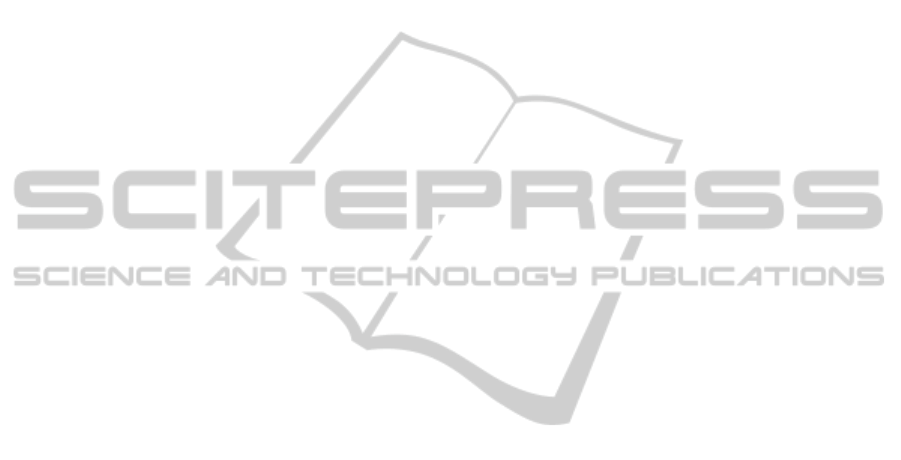
additive mixture of components is of particular
importance in addressing overfitting problem.
However, contemporary unsupervised
decomposition methods require label (diagnoses)
information to select component with cancer
relevant variables. Such component is useful for
biomarker identification studies but it does not
suffice to learn diagnostic model. In addition to that,
most of existing unsupervised decomposition
methods assume linear additive mixture model of a
sample. Herein, we have proposed an approach for
variable selection by decomposing each sample
individually into sparse components according to
nonlinear mixture model of a sample, whereas
decomposition is performed with respect to a
reference sample that represents negative (healthy)
class. This enables to select cancer related
components automatically and use them for either
biomarker identification studies or learning
diagnostic models. It is conjectured that outlined
properties of proposed approach to variable selection
enabled competitive diagnostic accuracy with small
number of variables on cancer related human gene
and protein expression datasets. While proposed
approach to variable selection is developed for
binary (two-class) problems its extension for multi-
category classification problems is aimed for the
future work.
ACKNOWLEDGEMENTS
This work has been supported through grant
9.01/232 funded by the Croatian Science
Foundation.
REFERENCES
Aliferis, C. F., et al. (2010a). Local Causal and Markov
Blanket Induction for Causal Discovery and Feature
Selection for Classification - Part I: Algorithms and
Empirical Evaluation. J. Mach. Learn. Res., 11, 171-
234.
Aliferis, C. F., et al. (2010b). Local Causal and Markov
Blanket Induction for Causal Discovery and Feature
Selection for Classification - Part II: Analysis and
Extensions. J. Mach. Learn. Res., 11, 235-284.
Alon, U., et al. (1999). Broad patterns of gene expression
revealed by clustering analysis of tumor and normal
colon tissues probed by oligonucleotide arrays. Proc.
Natl. Acad. Sci. USA, 96, 6745-6750.
Alter, O., Brown, P. O., and Botstein, D. (2000). Singular
value decomposition for genome-wide expression data
processing and modeling. Proc. Natl. Acad. Sci. USA,
97, 10101-10106.
Aronszajn, N. (1950). The theory of reproducing kernels.
Trans. of the Amer. Math. Soc., 68, 337-404.
Beck, A. and Teboulle, M. (2009). A fast iterative
shrinkage-thresholding algorithm for linear inverse
problems. SIAM J. on Imag. Sci., 2, 183-202.
Ben-Dor, A., Shamir, R., and Yakhini, Z. (1999).
Clustering gene expression patterns. J. Comp. Biol., 6,
281-297.
Brown, G. (2009). A New Perspective for Information
Theoretic Feature Selection. J. Mach. Learn. Res., 5,
49-56.
Brunet, J. P., et al. (2004). Metagenes and molecular
pattern discovery using matrix factorization. Proc.
Natl. Acad. Sci. USA, 101, 4164-4169.
Chang, C. C., and Lin, C. J. (2003). LIBSVM: a library for
support vector machines.
Cichocki, A., et al. (2010). Nonnegative Matrix and
Tensor Factorizations. John Wiley, Chichester.
Decramer, S., et al. (2008). Urine in clinical proteomics.
Mol Cell Proteomics, 7, 1850-1862.
Dudoit, S., Fridlyand, J., and Speed, T. P. (2002).
Comparison of Discrimination Methods for the
Classification of Tumors Using Gene Expression Data.
J. of the Amer. Stat. Assoc., 97, 77-87.
Gao, Y., and Church, G. (2005). Improving molecular
cancer class discovery through sparse non-negative
matrix factorization. Bioinformatics, 21, 3970-3975.
Gillis, N., and Vavanis, S. A. (2012). Fast and Robust
Recursive Algorithms for Separable Nonnegative
Matrix Factorization, arXiv , v2.
Girolami, M., and Breitling, R. (2004). Biologically valid
linear factor models of gene expression.
Bioinformatics, 20, 3021-3033.
Gribonval, R., and Zibulevsky, M. (2010). Sparse
component analysis. In Jutten, C., and Comon, P.
(eds.), Handbook of Blind Source Separation,
Elsevier, pp. 367-420.
Guyon, I., et al. (2002). Gene selection for cancer
classification using support vector machines. Machine
Learning, 46, 389-422.
Guyon, I., Elisseeff, A. (2002). An introduction to variable
and feature selection. J. of Machine Learning Res., 3,
1157-1182.
Harmeling, S., Ziehe, A., and Kawanabe, M. (2003).
Kernel-Based Nonlinear Blind Source Separation,
Neural Comput., 15, 1089-1124.
Hyvärinen A., Karhunen J., and Oja E. (2001).
Independent Component Analysis. John Wiley & Sons,
New York.
Hoyer, P. O. (2004). Non-negative matrix factorization
with sparseness constraints, J. Mach. Learn. Res., 5,
1457-1469.
Jutten, C., Babaie-Zadeh, M., and Karhunen, J. (2010).
Nonlinear mixtures. In Jutten, C., and Comon, P.
(eds.), Handbook of Blind Source Separation,
Elsevier, pp. 549-592.
Kim, H., and Park, H. (2007). Sparse non-negative matrix
factorizations via alternating non-negativity
constrained least squares for microarray data analysis.
ANonlinearMixtureModelbasedUnsupervisedVariableSelectioninGenomicsandProteomics
91