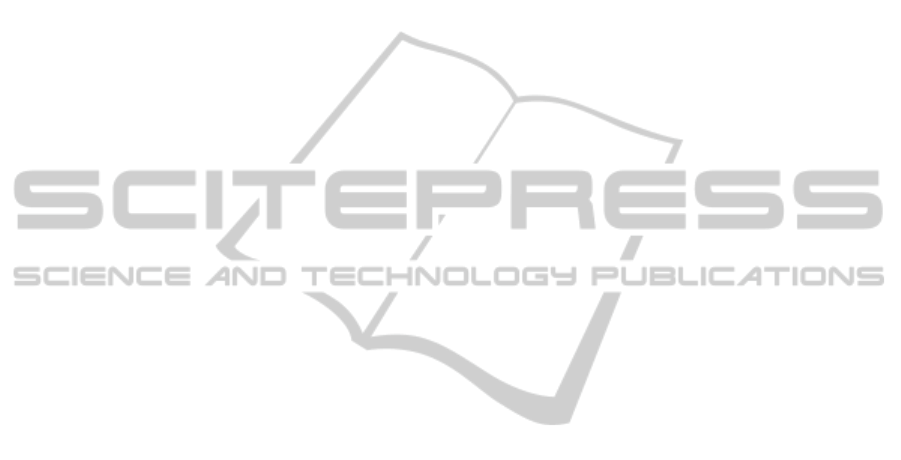
for a nonlinear model, the form of the model must be
specified, the parameters need to be estimated, and
starting values for those parameters must be carefully
provided.(Box and Tidwell, 1962) proposed the first
technique for model selection. (Royston and Sauer-
brei, 2008) suggested a class of regression models by
fractional polynomials (FP), involving model choice
from a specific number of models, but all of these
methods work only in case of complete data. We will
try to modify one of these approaches and apply it on
missing data. To further improve and test the perfor-
mance of the proposed method, we will try and incor-
porate good ideas of other methods for example those
presented in (Luengo et al., 2012)also carry out com-
parative studies on real data sets, readers are referred
to (Alcal
´
a et al., 2010) for good sample data sets.
REFERENCES
Alcal
´
a, J., Fern
´
andez, A., Luengo, J., Derrac, J., Garc
´
ıa,
S., S
´
anchez, L., and Herrera, F. (2010). Keel data-
mining software tool: Data set repository, integration
of algorithms and experimental analysis framework.
Journal of Multiple-Valued Logic and Soft Computing,
17:255–287.
Allison, P. D. (2002). Missing data: Quantitative applica-
tions in the social sciences. British Journal of Mathe-
matical and Statistical Psychology, 55(1):193–196.
Azar, B. (2002). Finding a solution for missing data. Mon-
itor on Psychology, 33(7):70–1.
Baraldi, A. N. and Enders, C. K. (2010). An introduction
to modern missing data analyses. Journal of School
Psychology, 48(1):5–37.
Box, G. E. and Tidwell, P. W. (1962). Transformation of the
independent variables. Technometrics, 4(4):531–550.
Dempster, A. P., Laird, N. M., and Rubin, D. B. (1977).
Maximum likelihood from incomplete data via the em
algorithm. Journal of the Royal Statistical Society. Se-
ries B (Methodological), pages 1–38.
Draper, N. R. and Smith, H. (1981). Applied regression
analysis 2nd ed.
Enders, C. K. (2006). A primer on the use of modern
missing-data methods in psychosomatic medicine re-
search. Psychosomatic medicine, 68(3):427–436.
Enders, C. K. (2010). Applied missing data analysis. Guil-
ford Press.
Enders, C. K. and Bandalos, D. L. (2001). The relative per-
formance of full information maximum likelihood es-
timation for missing data in structural equation mod-
els. Structural Equation Modeling, 8(3):430–457.
Graham, J. W. (2009). Missing data analysis: Making it
work in the real world. Annual review of psychology,
60:549–576.
Little, R. J. and Rubin, D. B. (2002). Statistical analysis
with missing data.
Luengo, J., Garc
´
ıa, S., and Herrera, F. (2012). On the
choice of the best imputation methods for missing val-
ues considering three groups of classification meth-
ods. Knowledge and information systems, 32(1):77–
108.
Montez-Rath, M. E., Winkelmayer, W. C., and Desai, M.
(2014). Addressing missing data in clinical studies
of kidney diseases. Clinical Journal of the American
Society of Nephrology, pages CJN–10141013.
Montgomery, D., Peck, E., and Vining, G. (2006). Diagnos-
tics for leverage and influence: Introduction to linear
regression analysis . hoboken.
Ng, S. K., Krishnan, T., and McLachlan, G. J. (2012). The
em algorithm. In Handbook of computational statis-
tics, pages 139–172. Springer.
Royston, P. and Sauerbrei, W. (2008). Multivariable model-
building: a pragmatic approach to regression anaylsis
based on fractional polynomials for modelling contin-
uous variables, volume 777. John Wiley & Sons.
Schafer, J. L. (2010). Analysis of incomplete multivariate
data. CRC press.
Schafer, J. L. and Graham, J. W. (2002). Missing data: our
view of the state of the art. Psychological methods,
7(2):147.
Schlomer, G. L., Bauman, S., and Card, N. A. (2010). Best
practices for missing data management in counsel-
ing psychology. Journal of Counseling Psychology,
57(1):1.
Seaman, S. R. and White, I. R. (2013). Review of inverse
probability weighting for dealing with missing data.
Statistical Methods in Medical Research, 22(3):278–
295.
Smyth, G. K. (2002). Nonlinear regression. Encyclopedia
of environmetrics.
ICPRAM2015-InternationalConferenceonPatternRecognitionApplicationsandMethods
148