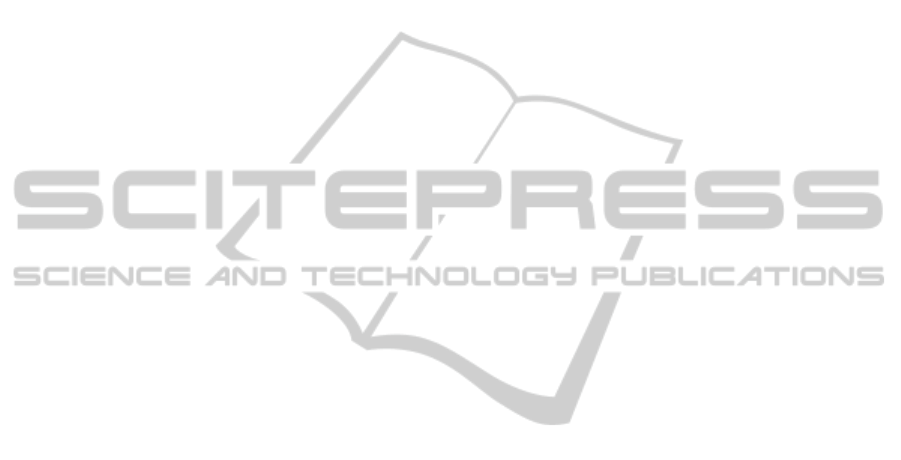
number of parts (up to 7). No changes were applied to
the default settings of Morphessor and corresponding
morpheme division. SWER stands for the sub-word
ER. The RTF for syllable LMs is 0.58, for morpheme
LMs - 0.45 and for MrphS LM - 0.49.
As can be seen, the best sub-word recognition ac-
curacy was achieved for syllable LMs, since the lexi-
con size is relatively small and each Russian syllable
consists obligatory of one vowel which usually pro-
nounced longer and is easier to recognize. However,
after straight-forward word synthesis the ”base+affix”
MrphA LM turns to be the best choice for very large
vocabulary Russian ASR despite of high perplexity
(PP). It’s also worth mentioning, that OOV-rate on the
development and test sets was non-zero for morpheme
LMs only. Other LMs were able to find the appropri-
ate sub-word units to cover the unknown words. In-
teresting result is that the inclusion of prefixes into
the morpheme LM decreases dramatically the recog-
nition accuracy. This is explained by the small size of
prefixes on average and by the fact, that lots of Rus-
sian prefixes do not include vowel sounds. The same
explanation is valid for MrphS LM: the vast amount
of small sub-words without vowels in the model.
5 CONCLUSION AND FUTURE
WORK
In this study different sub-word LMs were compared.
The language and acoustic training were conducted
under the same conditions, using the same textual and
speech material. The ”stem+affix” morpheme model
outperformed significantly other LMs being an opti-
mum trade-off between the number of sub-words and
their size. Relatively low recognition accuracy is ex-
plained by the highly inflective nature of Russian and
conforms with the results of other state-of-the-art re-
search for Russian SR (Karpov et al., 2011). The
word forms of one lemma in Russian sound often very
similar making them hard to distinguish even for a
human listener. Quite relaxed word order constraints
complicates the statistical modeling of word inflec-
tion.
The graphone LMs (Shaik et al., 2011) were
not investigated in this study. However, they could
achieve the better recognition accuracy for Russian
and should be tested in the future. As shown in (El-
Desoky et al., 2009) it is better for sub-word LM to
not decompose the N most frequent words. In our ex-
periments all the decomposable words were split into
parts that resulted in the low WER but this was done
on purpose to compare the LMs under similar condi-
tions.
As shown in our previous work (Zablotskiy et al.,
2011a), it is possible to concatenate the sub-words
even without any markers using the genetic global
search algorithm capable to correct some SR errors.
However, for this algorithm to work the relatively
small SWERs are required. From this perspective, the
syllable LM is the most suitable for Russian LVCSR.
ACKNOWLEDGEMENTS
This work is partly supported by the DAAD (German
Academic Exchange Service).
REFERENCES
A. Stolcke, J. Zheng, W. W. and Abrash, V. (2011). SRILM
at sixteen: Update and outlook. In Proc. IEEE Auto-
matic Speech Recognition and Understanding Work-
shop, Waikoloa, Hawaii.
Arsoy, E., Can, D., Parlak, S., Sak, H., and Saras¸lar, M.
(2009). Turkish broadcast news transcription and re-
trieval. IEEE Transactions on Audio, Speech and Lan-
guage Processing, 17(5).
Bisani, M. and Ney, H. (2005). Open vocabulary speech
recognition with flat hybrid models. In Proc. of the
European Conf. on Speech Communication and Tech-
nology (Eurospeech’05), pages 725–728, Lisbon (Por-
tugal).
Bogdanov, D., Bruhtiy, A., Krivnova, O., Podrabinovich,
A., and Strokin, G. (2003). Organizational Con-
trol and Artificial Intelligence, chapter Technology
of Speech Databases Development (in Russian), page
448. Editorial URSS.
Byrne, W., Haji
ˇ
c, J., Ircing, P., Krbec, P., and Psutka, J.
(2000). Morpheme based language models for speech
recognition of Czech. In Sojka, P., Kopecek, I., and
Pala, K., editors, Text, Speech and Dialogue, volume
1902 of Lecture Notes in Computer Science, pages
139–162. Springer Berlin / Heidelberg.
Carnegie Mellon University (2012). CMUSphinx. Open
source toolkit for speech recognition.
Chen, S. F. and Goodman, J. (1998). An empirical study of
smoothing techniques for language modeling. Techni-
cal Report TR-10-98, Computer Science Group, Har-
vard University.
Creutz, M. and Lagus, K. (2005). Unsupervised mor-
pheme segmentation and morphology induction from
text corpora using morfessor 1.0. Technical Report
A81, Helsinki University of Technology.
El-Desoky, A., Gollan, C., Rybach, D., Schlter, R., and Ney,
H. (2009). Investigating the use of morphological de-
composition and diacritization for improving Arabic
LVCSR. In Proc. of the 10th Annual Conference of
the International Speech Communication Association
(Interspeech’09), Brighton (UK).
RussianSub-WordBasedSpeechRecognitionUsingPocketsphinxEngine
843