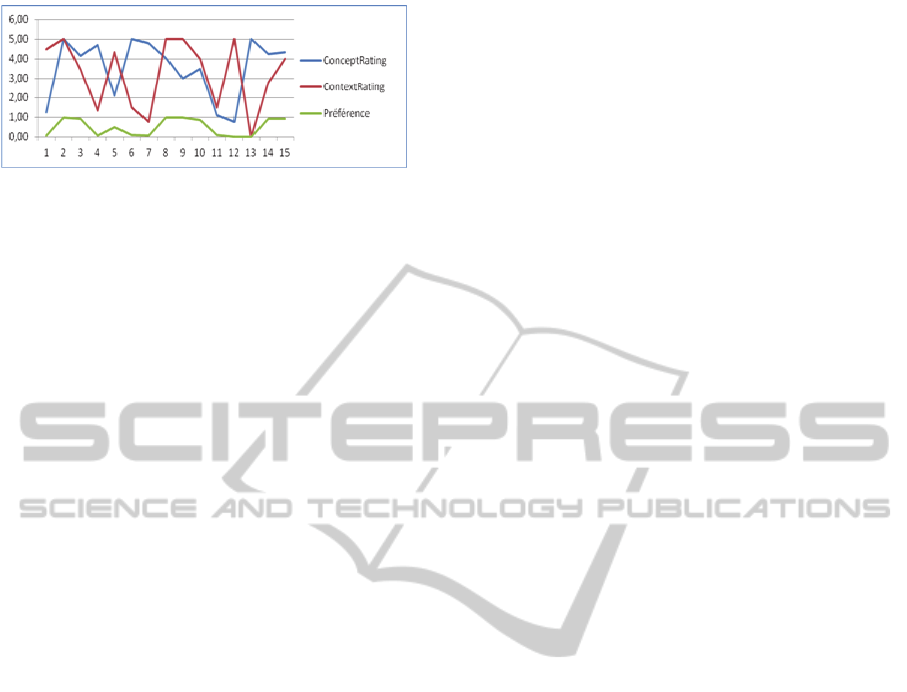
Figure 6: Context impact on user preferences decision.
5 CONCLUSION
In this paper we present our personalized image
retrieval system based on user profile modeling
depending on user’s context. We adopt a fuzzy
logic-based user profile modeling due to its
flexibility in decision making. This model work with
a list of concept and context where the user is asked
to rate them according to his interest and these rates
help in predicting the preference degree related to
each concept for such context. As for as the
experiments, the advanced user profile modeling
with fuzzy logic shows more flexibility in the
interpretation of the query compared with the
standard boolean model. For future work, we aim to
make the context detection automatic without user
intervention and the same thing for the concept
rating where the rate value will be deduced from the
user profile extracted from social network.
ACKNOWLEDGEMENTS
The authors would like to acknowledge the financial
support of this work by grants from General
Direction of Scientific Research (DGRST), Tunisia,
under the ARUB program.
REFERENCES
Hanjalic.A, New grand challenge for multimedia
information retrieval:bridging the utility gap,
International Journal of Multimedia Information
Retrieval, 2012
Ksibi.A Feki.G, Ben Ammar.A, Ben Amar.C Effective
Diversification for Ambiguous Queries in Social
Image Retrieval. CAIP (2) 2013: 571-578.
Gemmell,J Schimoler,T. Mobasher,B. Burke,R. 2011.
Tag-Based Resource Recommendation in Social
Annotation Applications UMAP 2011, LNCS 6787,
pp. 111–122, 2011.
Gemmell, J., Schimoler, T., Mobasher, B., Burke, R.2010
Hybrid tag recommendation for social annotation
systems. In: 19th ACM International Conference on
Information and Knowledge Management, Toronto,
Canada (2010)
Pitsilis.G , Knapskog. Svein J, Social Trust as a solution
on address sparsity-inherent problems of
Recommender systems, ACM RecSys 2009,
Workshop on Recommender Systems & The Social
Web, New York, USA. 2009
Feki.G, Ksibi.A, Ben Ammar.A, Ben Amar.C. Improving
image search effectiveness by integrating contextual
information. CBMI 2013: 149-154.
Feki.G, Ksibi.A, Ben Ammar.A, Ben Amar.C. REGIMvid
at ImageCLEF2012: Improving Diversity in Personal
Photo Ranking Using Fuzzy Logic. CLEF 2012.
Kalervo Järvelin and Jaana Kekäläinen. 2002. Cumulated
gain-based evaluation of IR techniques. ACM Trans.
Inf. Syst. 20, 4 (October 2002), p422-446.
Nowacka.K, Zadrozny.S Kacprzyk.J, An experimental
comparison of various aggregation operators in a
fuzzy information retrieval model, In proceeding of:
Fuzzy Information Processing Society, 2008
Kirchhoff.L, 2010, Thesis: Applying Social Network
Analysis to Information Retrieval on the World Wide
Web: A Case Study of Academic Publication Space
,University of St. Gallen , Germany, 2010
Oussalah.M and Eltigani.A, Personalized Information
Retrieval system in the Framework of Fuzzy Logic,
2005
Ghaderi. M Ali, Yazdani.N, Moshiri.B, A Social Network-
based Meta Search Engine, Information Retrieval,
2010 , p744-749
Fakhfakh.R, Feki.G, Ksibi.A, Ben Ammar.A, Ben
Amar.C, REGIMvid at ImageCLEF2012: Concept-
based Query Refinement and Relevance-based
Ranking Enhancement for Image Retrieval, CLEF
(Online Working Notes/ Labs/ Workshop), 2012.
Fakhfakh.R, Ksibi.A, Ben Ammar.A, Ben Amar.C,
Enhancing query interpretation by combining textual
and visual analyses, International Conference on
Advanced Logistics and Transport (ICALT), 2013,
p170-175
Sharma,N. Sharma,M. Gupta,O. 2012. Search Engine
Personalization Using Concept Based User Profiles.
International Journal of Scientific Research
Engineering &Technology (IJSRET) Volume 1 Issue4
pp 084-087 July 2012
Shen X., Tan B., and Zhai C.2005. Implicit user modeling
for personalized search. In Proc. Int. Conf. on
Information and Knowledge Management, 2005, pp.
824–831.
Silvia.C and Elie.S , A Fuzzy Ontology-Approach to
improve Semantic Information Retrieval, Workshop
on Uncertainty Reasoning for the Semantic Web
Busan, Korea, November 12, 2007.
Yang.L , Jian.S, Jun.X, Fei.W, Yueting.Z, Hypergraph
Spectral Hashing for image retrieval with
heterogeneous social contexts, Neurocomputing 119
(2013) 49–58, 2013
KDIR2014-InternationalConferenceonKnowledgeDiscoveryandInformationRetrieval
436