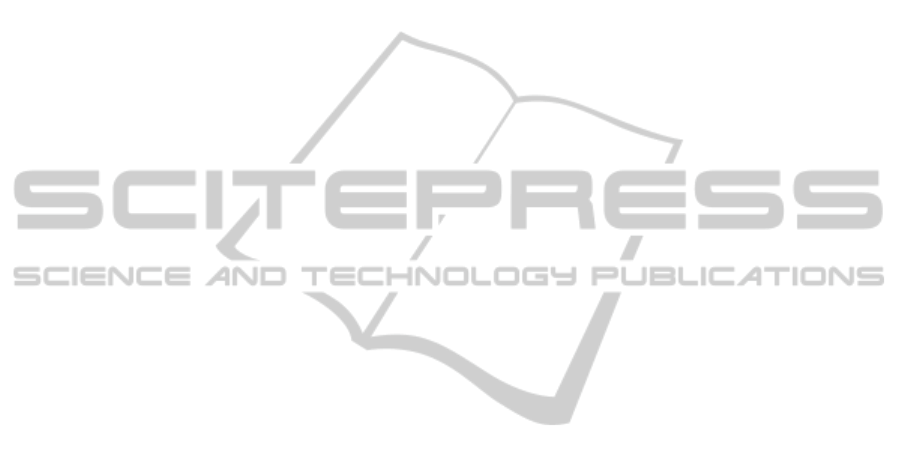
Features pointed out in the literature was considered
the gold pattern and F-score calculus was performed
for each approach. Figure 2 presents a comparative
graph.
F-score shows how much the selection of
features by an approach is close to literature. In
other words, how much a feature selection method is
according to human expert and semantic election.
The choosing of some approaches in specifics
data sets is very meaningless, according literature.
For example, this occurs on CFS with Breast Cancer
and on RSAR with Lymphograpy, because there is
no point for them.
For Central Nervous System data set, only the
proposal of this work, named MSSAR, had points.
MSSAR had points in all tested data sets. More
details concerning the tests are available at https://
drive.google.com/folderview?id=0B1CL3P-wkasBT
VBCZFB0UDFadncandusp=sharing.
REFERENCES
Almuallim, H.; Dietterich T. G. 1991. Learning with
Many Irrelevant Features. In: Proceedings of the 9th
National Conference on Artificial Intelligence,
Anaheim, CA, v. 2, pp. 547–552.
Almuallim, H.; Dietterich, T. G. 1992. Efficient algorithms
for identifying relevant features. In: Proceedings of the
Ninth Canadian Conference on Artificial Intelligence,
Vancouver, BC: Morgan Kaufmann. May 11-15, pp.
38-45.
Ammu, P. K.; Preeja, V. 2013. Review on Feature
Selection Techniques of DNA Microarray Data. In:
International Journal of Computer Applications 0975
– 8887 Volume 61– No.12, January 2013. pp. 39-44.
Bray, F.; Ren, J. S.; Masuyer, E.; Ferlay, J. Estimates of
global Cancer prevalence for 27 Sites in the Adult
Population in 2008. Int J Cancer. 2013 Mar 1; 132
(5):1133-45. doi:10.1002/ijc.27711. Epub 2012 Jul 26.
Chahkandi,Vahid; Yaghoobi, Mahdi; Veisi, Gelareh. 2013.
Feature Selection with Chaotic Hybrid Artificial Bee
ColonyAlgorithm based on Fuzzy CHABCF In:
Journal of Soft Computing and Applications. pp. 1-8
Chouchoulas, A.; Shen, Q. 2001. Rough set-aided keyword
reduction for text categorization. Applied Artificial
Intelligence: An International Journal. 159:843-873.
Cover, T. M.; Thomas, J. A. 1991. Elements of Information
Theory. Copyright © 1991 John Wiley and Sons, Inc.
Print ISBN 0-471-06259-6 Online ISBN 0-471-20061-
1. 563 p.
Dash, M.; Liu, H. 2003. Consistency-Based Search in
Feature Selection. Artificial Intelligence. 1511-2:155-
176, December, 2003.
