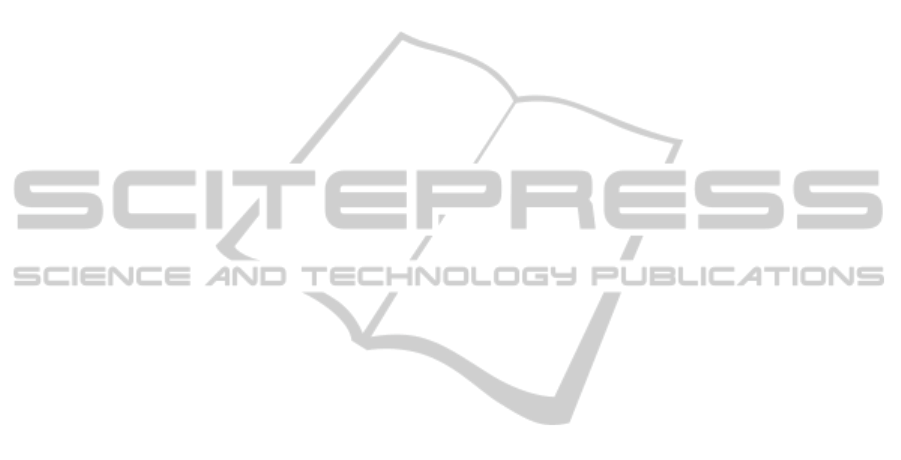
6 CONCLUSION AND FUTURE
WORK
In this paper, we proposed a representation of textual
data that improves classification of document meth-
ods by generalizing some features (words) to their
POS category when these words appear as less dis-
criminant for the task. Our results show that this ap-
proach, called GENDESC is appropriate when clas-
sification is at stake, regardless of the nature of its
criteria. We have also demonstrated that D, Discrim-
inence, is a measure that can be relevant to find se-
mantically important words in a corpus. In our future
work, we plan to use semantic information to improve
classification.
In previous work, we proved that n-grams can
be combined with GENDESC to slightly improve the
classification (Tisserant et al., 2013). HashTag can
probably be generated with n-grams of words with
high D value. So we plan to use these n-grams in
order to construct new Hashtags (e.g. kdir 2014 →
#kdir2014). They could be useful to detect Hash-
Tags which combine several concepts associated with
n-grams returned with GENDESC (i.e. n-grams of
words and/or Hashtags). As an example, a lot of
tweets contain both HashTag #Iran and word nuclear,
and they are often close to each other. The system
should detect that #IranNuclear could be an interest-
ing HashTag for all these tweets, which evoke the Ira-
nian nuclear issue. If enough people use the proposed
HashTag, they could follow news about ”Iranian nu-
clear” more easily.
REFERENCES
B
´
echet, N., Chauch
´
e, J., Prince, V., and Roche, M. (2014).
How to combine text-mining methods to validate in-
duced verb-object relations? Comput. Sci. Inf. Syst.,
11(1):133–155.
Chamberlain, J., Fort, K., Kruschwitz, U., Lafourcade, M.,
and Poesio, M. (2013). Using games to create lan-
guage resources: Successes and limitations of the ap-
proach. In The Peoples Web Meets NLP, pages 3–44.
Springer.
Conover, M., Gonc¸alves, B., Ratkiewicz, J., Flammini, A.,
and Menczer, F. (2011). Predicting the political align-
ment of twitter users. In Proceedings of 3rd IEEE
Conference on Social Computing (SocialCom).
Costa, J., Silva, C., Antunes, M., and Ribeiro, B. (2013).
Defining semantic meta-hashtags for twitter classifi-
cation. In Adaptive and Natural Computing Algo-
rithms, pages 226–235. Springer.
Faure, D. and Nedellec, C. (1999). Knowledge acquisition
of predicate argument structures from technical texts
using machine learning: The system asium. In In Pro-
ceedings of EKAW, pages 329–334.
Gamon, M. (2004). Sentiment classification on customer
feedback data: noisy data, large feature vectors, and
the role of linguistic analysis. In Proceedings of COL-
ING ’04.
Guyon, I. and Elisseeff, A. (2003). An introduction to vari-
able and feature selection. The Journal of Machine
Learning Research, 3:1157–1182.
Hall, M., Frank, E., Holmes, G., Pfahringer, B., Reute-
mann, P., and Witten, I. H. (2009). The weka data
mining software: an update. SIGKDD Explor. Newsl.,
11(1):10–18.
Hirano, T., Matsuo, Y., and Kikui, G. (2007). Detecting
semantic relations between named entities in text us-
ing contextual features. In Proceedings of the 45th
Annual Meeting of the ACL on Interactive Poster and
Demonstration Sessions, pages 157–160. Association
for Computational Linguistics.
Jones, K. S. (1972). A statistical interpretation of term
specificity and its application in retrieval. Journal of
Documentation, 28:11–21.
Joshi, M. and Penstein-Ros
´
e, C. (2009). Generalizing de-
pendency features for opinion mining. In Proceedings
of the ACL-IJCNLP 2009 Conference Short Papers,
pages 313–316.
Kywe, S. M., Hoang, T.-A., Lim, E.-P., and Zhu, F.
(2012). On recommending hashtags in twitter net-
works. In Proceedings of the 4th International Con-
ference on Social Informatics, SocInfo’12, pages 337–
350, Berlin, Heidelberg. Springer-Verlag.
Luhn, H. P. (1957). A statistical approach to mechanized
encoding and searching of literary information. IBM
J. Res. Dev., 1(4):309–317.
Mazzia, A. and Juett, J. (2011). Suggesting hashtags on
twitter. In EECS 545 Project, Winter Term, 2011. URL
http://www-personal.umich.edu/ amazzia/pubs/545-
final.pdf.
Ozdikis, O., Senkul, P., and Oguztuzun, H. (2012). Seman-
tic expansion of hashtags for enhanced event detec-
tion in twitter. In Proceedings of the 1st International
Workshop on Online Social Systems.
Porter, M. (1980). An algorithm for suffix stripping. Pro-
gram, 14(3):130–137.
Salton, G. and McGill, M. J. (1986). Introduction to Mod-
ern Information Retrieval. McGraw-Hill, Inc.
Sriram, B., Fuhry, D., Demir, E., Ferhatosmanoglu, H.,
and Demirbas, M. (2010a). Short text classification
in twitter to improve information filtering. In Pro-
ceedings of the 33rd international ACM SIGIR con-
ference on Research and development in information
retrieval, pages 841–842. ACM.
Sriram, B., Fuhry, D., Demir, E., Ferhatosmanoglu, H.,
and Demirbas, M. (2010b). Short text classification
in twitter to improve information filtering. In Pro-
ceedings of the 33rd international ACM SIGIR con-
ference on Research and development in information
retrieval, pages 841–842. ACM.
Tisserant, G., Roche, M., and Prince, V. (2013). Gendesc :
Vers une nouvelle reprsentation des donnes textuelles.
RNTI.
Witten, I. H. and Frank, E. (2005). Data Mining: Practi-
cal machine learning tools and techniques. Morgan
Kaufmann.
MiningTweetData-StatisticandSemanticInformationforPoliticalTweetClassification
529