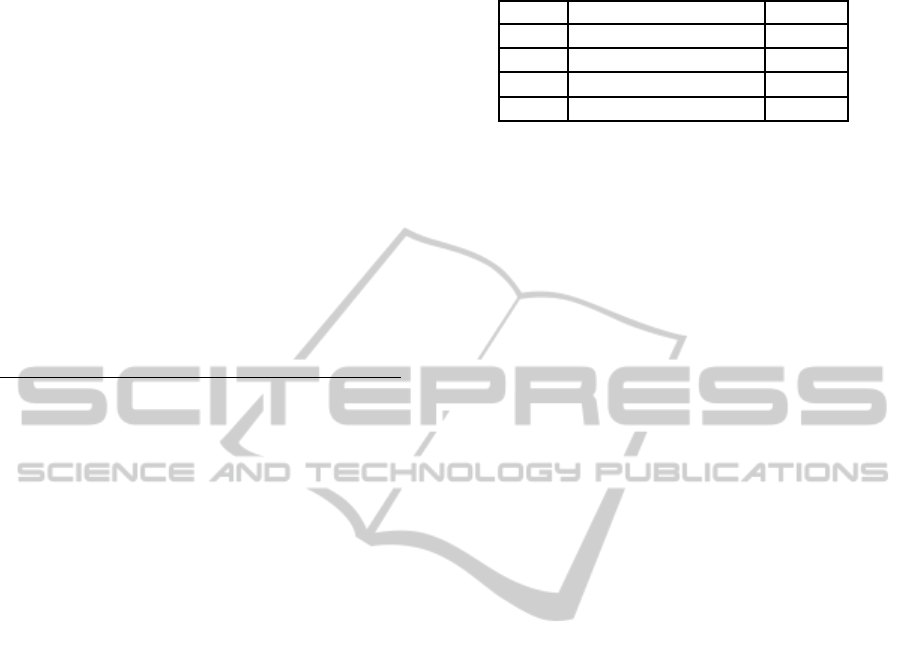
help of procedures verifying complicated models rel-
atively simple models that were discussed in previous
sections.
3.5 Verification
At the first stage null hypothesis about independence
of y
ph
on x
vd
was tested with the help previously dis-
cussed in (Senko and Kuznetsova, 2006) permutation
test version. Set of random permutations of integers
1, . . . , m was formed with the help of random num-
bers generator. This set
e
f
rng
consisted of N
g
elements.
Data sets {
e
S
p
( f
j
)| f
j
∈
e
f
rng
} was built from
e
S
0
by ran-
dom permutation of y
ph
positions relatively fixed po-
sitions of x
vd
. Statistical validity of null hypothesis is
evaluated with the help of p-value that is equal ratio
|{ f
j
∈
e
f
rng
|Q
min
[
e
S
p
( f
j
),
e
M
pwl
] < Q
min
(
e
S
0
,
e
M
pwl
)}|
N
g
.
In other words p-value is calculated as fraction of
random data sets where dependence of y
ph
on x
vd
is approximated better than at initial set
e
S
0
. Values
Q
min
(
e
S
0
,
e
M
pwl
) and Q
min
[
e
S
p
( f
j
),
e
M
pwl
] are calculated
with the help of procedure that is describe in section
3.3. Piecewise-linear modeling of y
ph
from x
vd
allows
to reject null hypothesis with p-value equal 0.000041.
Piecewise-linear modeling of y
lph
from x
vd
allows to
reject null hypothesis with p-value equal 0.000079.
At that number of random permutations was equal
10
6
. Then piecewise-linear regressions were veri-
fied relatively simple regression models. Optimal
piecewise-linear regression y
ph
= F
o
pwl
(x
vd
)+ε
pw
was
verified by testing null hypothesis about exhaustive
description of dependence by simple linear regression
y
ph
= α
0
+ α
1
x
vd
+ ε
1
. Piecewise-linear regression
y
lph
= F
o
pwl
x
vd
+ ε
pw
was verified by testing null hy-
pothesis about exhaustive description of dependence
by simple linear regression y
lph
= α
l
0
+ α
l
1
x
vd
+ ε
2
.
Two ways of regression coefficients α
0
, α
l
0
, α
1
, α
l
1
calculating were considered:
• simple regression coefficients were searched with
the help of standard LS procedure,
• such simple regression coefficients were chosen
that distance between verified piecewise-linear re-
gression and simple regression was minimal.
Let suppose that x values in
e
S
0
belong to some in-
terval (a
l
, a
h
). Then distance between two predicting
functions F
1
(x) and F
2
(x) is calculated by formula
D[F
1
(x), F
2
(x)] =
Z
a
h
a
l
[F
1
(x) − F
2
(x)]
2
dx.
Ratio (4) was used to estimate p-values. At that num-
ber of permutations was equal 10
6
. Results of verifi-
cation are represented in table.
Table 1: Results of verification.
target type of symple model p-value
y
ph
standard LS 0.022
y
lph
standard LS 0.026
y
ph
most close to F
o
pw
0.015
y
lph
most close to F
o
pw
0.0218
It is seen from table that p-values for null hypothe-
ses about exhaustive description of data by simple re-
gressions do not exceed 0.026. This result is strong
argument that simple regressions are not sufficient to
explain data and more complicated piecewise-linear
regression models are really necessary. Thus suppo-
sition that vitD correlates with PTH only when vitD
concentration is less than certain threshold level is sta-
tistically valid.
4 CONCLUSIONS
So method was proposed that allows to evaluate valid-
ity of choice between simple or complicated regres-
sion models in terms of p-values. Method is based on
testing null hypothesis about independence of devia-
tions from simple predicting function on X variables.
Method was successfully used for evaluating correct-
ness of biomedical supposition that vitamin D status
correlates with parathyroid hormone levels. Method
may be used in variety of tasks where a problem of
choice between more complicated or simple models.
REFERENCES
Akaike, H. (1974). A new look at the statistical model iden-
tification. IEEE Transactions on Automatic Control,
vol.19, iss.6,:pp. 716–723.
Domingos, P. (1999). The role of occam’s razor in knowl-
edge discovery. Data Mining and Knowledge Discov-
ery, vol. 3, iss. 4:pp. 409–425.
Ernst, M. (2004). Permutation methods: A basis for exact
inference. Statistical Science, 19(4):676–685.
Golland, P. et al. (2000). Permutation test for classification.
Journal of Machine Learning Research, 1.
Good, P. (2005). Permutation, Parametric and Bootstrap
Tests of Hypotheses. Springer Science+Business Me-
dia, Inc.
Hannan, E. and Quinn, B. (1979). The determination of the
order of an autoregression. Journal of the Royal Sta-
tistical Society, Series B (Methodological), vol.41:pp.
190–195.
Kim, G. et al. (2012). Relationship between vitamin d,
parathyroid hormone, and bone mineral density in el-
derly koreans. J Korean Med Sci.
KDIR2014-InternationalConferenceonKnowledgeDiscoveryandInformationRetrieval
442