Deisy, C., Baskar, S., Ramraj, N., Saravanan Koori, J., and
Jeevanandam, P. 2010.. A novel information theoretic-
interact algorithm (IT-IN) for feature selection using
three machine learning algorithms. Expert Systems
with Applications, 37(12), 7589-7597. Elsevier Ltd.
doi:10.1016/j.eswa.2010.04.084
Euzenat, Jérôme and Shvaiko, Pavel. 2007. Ontology
matching, Springer-Verlag, 978-3-540-49611-3.
Fellbaum, Christiane. 1998. WordNet: An Electronic
Lexical Database. Cambridge, MA: MIT Press.
Ferlay, J.; Soerjomataram, I.; Ervik, M. R.; Dikshit, S.;
Eser, C.; Mathers, M.; Rebelo, M.; Parkin, D.;
Forman, D.; Bray, F. GLOBOCAN 2012 v1.0, Cancer
Incidence and Mortality Worldwide: IARC Cancer
Base No. 11 [Internet]. Lyon, France: Inter-national
Agency for Research on Cancer; 2013. Available at:
http://globocan.iarc.fr, Accessed on June 2014.
Freitas, A. A. 2001. Understanding the Crucial Role of
Attribute Interaction in Data Mining. Artificial
Intelligence Review, (1991), 177-199.
Hall, M. A. 2000. A correlation-based feature selection for
discrete and numeric class machine learning.
ICML'00. In: Proceedings of the 17th International
Conference on Machine Learning. pp. 1157-1182.
He, X.; Cai, D.; Niyogi, P. 2005. Laplacian score for
feature selection. In: Y. Weiss, B. Scholkopf, and J.
Platt, editors, Advances in Neural Information
Processing Systems 18, Cambridge, MA, MIT Press.
Hein, N.; Kroenke, A. 2010 Escólios sobre a Teoria dos
Conjuntos Aproximados – Commentaries about the
Rough Sets Theory. In: Revista CIATEC – UPF, vol.2
1, pp. 13-20. doi: 10.5335/ciatec.v2i1.876 13.
Inbarani, H. H.; Thangavel, K.; Pethalakshmi, A. 2007.
Rough Set Based Feature Selection for Web Usage
Mining. In: International Conf. on Computational
Intelligence and Multimedia Applications. ICCIMA
2007, pp. 33-38. IEEE. doi:10.1109/ICCIMA.2007.356
Jaro, M. A. 1989. Advances in record linkage methodology
as applied to the 1985 census of Tampa Florida.
Journal of the American Statistical Association 84
(406): 414–20. doi:10.1080/01621459.1989.10478785.
Jaro, M. A. (1995). Probabilistic linkage of large public
health data file. Statistics in Medicine. 14 (5–7): 491–
8. doi:10.1002/sim.4780140510. PMID 7792443.
Jemal, A.; Bray, F.; Center, M.; Ferlay, J.; Ward, E.;
Forman., D. 2011. Global Cancer statistics. CA
Cancer Journal for Clinicians.; 61(2):69–90.
Kira, K.; Rendell, L. A. 1992. The Feature Selection
Problem: Traditional Methods and a New Algorithm,
In: Proceedings of 10th Conference on Artificial
Intelligence, Menlo Park, CA, pp. 129-136.
Kira, K.; Rendell, L. A.1992. A practical approach to
feature selection. In: Sleeman and P. Edwards,editors,
Proceedings of the 9th International Conference on
Machine Learning ICML-92, Morgan Kaufmann, pp.
249-256.
Kononenko, I. 1994. Estimating attributes: Analysis and
extension of RELIEFF. In: F. Bergadano and L. de
Raedt, editors, In: Proceedings of the European
Conference on Machine Learning, April 6-8, Catania,
Italy, Berlin: Springer-Verlag, pp. 171-182.
Kuo, Y-T.; Lonie, A.; Sonenberg, L. Domain Ontology
Driven Data Mining: A Medical Case Study.
Proceddings of 2007 ACM SIGKDD Workshop on
SemanticEnrichmentofRelevantFeatureSelectionMethodsforDataMininginOncology
29