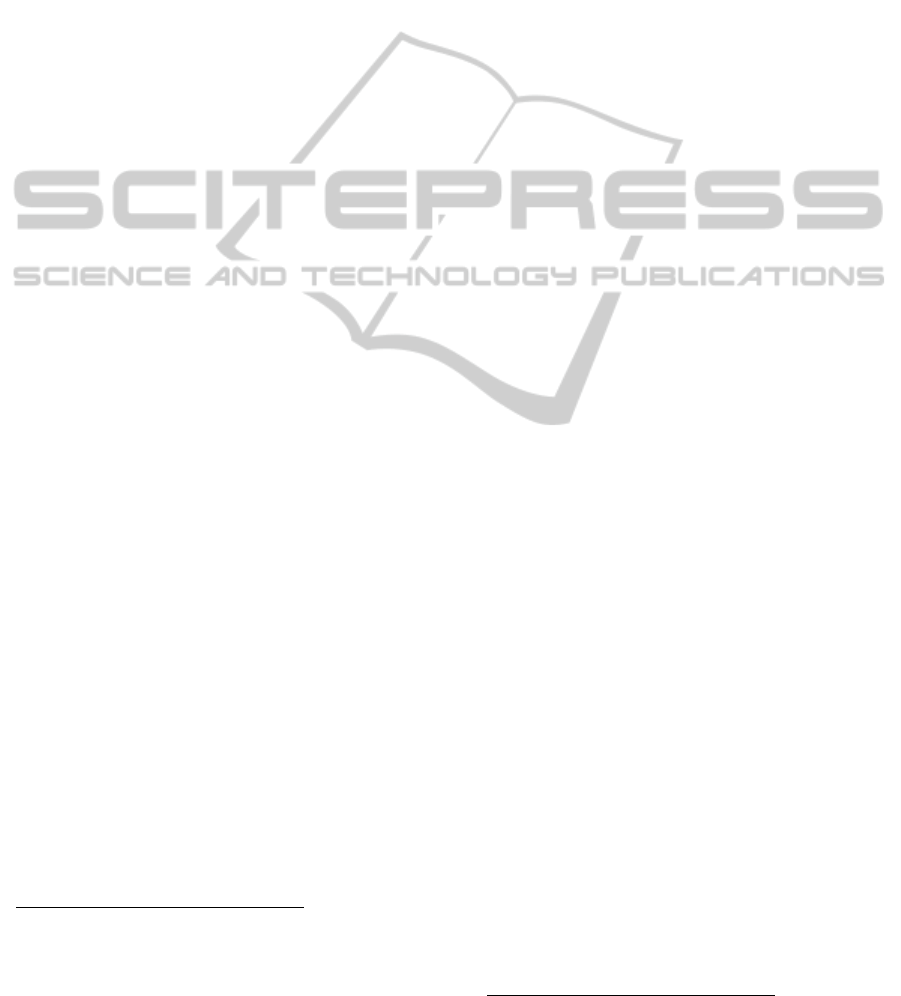
text-based construction (Upadhyay et al., 2014).
This pool can be structured by fixing the number of
retrieved images for each meaning according to their
relatedness score to the main topic (Hoque et al.,
2012) and by determining the similarity of a given
image to its neighbours. Obtained images are
organized founded on their visual similarity on a 2D
virtual canvas. As for the re-ranking step, the user
can use a concept hierarchy to focus and filter the
search results, or perform visual filtering through
pan and zoom operations (Hoque et al., 2013).
Google
1
Image search engine ameliorates the
query understanding by performing synonyms and
searching in the title and the surrounding text
(MacKinlay, 2005) in order to cover the majority of
the meanings and provide textual suggestions, but it
focus on the most well known meaning in ranked
images and gives similar images (Chechik et al.,
2010). Picsearch
2
offers also textual suggestions to
the user to boost the right understanding of the
ambiguous query and provides both simple and deep
search options, with the possibility of limiting the
search by colour, or to one of seven different
gradations of size.
Bing
3
, the search engine of Microsoft, refines the
result with five filters based on image size, aspect
ratio, colour or black and white, photo or illustration,
and facial features recognition. As well, Exalead
4
Images search engine presents a variety of filters. In
fact, it supplies the ability to specify the type of
images you wish. Details are also about the size, the
format (jpeg, gif or png), the orientation (landscape
or portrait), the dominant colour and the content
(image contains face) of the image. For ambiguous
query, the two last details can improve the
understanding of the user intent. Nevertheless, the
face filter becomes not efficient if there are many
famous persons who refer to the same query.
The Incogna
5
search engine tries from the
beginning of the search to cover all the sub-topics.
Therefore, unlike the major search engines, the more
you put keywords in your query, the more the result
is relevant. Then, if the user chooses an image
describing his intent, Incogna will provide similar
images thanks to its large-scale searchable visual
index built by processing the shape in every image.
1
http://images.google.com
2
http://fr.picsearch.com/
3
https://www.bing.com
4
http://www.exalead.com/search/image/
5
http://www.incogna.com/#random
Finally, having a large number of high quality
images, Flickr
6
, a very large photo-sharing site,
enhances the Yahoo image retrieval process.
However, as for scientific images search, Flickr may
not be an ideal source and its insertion risks
obscuring adequate images. Unfortunately, it often
discards the diversity factor and considers only one
meaning.
Hence, the image search engines do not always
guess the right meaning and follow the user intent.
Even if they did, the ranked images would belong
always to only one or two meanings. In fact, a text
has not always expressed exactly the content of an
image especially when the query is specified with
few terms or the method of the query understanding
seems limited.
3 OUR PROPOSED TAD
APPROACH
3.1 Strategy
We propose the TAD approach for image retrieval
system. The TAD approach is a puzzle constituted
by three main components which are the TAWQU
(Thesaurus-Based Ambiguous Web Query
Understanding) process, the ASC (Adaptive
Semantic Construction) process and the DR
(Diversity-based Retrieval) process.
The principal input is a textual query. According
to the standard image retrieval process announced
previously in the second section, the process must
include a query formulation step, but we ignore it as
we have only one term and there is no need to filter
the query by eliminating the senseless words.
Therefore, we turn straight to the query
interpretation by executing the TAWQU process in
order to extract different meanings for a given query.
The obtaining of these weighted subtopics launches
the ASC process, where new concepts are added to
construct our adaptive semantic. Then, based on
extracted weighted subtopics, we return diverse
images and textual and visual suggestions for users.
The Figure 1 describes our strategy, which
ensures the ability of covering the majority of the
possible meanings for a given ambiguous query by
providing diverse resulting images.
6
https://www.flickr.com/explore
AdaptiveSemanticConstructionforDiversity-basedImageRetrieval
445