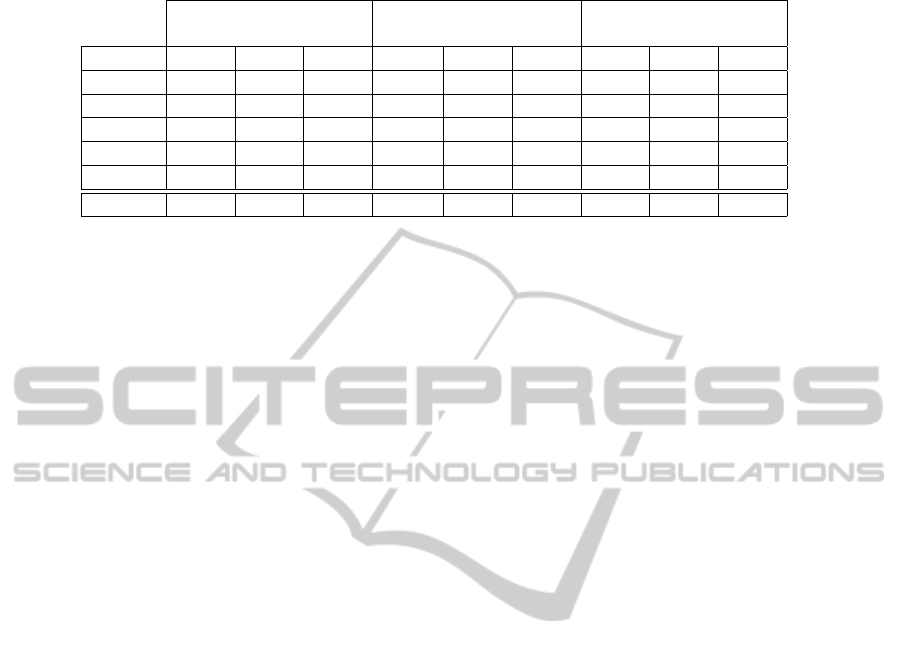
Table 1: Results of the 8-fold cross validation.
TRUE FALSE ACCURACY
POSITIVE RATE POSITIVE RATE RATE
USER NOF LAP CAR NOF LAP CAR NOF LAP CAR
A 62.50 69.44 66.67 41.67 22.22 19.44 60.42 73.61 73.61
B 77.08 89.58 93.75 60.08 43.75 43.75 58.33 72.92 75.00
C 80.56 77.78 66.67 44.44 26.39 26.39 68.06 75.69 70.14
D 70.31 59.38 51.56 48.44 26.56 21.88 60.94 66.41 64.84
E 48.21 46.43 58.93 30.36 53.57 53.57 58.93 46.43 52.68
MEAN 67.73 68.52 67.52 45.00 34.50 33.01 61.34 67.01 67.25
4 CONCLUSIONS AND FUTURE
WORKS
In this paper a system to detect the intention to start
walking has been presented. According to the results
obtained from five healthy users, the system has to
be improved to achieve better TPR and FPR. If the
system is able to predict each movement with a re-
ally low FP rate, the classifier output could serve as a
command to activate the engines of an exoskeleton or
a FES system to start walking. Therefore, in a rehabil-
itation process an exoskeleton could be used to sup-
port the lower limbs while the user carries out men-
tally walking intentions. The relationship between the
cognitive process to perform such movement and the
real movement could improve the rehabilitation due
to cerebral plasticity.
Therefore, other methods to characterize the EEG
signals before the movement onset will be studied.
For example, it is possible to calculate the power
spectral and to extract the best component of each
user to be used as feature. Moreover, a frequency fil-
ter narrower could be applied in order to isolate the
mu and beta frequency bands which are involved in
ERD phenomenon. Furthermore, ERD can be used
purely as theory indicates (a relative decrement of
power spectral in a special frequency band measured
in percentage). Usually, EEG signals have some arti-
facts like eye blinks or muscle activity that should be
removed. In particular, eye movements could be re-
moved from EEG signals recording the electroculag-
raphy (EOG) signals using 2 bipolar electrodes (hor-
izontal and vertical channels) and then reducing their
contribution through a linear regression which re-
lates EEG and EOG signals (Kenemans et al., 1991).
Moreover, artifacts due to neck movements can be
easily seen in EEG signals due to their magnitude, so
it is possible to add a voltage threshold to avoid it.
The population of this experiment and also the
number of sessions will be increased. Furthermore,
patients with complete and incomplete spinal cord in-
jury will perform the experiment in order to test the
system and to evaluate the performance. It is ex-
pected that some patients keep their brain procedures
related to the intention of movement as healthy people
although they could be weaker or allocated in other
brain area (Wei et al., 2011). Then, a real-time test
with a better system will be performed both healthy
as patient users.
ACKNOWLEDGEMENTS
This research has been funded by the Commission of
the European Union under the BioMot project - Smart
Wearable Robots with Bioinspired Sensory-Motor
Skills (Grant Agreement number IFP7-ICT- 2013-10-
611695), by the Spanish Ministry of Economy and
Competitiveness as part of the Brain2motion project
- Development of a Multimodal Brain-Neural Inter-
face to Control an Exoskeletal: Neuroprosthesis Hy-
brid Robotic System for the Upper Limb (DPI2011-
27022-C02-01), and by Conselleria d’Educaci
´
o, Cul-
tura i Esport of Generalitat Valenciana of Spain
through grant VALi+d ACIF/2012/135.
REFERENCES
Alhaddad, M. J. (2012). Common Average Reference
(CAR) Improves P300 Speller. International Journal
of Engineering and Technology, 2.
Bai, O., Rathi, V., Lin, P., Huang, D., Battapady, H., Fei,
D.-Y., Schneider, L., Houdayer, E., Chen, X., and
Hallett, M. (2011). Prediction of human voluntary
movement before it occurs. Clinical Neurophysiology,
122(2):364 – 372.
Guido Dornhege, Jos
´
e del R. Mill
´
an, T. H. D. J. M. and
Muller, K.-R. (2007). Towards Brain-Computer Inter-
facing. The MIT Press.
Kenemans, J. L., Molenaar, P. C., Verbaten, M. N., and
Slangen, J. L. (1991). Removal of the ocular artifact
PreliminaryStudytoDetectGaitInitiationIntentionThroughaBCISystem
65