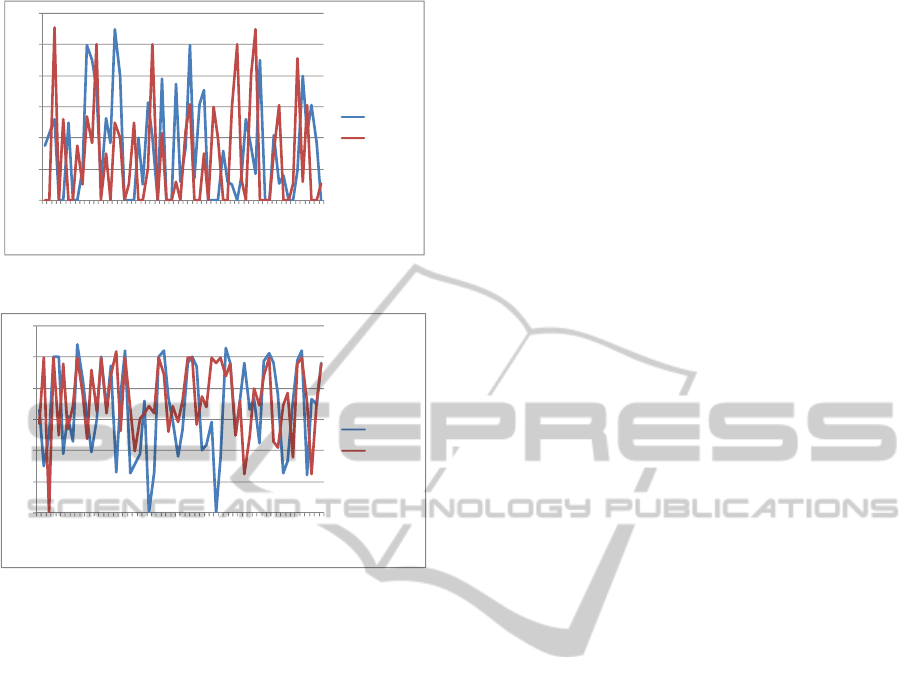
Figure 3: The experimental specificity result.
Figure 4: The experimental exhaustivity result.
Figure 4 show the experimental result of the
exhaustivity. The two curves displayed shows the
LR+ values by comparing our test outcome with to
the outcome of the two bases before mentioned
(wikipedia and imdb) for 60 films. We obtain a high
degree of exhaustivity (the most values are between
10 and 25).
When we examine these measures calculated on
the evaluation corpus, we note that the experimental
results are interesting) by comparing the correct
description rate of our test outcome (~83%) with the
correct description rate to the work of (Stanislas, O.
et al., 2010) (80%).
5 CONCLUSIONS
This paper presents an automatic audiovisual
documents genre description. The objective of this
approach is to overcome the semantic analysis gap
in order to extract the audiovisual genre. In this
context, we use the pre-production documents to
combine the statistical analysis and the semantic
analysis. Our statistical analysis is based on the TF-
IDF. We propose adapting this metric to the
semantic lexical database WordNet. In addition, we
exploit four semantic similarity measures to estimate
the proximity between terms and genres. In our
future work, we plan to explore the structuring and
the homogenization of the descriptions by XML
descriptors (metadata) integrated in the MPEG7
standard.
REFERENCES
Brezealed, B and Cook, D. 2006. Using closed captions
and visual features to classify movies by genre. In
Proceedings of Multimedia Data Mining/Knowledge
Discovery and Data Mining. MDM '07, New York,
NY, USA, Article No. 4.
Brett, K., Geoffrey, N., Hinrich, S. 1997. “Automatic
Detection of Text Genre”. ACL’97, Madrid, pages 32-
38.
Chernoff, H., 1954. On the distribution of the likelihood
ratio. Ann. Math. Stat. 25:573–578. Journal,Volume
25, Number 3 (1954), 573-578.
C. Van Rijsbergen. 1979. Information Retrieval (Book 2nd
ed). Butterworth-Heinemann Newton, MA, USA, 1979.
E.Stamatatos, N.Fakotakis, G. Kokkinakis, “Text Genre
Detection Using Common Word Frequencies”, In
Proc. of the 18th Int. Conf. on Computational
Linguistics (COLING2000) Saarbrücken, Germany,
pp. 808-814, 2000
Hyoyoung, K., Jin, Wan Park. , Genre Visualization Based
on Words Used in Text. International Conference,
HCI International 2013, Las Vegas, NV, USA, July,
2013, Proceedings, Part II .pp 551-554.
Internet Movie Database, Available at: http://www.imdb.
com/ last visit:18/07/2014.
Jaccard p. 1901.Etude comparative de la distribution
florale dans une portion des alpes et des jura. Bulletin
de la société vaudoise des sciences naturelles, vol 37,
pp 547-5790.
Karlgren, J and Douglass,C. 1994. Recognizing text
genres with simple metrics using discriminant
analysis. In Proceedings of Coling 94, Kyoto, Japan,
pp 1071-1075.
Karlgren, J., Ivan, B., Johan, D., Anders, H., Niklas, W.,
Iterative Information Retrieval Using Fast Clustering
and Usage-Specific Genres”, 8th DELOS workshop on
User Interfaces in Digital Libraries, Stockholm,
Sweden, 21-23 October 1998. Pages 85-92.
Lin Wei-Hao and Alexander Hauptmann. 2002. News
video classification using SVM-based multimodal
classifiers and combination strategies. In Proceedings
of the tenth ACM international conference on
Multimedia. ACM, New York, NY, USA, 323-326.
LR Lawlor, Overlap, similarity, and competition
coefficients. Journal, Vol. 61, No. 2, Apr., 1980.Pages
245-251.
Marc, N., Jocelyne N., Peter, R. King and Ludovic, G.
2007.Genre Driven Multimedia Document Production
by means of Incremental Transformation. In
proceedings of the 2007 ACM symposium on
0
0,2
0,4
0,6
0,8
1
1,2
film1
film5
film9
film13
film17
film21
film25
film29
film33
film37
film41
film45
film49
film53
film57
imdb
wikipedia
0
5
10
15
20
25
30
film1
film5
film9
film13
film17
film21
film25
film29
film33
film37
film41
film45
film49
film53
film57
imdb
wikipedia
KDIR2014-InternationalConferenceonKnowledgeDiscoveryandInformationRetrieval
542