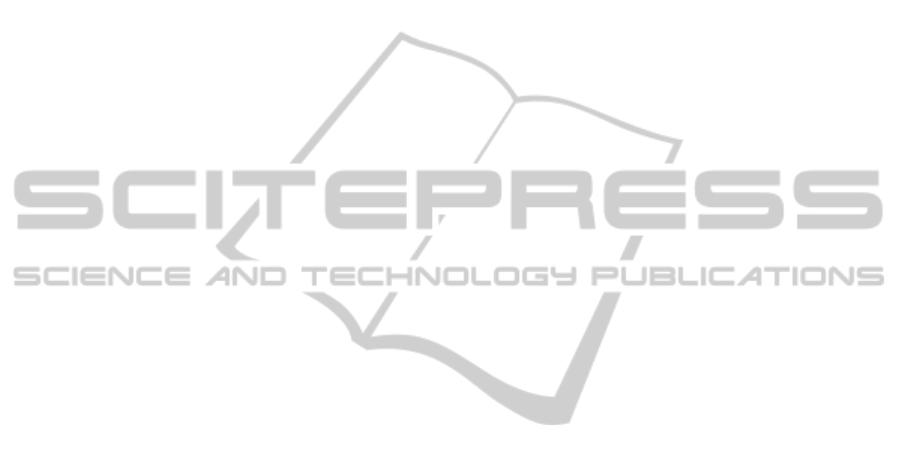
3 MULTIPLE CONTEXT-
DEPENDENT SOCIAL
NETWORKS
Since the social networks approaches typically
employed in research were not designed to provide
practical performance analysis insights for soccer
practitioners, in the second study we developed and
validated a multiple context-dependent social
networks method for applied performance analysis.
We used OPTA passing distribution raw data
from 32 teams obtained from 16 English Premier
League matches. Beyond the team global network
for the entire match we developed a set of specific
social networks capturing different game contexts:
goalkeeper’s distribution, defenders’ distribution,
midfielders’ distribution, forwards’ distribution,
shooting opportunities path analysis, 1st phase area
distribution, 2nd phase area distribution and creation
and finishing area distribution. Topological graph
visualizations and three individual players’ metrics
(betweenness, closeness and eigenvector) were
obtained from each specific network to objectively
quantify the individual influences in team
performance. This methodology was developed
under the advice of a panel of 5 experts, who assured
its face validity. Feedbacks from the experts were
integrated during the method developmental stage.
In a second step we determined the Content Validity
Ratio (CVR) (Lawshe, 1975) of a panel of 8 subject-
matter experts, who were asked to indicate whether
each context-dependent network is “essential” to
capture the essence of each corresponding game
context.
The CVR data of every context-dependent
network was superior to 0.5, which ensured the
content validity of the methodology. In a third step,
we tested the sensibility of the methodology
comparing the mean and variance of players’ metrics
in each match between the different context-
dependent networks, using repeated measures
ANOVA. The significant differences we found, with
large effect sizes attributed to eigenvector (ɳ
2
=.570)
and betweenness (ɳ
2
=.590) measures, suggested the
multiple context-dependent networks are sensitive
enough to capture differences in the way players
interact with each other in different game contexts.
4 CONCLUSIONS
In sum, social networks seem to be an appropriate
synthetic method to deal with large data sets
quantifying the number of connections between
team players. Here, we used passing data as
informational variables linking the network nodes.
However, future research should explore the
usefulness of positional tracked data from multiple
players’ movement trajectories to assess, for
instance, the positional stability of teams.
ACKNOWLEDGEMENTS
The authors wish to acknowledge OPTA statistics by
providing the data sets used in these studies.
REFERENCES
Cotta, C. Mora, A. M. Merelo, J. J. And Meelo-Molina, C.
(2013) A network analysis of the 2010 fifa world cup
champion team play. Journal of System Science and
Complexity. 26. p. 21–42.
Duch, J. Waitzman, J. S. And Amaral, L. A. N. (2010)
Quantifying the performance of individual players in a
team activity, PLoS ONE. 5(6). p. e10937.
Grund, T. (2012) Network structure and team
performance: The case of English Premier League
soccer teams. Social Networks. 34(4). p. 682–690.
Lames, M. And Mcgarry, T. (2007) On the search for
reliable performance indicators in game sports.
International Journal of Performance Analysis in
Sport. 7(1). p. 1-18.
Lawshe, C. H. (1975) A Quantitative Approach To
Content Validity. Personnel Psychology. 28(4). p.
563-575.
Travassos, B. Davids, K. Araújo, D. And Esteves, P. T.
(2013) Performance analysis in team sports: Advances
from an Ecological Dynamics approach. International
Journal of Performance Analysis in Sport. 13(1). p.
83-95.