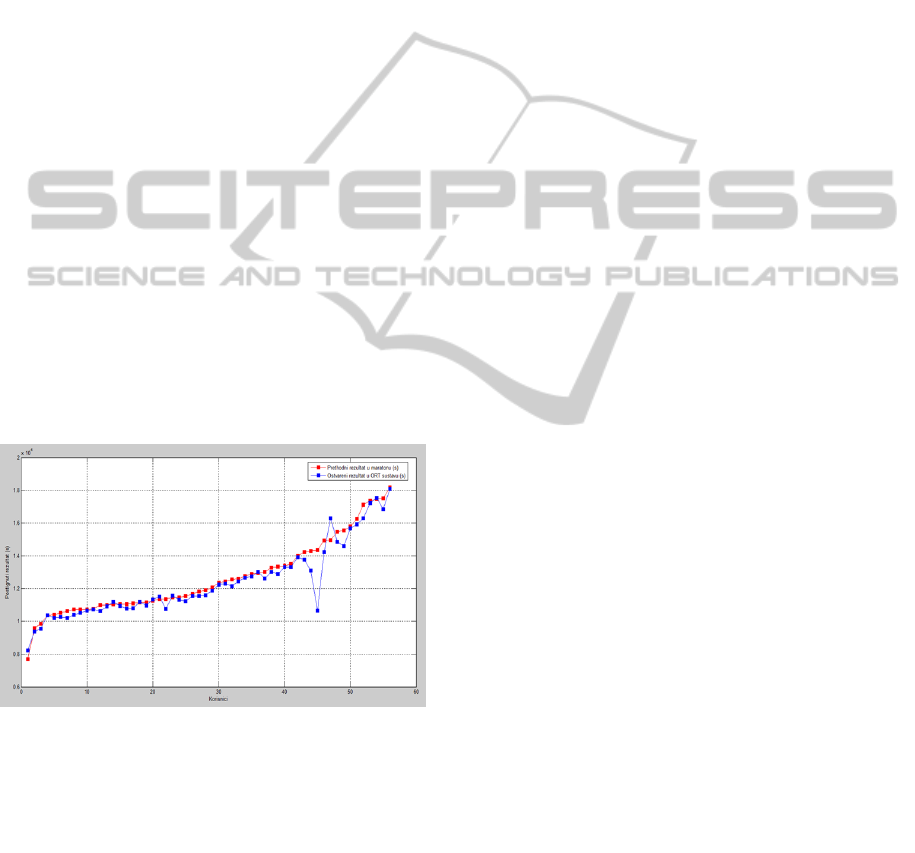
Periodic control trainings are envisaged based on
which one can monitor and calculate new values of
maximum aerobic capacity VO2
max
. Each positive
(or negative) change is dynamically in calculated in
all remaining training sessions, by means of which
one attains improvement in remaining preparation
period.
Developed prototype searches for similar runner
profile patterns, and based on known history of their
accomplishments forms and displays corresponding
corrections, generating individualized program for
preparation (Figure 1).
By filling data warehouse for even greater
sample of runners in long periods, conditions are
created for reliable prediction of future realizations
and timely correction of preparation program.
3 RESULTS
The majority of tested marathon runners train many
years and have achieved results in marathon, which
were compared with the results that were achieved
with the assistance of developed Online Running
Trainer (ORT) system. The comparison between
previous personal bests (achieved in the past three
years) and those achieved by using the implemented
system is shown in Figure 2.
Figure 2: The comparison between previous and current
results of tested users (in Croatian language).
X-axis of the Figure 2 represents tested users (58
marathon runners), while the y-axis represents the
achieved results of those runners in seconds. The red
curve in the Figure 2 shows previous results in
marathon, while the blue curve shows achieved
results with the ORT system. It can be seen that
almost all tested users have improved their results
and have successfully finished the training cycle
without sport injuries. It is important to mention that
they have not reached that result in several iterations
(training cycles), but within one season in which
they have been systematically guided.
4 DISCUSSION
Mentioned verification process has its flaws and was
not carried out in a way which would (possibly)
show more (or less) deviations from the anticipated
results. Only one group of athletes (58 athletes) was
tested, who used the developed system and ran on
their key races. The results of those runners in the
past three years, achieved without the help from the
developed prototype were compared with the results
with the assistance of ORT system, where there is
only partially possible to compare the parameters of
“different” groups of users who train in different
ways. Because of the specificity of testing sport
achievements, the comparison between the group of
marathon runners and the group of people who do
not actively engage in sport activities (running)
would not have any practical value. Listed
limitations of the conducted research should result in
additional evaluations, for the purpose of obtaining
quality and more certain judgments on a larger
sample of athletes. Periodic loading of data
warehouse (ETL process) will, in time, enable the
evaluation of algorithms and methods on a larger
data sample, while the developed ORT system will,
by the method of self-learning, correct the training
elements and generate better quality programs.
5 CONCLUSIONS
Machine learning means a computing method
enabling future actions be improved based on
information from past (Gamberger, 2011). Presented
method, further, is based not only on the study of
realization by one user, but the system learns as a
whole based on registered data on remaining
runners. This demonstrates that presented method
successfully implements machine learning paradigm
to the problem of improving sports training.
REFERENCES
Milanović, D., 2009., Theory and methodology of
trainings (in Croatian), Faculty of Kinesiology,
University of Zagreb, Zagreb.
Havaš, L., Vlahek P., 2006. Road running (in Croatian),
TK Međimurje. Čakovec.