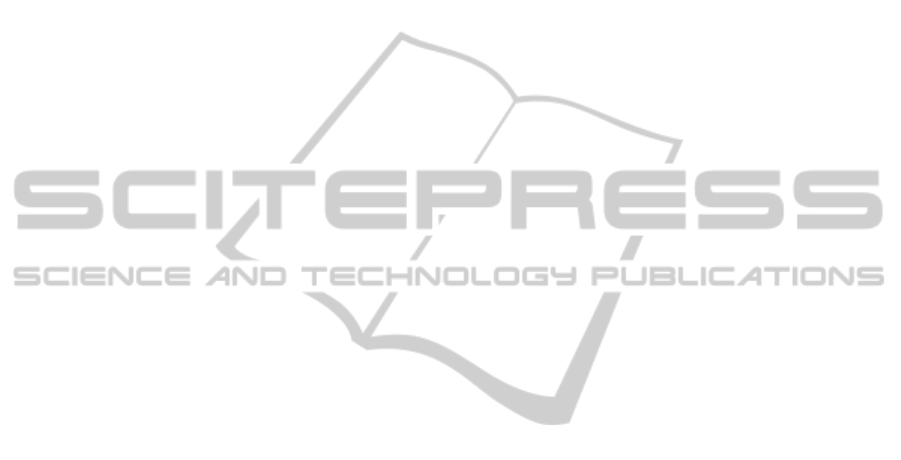
5 CONCLUDING REMARKS
I presented a novel online POMDP algorithm which
performs at least twice as good as the other algo-
rithms on a particular grid-world problem. The basic
algorithm with mean-as-threshold belief-state com-
pression always collected the most items (ic). How-
ever, because it takes more than twice (for h = 3) or
three times (for h = 4) as long as OUCEF to select
the next action, its effectiveness is significantly be-
low OUCEF’s (w.r.t. ic/s). OUCEF’s nominal perfor-
mance (ic) is comparable with that of the other algo-
rithms over the four experiment parameter combina-
tions.
The effectiveness of the OUCEF algorithm is due
to (i) unifying the branches due to nondeterministic
observations by collecting all belief-nodes at the ends
of these branchesinto one set B, and then (ii) selecting
the state most representative of B, by calculating the
expected values of the features of the states in B.
The aspect of this work most in need of attention is
to validate the approach on different benchmark prob-
lems. It might be the case that the OUCEF algorithm
is well suited to the kind of grid-world problems pre-
sented here, but to few other problems. Or it might
be suited to many kinds of problems. This paper is,
however, a first step in introducing and testing the al-
gorithm. At the very least, the new ideas presented
here might lead other researchers to new insights in
their online POMDP algorithm. A theoretical analy-
sis of the optimality of OUCEF is also required and
could lead to interesting insights.
REFERENCES
He, R., Brunskill, E., and Roy, N. (2011). Efficientplanning
under uncertainty with macro-actions. Journal of Ar-
tificial Intelligence Research (JAIR), 40:523–570.
Kaelbling, L., Littman, M., and Cassandra, A. (1998). Plan-
ning and acting in partially observable stochastic do-
mains. Artificial Intelligence, 101(1–2):99–134.
Koenig, S. (2001). Agent-centered search. Artificial Intelli-
gence Magazine, 22:109–131.
Kurniawati, H., Du, Y., Hsu, D., and Lee, W. (2011). Mo-
tion planning under uncertainty for robotic tasks with
long time horizons. International Journal of Robotics
Research, 30(3):308–323.
Li, X., Cheung, W., Liu, J., and Wu, Z. (2007). A
novel orthogonal NMF-based belief compression for
POMDPs. In Proceedings of the Twenty-fourth In-
ternational Conference on Machine Learning (ICML-
07), pages 537–544, New York, NY, USA. ACM
Press.
Lovejoy, W. (1991). A survey of algorithmic methods for
partially observed Markov decision processes. Annals
of Operations Research, 28:47–66.
McAllester, D. and Singh, S. (1999). Approximate plan-
ning for factored POMDPs using belief state simplifi-
cation. In Proceedings of the Fifteenth Conference on
Uncertainty in Artificial Intelligence (UAI-99), pages
409–416, San Francisco, CA. Morgan Kaufmann.
Monahan, G. (1982). A survey of partially observable
Markov decision processes: Theory, models, and al-
gorithms. Management Science, 28(1):1–16.
Paquet, S., Tobin, L., and Chaib-draa, B. (2005). Real-time
decision making for large POMDPs. In Advances in
Artificial Intelligence: Proceedings of the Eighteenth
Conference of the Canadian Society for Computa-
tional Studies of Intelligence, volume 3501 of Lecture
Notes in Computer Science, pages 450–455. Springer
Verlag.
Pineau, J., Gordon, G., and Thrun, S. (2003). Point-based
value iteration: An anytime algorithm for POMDPs.
In Proceedings of the International Joint Conference
on Artificial Intelligence (IJCAI), pages 1025–1032.
Poupart, P. and Boutilier, C. (2003). Value-directed com-
pression of POMDPs. In Advances in Neural Infor-
mation Processing Systems (NIPS 2003), pages 1547–
1554. MIT Press, Massachusetts/England.
Rens, G. and Ferrein, A. (2013). Belief-node condensa-
tion for online pomdp algorithms. In Proceedings of
IEEE AFRICON 2013, pages 1270–1274, Red Hook,
NY 12571 USA. Institute of Electrical and Electronics
Engineers, Inc.
Ross, S., Pineau, J., Paquet, S., and Chaib-draa, B. (2008).
Online planning algorithms for POMDPs. Journal of
Artificial Intelligence Research (JAIR), 32:663–704.
Roy, N., Gordon, G., and Thrun, S. (2005). Finding
approximate POMDP solutions through belief com-
pressions. Journal of Artificial Intelligence Research
(JAIR), 23:1–40.
ICAART2015-InternationalConferenceonAgentsandArtificialIntelligence
246