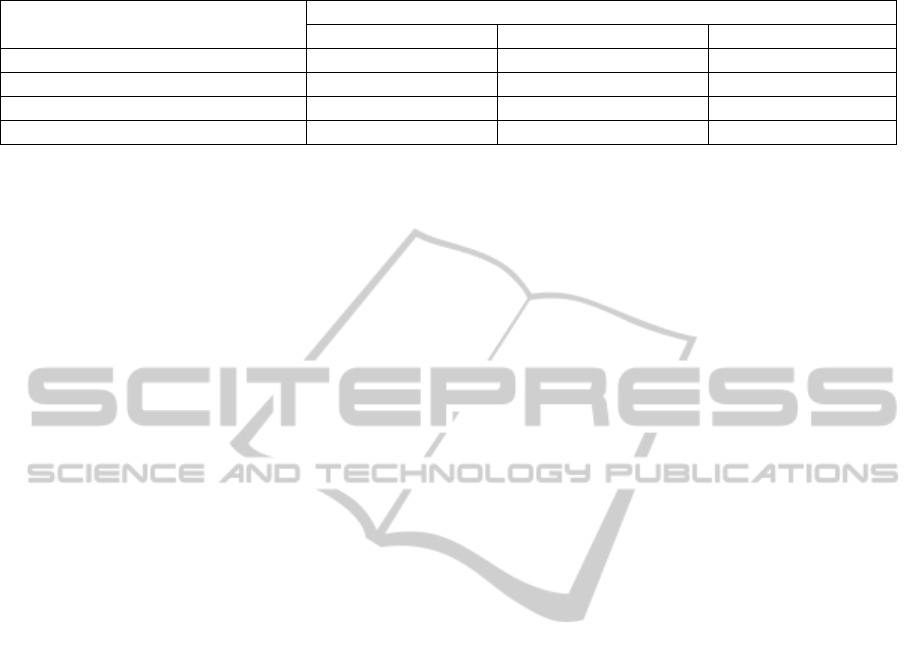
Table 2: Experimental results.
Method
Relative classification accuracy, %
Berlin SAVEE VAM
PNN 58.9 (384) 47.3 (384) 67.1 (384)
PCA-PNN 43.7 (129.3) 26.5 (123.6) 59.4 (148.6)
SPEA_wrapper-PNN 71.5 (68.4) 48.4 (84.1) 70.6 (64.8)
SPEA_filter-PNN 76.2 (138.6) 60.8 (142.0) 73.2 (152.8)
validation sample (20% of the training data set).
Table 2 contains the relative classification
accuracy for the presented corpora. In parentheses
there is the average number of selected features.
4 CONCLUSION
This study reveals advantages of using the MOGA-
PNN combination in feature selection by the
example of the speech-based emotion recognition
problem. The developed algorithmic schemes allow
not only a reduction in the number of features used
by the PNN-classifier (from 384 up to 64.8) but also
an improvement in its performance up to 29.4%
(from 58.9% on the full data set to 76.2% on the set
of selected features).
It was found that the filter approach permits the
achievement of better results in the sense of the
classification accuracy, whereas the usage of the
wrapper approach decreases the number of features
significantly.
Moreover, we compared these heuristic
procedures with conventional PCA with the 0.95
variance threshold. According to the obtained
results, the heuristic search for feature selection in
the emotion recognition problem is much more
effective than the application of the PCA-based
technique that leads to a decrease in the
classification accuracy.
Although the PNN accuracy is rather high, there
is an opportunity to investigate the self-adaptive
multi-objective genetic algorithm hybridized with
more accurate classifiers. Besides, other effective
MOGAs might be used to improve the performance
of the proposed technique.
REFERENCES
Boersma, P., 2002. Praat, a system for doing phonetics by
computer. Glot international, 5(9/10): pp. 341–345.
Burkhardt, F., Paeschke, A., Rolfes M., Sendlmeier, W. F.,
Weiss, B., 2005. A database of german emotional
speech. In Interspeech, pp. 1517–1520.
Daridi, F., Kharma N., Salik, J., 2004. Parameterless
genetic algorithms: review and innovation. IEEE
Canadian Review, (47): pp. 19–23.
Eiben A.E., Hinterding R., and Michalewicz Z., 1999.
Parameter control in evolutionary algorithms. IEEE
Transactions on Evolutionary Computation, 3(2): pp.
124–141.
Eyben, F., Wöllmer, M., and Schuller, B., 2010.
Opensmile: the Munich versatile and fast opensource
audio feature extractor. In Proceedings of the
international conference on Multimedia, pp. 1459–
1462. ACM.
Grimm, M., Kroschel, K., Narayanan, S., 2008. The vera
am mittag german audio-visual emotional speech
database. In Multimedia and Expo, 2008 IEEE
International Conference on, 865–868. IEEE.
Haq, S., Jackson, P., 2010. Machine Audition: Principles,
Algorithms and Systems, chapter Multimodal Emotion
Recognition, 398–423. IGI Global, Hershey PA.
Kohavi, R., John, G.H., 1997. Wrappers for feature subset
selection. Artificial Intelligence, 97: pp. 273-324.
Specht, D.F., 1990. Probabilistic neural networks. Neural
networks, 3(1): pp. 109–118.
Venkatadri, M., Srinivasa Rao, K., 2010. A multiobjective
genetic algorithm for feature selection in data mining.
International Journal of Computer Science and
Information Technologies, vol. 1, no. 5, pp. 443–448.
Zitzler, E., Thiele, L., 1999. Multiobjective evolutionary
algorithms: A comparative case study and the strength
pareto approach, Evolutionary Computation, IEEE
Transactions on, vol. 3, no. 4, pp. 257–271.
AcousticEmotionRecognition-TwoWaysofFeaturesSelectionbasedonSelf-AdaptiveMulti-ObjectiveGenetic
Algorithm
855