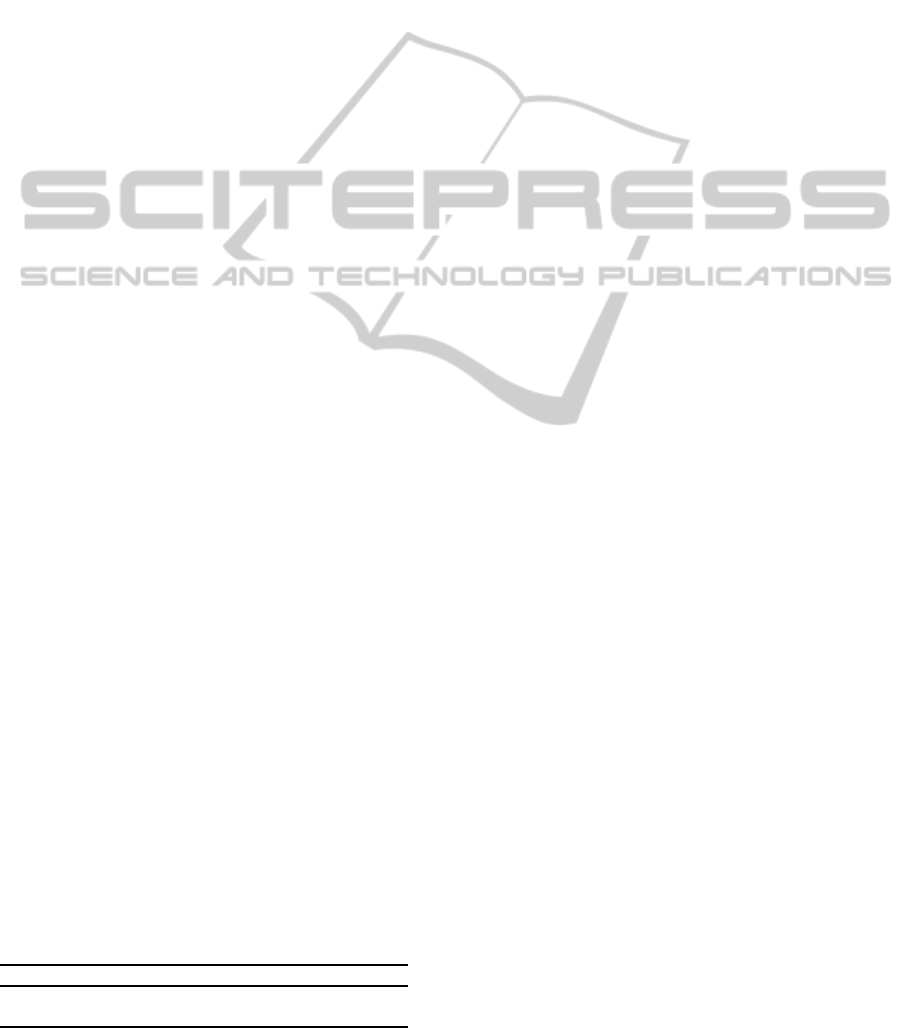
mean value for the mth lead selected by the model
from the complete set of 8 leads. A backward elimi-
nation method that discards the least significant vari-
able at each iteration according to the Wald index was
employed (Indrayan, 2012) to keep only the most rel-
evant ECG leads.
On the other hand, SVM separates the given set of
binary labeled training data with a hyperplane that is
maximally distant (Euclidean distance) from the two
classes success and failure CA outcome). Linear dis-
criminant functions define a decision hyperplane in a
multidimensional feature space as:
g(x) = w
T
x+ w
0
= 0 (2)
where w is known as the weight vector and x is the
feature vector (set of lead amplitude values). Symbol
w
0
denotes the threshold and (·)
T
the transpose op-
erator. Note that elements in vector w close to zero
have little influence on g(x) and hardly contribute to
the decision. In (Guyon et al., 2002) it was suggested
a recursive feature elimination technique (SVM-RFE)
that discards iteratively the features corresponding to
the lowest values of |w
i
|. After feature elimination,
the classifier is evaluated using a leave-one-out cross-
validation strategy. In our case, the initial feature vec-
tor x is made up of the mean fibrillatory amplitude
values computed in each of the 8 leads considered
in this study. The SVM-RFE algorithm was imple-
mented using MATLAB version R2011a.
3 RESULTS
No significant inter-class differences were found for
any of the single-lead amplitude parameters consid-
ered separately.
After applying LR by using the backward elimi-
nation method, the remaining leads were I, V1, V2
and V5. Table 1 summarizes the best results in terms
of AUC by applying univariate and multivariate ap-
proaches. The best cut-off point was θ = 0.7 yielding
83% sensitivity, 73.3% specificity and 80.6% total ac-
curacy.
The SVM-RFE algorithm ranked the leads as fol-
lows: I, V5, V2, V1, II, V4, V6 and V3 (ordered from
Table 1: Best accuracy results by the univariate contrast
with the highest AUC and the multivariate analysis after
LR backward elimination. AUC: area under the curve; IC:
AUC’s interval of confidence; p: AUC’s statistical signifi-
cance.
Analysis AUC IC p
Univariate (lead I) 0.678 0.50 to 0.85 0.039
Multivariate (θ) 0.854 0.75 to 0.96 <0.001
the most to the least relevant). The best result in terms
of accuracy is obtained by using only the three most
relevant leads (I, V5 and V2) reaching up to 82.3%
accuracy, 70% specificity and 84.6% sensitivity.
4 CONCLUSION
This work has shown that analyzing the fibrilla-
tory amplitudes measured in multiple ECG leads im-
proves the accuracy of previous CA outcome pre-
dictors based on a single lead (Nault et al., 2009).
The multivariate amplitude analysis model reaches
an AUC of up to 0.854 with four leads. Both LR
and SVM-RFE agree in pointing to I, V1, V2 and
V5 as the most discriminant leads to determine mid-
and long-term CA outcome. Along the lines of (Meo
et al., 2013), these results support the suitability of
taking into consideration the information of several
leads that are often neglected in CA outcome predic-
tion and AF analysis (Nault et al., 2009; Matsuo et al.,
2009).
ACKNOWLEDGEMENTS
Work supported in part by the French National
Research Agency under contract ANR-2010-JCJC-
0303-01 “PERSIST” and the Centre Scientifique de
Monaco. A. R. Hidalgo-Mu˜noz holds a postdoctoral
research fellowship awarded by Univ. Nice Sophia
Antipolis.
REFERENCES
Burges, C. (1998). A tutorial on support vector machines
for pattern recognition. Data Mining and Knowledge
Discovery, 2(2):121–167.
Calkins, H., Kuck, K. H., Cappato, R., Brugada, J., Camm,
A. J., et al. (2012). 2012 HRS/EHRA/ECAS expert
consensus statement on catheter and surgical ablation
of atrial fibrillation: recommendations for patient se-
lection, procedural techniques, patient management
and follow-up, definitions, endpoints, and research
trial design. Europace, 14:528–606.
Guyon, I., Weston, J., Barnhill, S., and Vapnik, V. (2002).
Gene selection for cancer classification using support
vector machines. Machine Learning, 46:389–422.
Indrayan, A. (2012). Medical Biostatistics. CRC Press.
Jones, A. R., Krummen, D. E., and Narayan, S. M. (2013).
Non-invasive identification of stable rotors and focal
sources for human atrial fibrillation: mechanistic clas-
sification of atrial fibrillation from the electrocardio-
gram. Europace, 15(9):1249–1258.