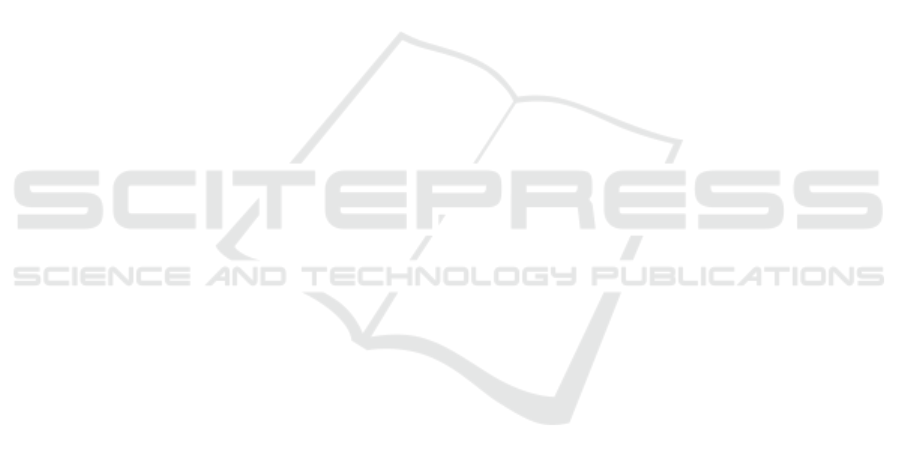
4 ENVIRONMENTAL ANALYSIS
Figure 5 shows the classes which have the most
change rate and magnitude of their change in terms of
pixels. As the statistics show from 1998 to 2010 the
number of pixels which converted from planted
forest, semi-dense forest, dense forest, and dense
grassland classes to road, residential, low density
grassland, irrigated agriculture, plantations,
shrubbery and non-dense forest classes is very high
and this represents a serious degradation in this area.
Road construction in forests regardless of its negative
effects on the forest, inappropriate urban
development, human progression in nature, cutting
trees for fuel, human farming in the forests to provide
food supply, and etc. are some main reasons for
degradation in this area.
A similar research was developed and
demonstrated by Fraser using six national parks in
Canada. It covered a range of geographical and
ecological conditions and was subject to a variety of
change agents including forest harvesting, wildfire,
land use development, and climate/weather (Fraser,
Olthof and Pouliot, 2009). In contrast to Golestan
National Park area that is located on one Landsat
scene and there is no need to mosaic Landsat images,
the area of Fraser’s study was vast and required more
than one Landsat frame to provide complete
coverage. They used 30m resolution Landsat EM and
ETM+, from 1990 to 2005 to generate baseline land
cover classification at five years intervals. Due to
huge height difference, removing haze and
topographic effects for Canada’s national parks was
necessary. However in Golestan National Park,
topographical elevations are fairly smooth and there
was no need to apply topological corrections in pre-
processing. Moreover, radiometric normalization in
Canada national parks was done by using filtering,
while for Golestan National Park it was done by
histogram matching. In both methods, identifying the
changed pixels and labelling them, were determined
using CVA and signature extension. Finally in
Canada baseline land cover and changes were
validated by updated available maps and in Golestan
National Park by high-resolution Geoeye images (due
to the lack of updated maps). Fraser reported 92%
correctly identified changed pixels and 8% omission
error rate in Canada’s parks.
5 CONCLUSION
Timely and accurate change detection of Earth’s
surface features is extremely important for
understanding relationships and interactions between
human and natural phenomena in order to promote
better decision making. Remote sensing data are
primary sources extensively used for change
detection in recent decades and many change
detection techniques have been developed based on
them. The common goal of all these algorithms is to
improve the accuracy of the information extracted
from remote sensing images. In this paper, a change
detection method was proposed to determine changes
in the forests of Northern Iran (Golestan National
Park). Using the combination of spectral and
classification methods lead to an acceptable accuracy.
In comparison with the conducted research on
national parks of Canada, lack of updated reference
maps, has a direct impact on the final accuracy. The
results of the assessment indicated that change
detection method should be developed based on local
knowledge. While this method provides a set of
generic procedures and tools for change detection, its
successful application requires an analyst
experienced in land cover interpretation and image
processing. In particular, the baseline land cover
labeling, assessing results from the image correction
methods, determining a CVA change threshold, and
development of signature extension rules, are
subjective and will determine the final accuracy of the
land cover change products. This algorithm is a cost-
effective change detection method in large areas and
tries to minimize the role of the human operator. It
can be implemented for most forests regardless of
their vegetation. This study is intended to explorer use
of high resolution images in the future in order to
investigate its capabilities to determine the change of
plant species. In future this method also can be
elevated using optimisation methods to find the best
values for CVA thresholds, number of clusters, and
similarity measure and result in an extended
intelligent version of current change detection
method.
REFERENCES
Chen, J., Gong, P., He, C., Shi, R. & P. (2003). Land-
Use/Land-Cover Change Detection Using Improved
Change-Vector Analysis.
Chen, X., Chen, J. (2012). An automated approach for
updating land cover maps based on integrated change
Third International Conference on Telecommunications and Remote Sensing