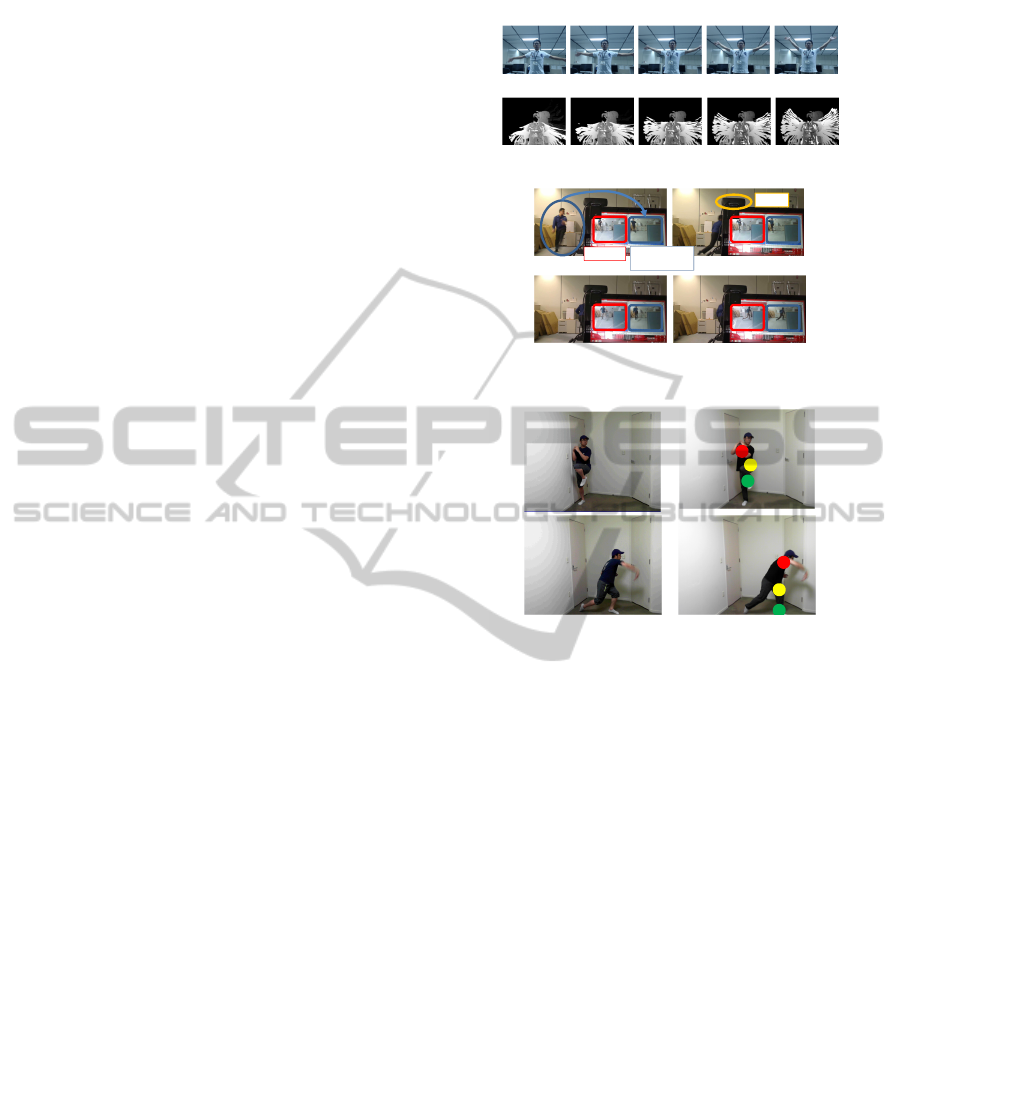
2001), and is employed in the proposed system. Fig-
ure 5 shows an MHI and snapshots of the correspond-
ing image sequence, where the snapshots are shown
from left to right in time order. In the MHI, the value
of each pixel shows how recently a motion was de-
tected on the pixel. Bright (white) pixels denote pixels
at which motions are detected. As the time proceeds
from the most recent motion, the pixels turn dark.
3 RESULTS
Currently, we have not yet verified the motor learn-
ing efficacy obtained with the proposed method. This
section only shows the appropriate motion synchro-
nization and body part estimation it provides.
First, we verified the system provides realtime and
automatic motion synchronization. Fig.6 shows an
example of the results obtained in the verification pro-
cedure. As shown in Fig.6, the trainee’s movement in
front of camera is correctly synchronized with the ref-
erence movement on the display within one second by
using a tablet PC.
We also verified the accuracy with which the sys-
tem estimates body parts for displaying sensor data.
The result sequences are shown in Fig.7. The red, yel-
low and green dots denote arm thigh, and toe, respec-
tively; the left row denotes reference and the right one
denotes synchronized practice sequences. As shown
in Fig.7, the proposed system works well for different
types of clothing worn by trainees. A few errors were
found to have occurred in the body parts estimation,
but the accuracy is good enough for showing sensor
data. So far, we sensors’ output didn’t be used, but
they can be assigned to size and/or color of dot for
intuitive feedback.
4 SUMMARY
In this paper we proposed a new visual feedback
method with the aim of providing visual feedback of
trainee’s movements for effective motor learning. The
method incorporates three main features: (1) auto-
matic temporal synchronization of trainer and trainee
motions, (2) intuitive presentation of sensor data, e.g.
surface electromyography (EMG) and cardiac rate,
based on spatial position of a sensor attached to the
user, and (3) an absence of restrictions on cloth-
ing worn by the user and on illumination conditions.
Future work will include verifying the actual motor
learning obtained with the proposed method.
Original image sequence
MHI sequence
Figure 5: Motion feature MHI.
Reference
Practice
in front of camera
Trainee’s motion
with 1 sec delay
Camera
Figure 6: Realtime processing on tablet PC is verified.
Figure 7: Body parts estimation; red dots denote arm, yel-
low dots denotes thigh, and green dots denotes toe.
REFERENCES
Bobick, A. and Davis, J. (2001). The representation and
recognition of action using temporal templates. IEEE
Trans. PAMI, 23(3).
Choi, W., Mukaida, S., Sekiguchi, H., and Hachimura, K.
(2008). Qunatitative analysis of iaido proficiency by
using motion data. In ICPR.
Chua, P., Crivella, R., Daly, B., Hu, N., Schaaf, R., Ven-
tura, D., Camil, T., Hodgins, J., and Paush, R. (2003).
Training for physical tasks in virtual environments:
Tai chi. In IEEE VR.
Effenberg, A., Fehse, U., and Weber, A. (2011). Movement
sonification: Audiovisual benefits on motor learning.
In The International Conference SKILLS.
Guadagnoli, M., Holcomb, W., and Davis, M. (2002). The
efficacy of video feedback for learning the golf swing.
Journal of Sports Science, 20:615–622.
Wieringen, P. V., Emmen, H., Bootsma, R., Hoogesteger,
M., and Whiting, H. (1989). The effect of videofeed-
back on the learning of the tennis service by interme-
diate players. Journal of Sports Science, 7:156–162.