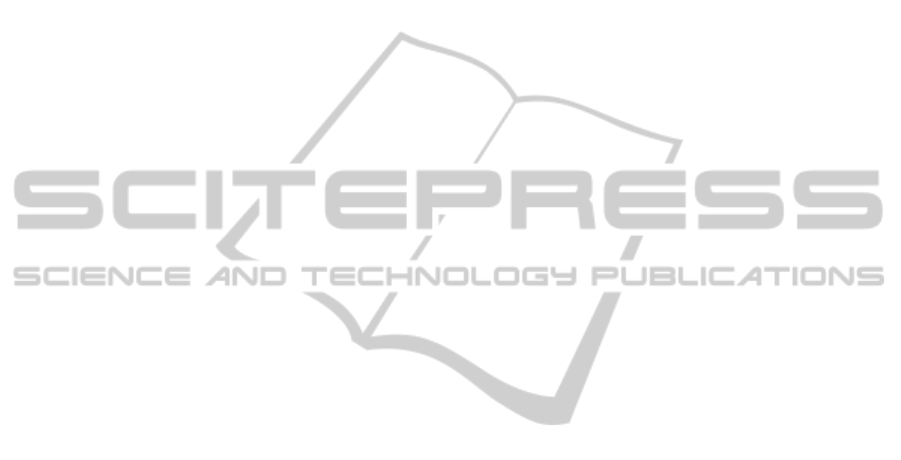
It is pointed here that such phenomena have been
well established in engineering and science, as for
example in some dynamic-chaotic systems that are
highly sensitive to initial conditions. In these, a
small perturbation of the initial conditions could
drive the system to totally different extremes
(behaviour).
As pointed, this second claim is a counter-
generalization observation, which may have a
profound negative impact on the development of
ANNs, especially for critical applications such as in
critical medical diagnostic and other systems such as
in autonomous/driverless cars and other vehicles, in
crucial google glass applications, in salvage
operations, in critical/sensitive military applications,
etc.
Here are some more specific examples that may
make one think twice before relying on feedforward
ANNs for decisions:
A self-driving/autonomous car that uses an
ANN (e.g. deep neural network) does not
recognize a human standing in front of the
car. It may interpret the road as clear,
resulting in highly risky and dangerous
situations for pedestrians.
An ANN that is used in a critical medical
diagnostic operation that misclassifies as a
false positive a specific cancer image or
medical signal.
An ANN that is used in military operations
and misclassifies a building as having
terrorists that should be bombed!
A prisoner convicted to death penalty, where
the realization of this verdict depends on
his/her IQ being above a certain threshold,
which had been wrongly established by an
ANN (e.g. case of Ted Herring in Florida
State, USA).
An interesting issue that comes to mind is
whether such “blind spots” also exist in biological
neural networks. We know that certain blind spots
(static or dynamic) have been observed, e.g. the
attentional blink (Marois et al. 2000; Neokleous et al
2009). This occurs in a large number of individuals.
That is, it has a high statistical significance, but it is
not universal. That is, we may speculate that some
biological neural networks express a uniformly blind
spot.
Even though, for most people, the brain has an
impressive capacity to recognize images in diverse
orientations, lighting conditions, deformations,
modifications, perturbations etc., may occasionally
make wrong classifications, generalizations,
interpretations. It can even properly identify words
in the well-known Cambridge University
observation, popularized by the following extract:
“The phaonmneal pweor of the hmuan mnid,
aoccdrnig to a rscheearch at Cmabrigde Uinervtisy,
it dseno't mtaetr in waht oerdr the ltteres in a wrod
are, the olny iproamtnt tihng is taht the frsit and lsat
ltteer be in the rghit pclae. The rset can be a taotl
mses and you can sitll raed it whotuit a pboerlm.
Tihs is bcuseae the huamn mnid deos not raed ervey
lteter by istlef, but the wrod as a wlohe”.
If it is a fact that the biological brains may
misclassify, misinterpret, miscalculate and
misunderstand, then this creates numerous legal,
ethical, and philosophical questions.
Concerning the claim for the existence of
adversarial examples, we suggest the following
criticism.
a) This is a premature claim. The
SZSBEGF2014 researchers have tested a
large number of ANN structures, but they
were all of feedforward topology. They did
not say whether they have also tested
recurrent structures (dense or sparse). How
could we know whether similar behavior
occurs in artificial neural structures that
have recurrences? We know, for instance
that biological neural networks, and more
profoundly the human brain, are highly
recurrent structures. Thus, more
investigation into this issue is needed.
b) Even though one can conduct extensive
simulations on diverse networks, there will
still be gray areas, unless one manages to
prove in a coherent - preferably
mathematical formalism - that the blind
spots are universal to all network structures.
So, here is a new research field for
exceptional theoreticians!
c) Considering the cases of blind spots in
biological neural recognizing systems such
as the human brain, one can observe that
these blind spots are not universal. Indeed,
one can find human brains that correctly
identify images that they were erroneously
mislabeled by a large population. Thus,
there may be ANNs that can correctly
identify adversarial examples. It is rather a
matter of finding these networks.
In any case, as it is, it should make us very
cautious in building critical applications in which
ANNs are embedded, e.g. in medical diagnostic
systems for critical diseases.
NCTA2014-InternationalConferenceonNeuralComputationTheoryandApplications
308