomson
Reuters Conference Proceedings Citation Index (ISI),
INSPEC, DBLP and EI (Elsevier Index) Abstracts in:
http://www.simultech.org/Abstracts/2013/MSCCEC_2
013_Abstracts.htm.
Gay, C., Sánchez, O., Martínez-López, B., Nébot, Á.,
Estrada, F. 2013. Fuzzy Models: Easier to Understand
and an Easier Way to Handle Uncertainties in Climate
Change Research. In: Simulation and Modeling
Methodologies, Technologies and Applications.
Volume Editor(s): Pina, N., Kacprzyk, J. and Filipe, J.
In the series "Advances in Intelligent and Soft
Computing". Springer- Verlag GmbH Berlin
Heidelberg.
Giorgi, F., Mearns, L.O. 2002. Calculation of Average,
Uncertainty Range, and Reliability of Regional
Climate Changes from AOGCM Simulations via the
‘‘Reliability Ensemble Averaging’’ (REA) Method.
Journal of Climate, Vol. 15; 1141-1158.
IPCC-WGI, 2013: Climate Change 2013: The Physical
Science Basis. Contribution of Working Group I to the
Fifth Assessment Report of the Intergovernmental
Panel on Climate Change [Stocker, T. F., D. Qin, G.-
K. Plattner, M. Tignor, S. K. Allen, J. Boschung, A.
Nauels, Y. Xia, V. Bex and P. M. Midgley (eds.)].
Cambridge University Press, Cambridge, United
Kingdom and New York, NY, USA, 1535 pp.
IPCC-WGI, 2007: Climate Change 2007: The Physical
Science Basis. Contribution of Working Group I to the
Fourth Assessment Report of the Intergovernmental
Panel on Climate Change [Solomon, S., D. Qin, M.
Manning, Z. Chen, M. Marquis, K. B. Averyt, M.
Tignor and H. L. Miller (eds.)] Cambridge University
Press, Cambridge, United Kingdom and New York,
NY, USA, 996 pp.
IPCC-WGI, 2001: Climate Change 2001: The Scientific
Basis, Contribution of Working Group I to the Third
Assessment Report of the Intergovernmental Panel on
Climate Change, [Houghton, J. T.; Ding, Y.; Griggs,
D. J.; Noguer, M.; van der Linden, P. J.; Dai, X.;
Maskell, K.; and Johnson, C. A., ed.] Cambridge
University Press, ISBN 0-521-80767-0, (pb: 0-521-
01495-6).
Meinshausen, M., S. C. B. Raper and T. M. L. Wigley
(2011). "Emulating coupled atmosphere-ocean and
carbon cycle models with a simpler model,
MAGICC6: Part I – Model Description and
Calibration." Atmospheric Chemistry and Physics 11:
1417-1456. doi:10.5194/acp-11-1417-2011.
Wehner, M. F. 1998. Determination of the Sampling Size
of AGCM Ensemble Simulations. Program for
Climate Model Diagnosis and Intercomparison
(PCMDI) Report No. 47. 24 pp. (On line: http://www-
pcmdi.llnl.gov/publications/pdf/47.pdf).
Wigley T. M. L. 2008. MAGICC/SCENGEN V. 5.3: User
Manual (version 2). NCAR, Boulder, CO. 80 pp. (On
line: http://www.cgd.ucar.edu/cas/wigley/magicc/).
Zadeh, L. A. 1965. Fuzzy Sets: Information and Control.
Vol. 8(3) p. 338-353.
TheFuzzyNatureofClimateChangeScenariosMaps
873