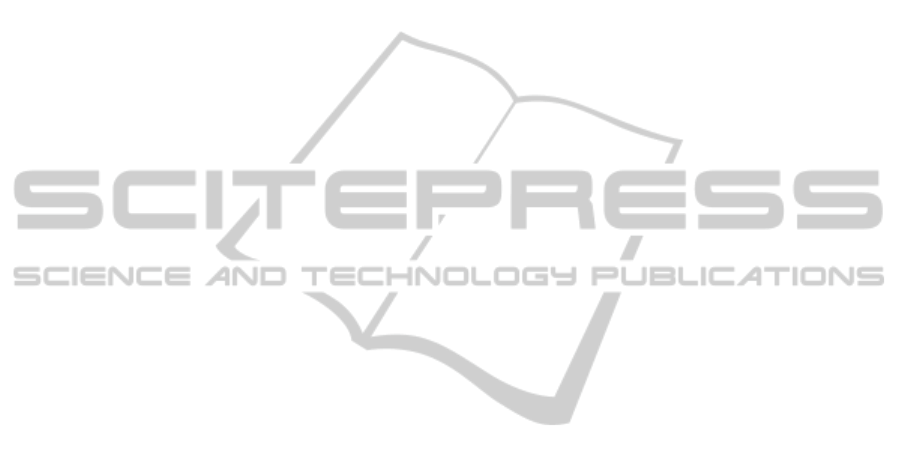
three databases by using data mining tools such as
classification and association rules is a sophisticated
approach to predict and describe the risk of lifestyle
diseases when liked with food consumption patterns
in a big database for a specific population belonging
to a demographical subgroup. Furthermore since this
study uses a large food consumption database, the
incorporated rules are by far more realistic than rules
that have been incorporated by using food
consumption data from interviewers in survey,
questionnaires or polls, since these data retrieval
methods have the unfortunate disadvantage that the
interviewers claim their wishful thoughts rather than
their daily food consumption facts.
In fact, our demographical and health data do not
possess the level of distinctive accuracy we aimed for
in order to link these patterns with specific age groups
and races among different geographical areas of the
U.S. west coast. Since such a thorough research on
the internet back to the year of 2000 revealed itself a
laborious one, we have chosen two demographical
attributes, age group and race, in order to demonstrate
the feasibility of our concept.
Furthermore, one limitation of our study consists
of focusing on data from customers of a specific
grocery chain. This might create bias in the outcomes
and therefore the rules produced. Further research is
needed to explore the grocery shopping patterns of
customers based on multiple grocery chain stores.
In the section of data mining and knowledge
interpretation, we have listed some preliminary
interesting rules along with a corresponding
interpretation.
In epidemiology, disease incidence and
nutritional behavior could be combined to assign
population attributable risks. In data mining, these
risks are detected because of the combination of huge
databases, which are linked by foreign keys. Risks
assigned to the population could be checked for
individuals by comparing the individual’s profile to
the pre-defined population risk patterns. Thus, typical
profiles related to high disease risks as a result of a
nutritional behavior could be collected and be made
available to dietitians as checklists to facilitate the
detection of those patients and clients who are at risk.
For our future work, we intend to gather more
actual and precise data coming preferably from a
grocery chain in Switzerland or Europe. Our
cooperation with the health institutes in Switzerland
and in the European countries will be essential to
receive accurate demographical and health data,
which should help us derive interesting and possibly
novel hidden patterns. Our ultimate goal is to find
valid rules in order to be able to predict and prevent
lifestyle diseases by detecting critical food
consumption patterns. Data mining is an enormously
mighty technique that allows us to help reach our goal
without the common limitations of the previous
research efforts, which used the classical statistical
hypothesis-bound methods.
REFERENCES
WHO, World Health Organization Geneva 2003, Diet,
Nutrition and the Prevention of Chronic Diseases,
Report of a Joint WHO/FAO Expert Consultation.
I. P Hearty and M. J Gibney, 2008, Analysis of meal
patterns with the use of supervised data mining
techniques artificial neural networks and decision trees,
88:1632–42. American Society for Nutrition.
M. Sulaiman Khan, Maybin Muyeba, Frans Coenen, 2008,
On Exraction of Nutritonal Patterns (NPS) using Fuzzy
Association Rule Mining, Healthinf 2008.
R. Agrawal and R. Srikant, 1996, Quest Synthetic Data
Generator, IBM Almaden Research Center.
L. Manikonda, R. Mall, V. Pudi, 2011, "Mining Nutrition
Survey Data", SSCI 2011, CIDM 2011, Paris, France.
J.D. Kinsey, P. Wolfson, N. Katsaras, B. Senauer, 2001,
Data mining A segmentation analysis of US grocery
shoppers, Working paper (Univer -sity of Minnesota.
Retail Food Industry Center), 01-01.
S. Kumar, V. Bishnoi, 2011, Indian Consumer Food
Shopping Behaviour and their Choice & Preference for
Packaged Food and Food Retailers, an Exploratory
Study, Proceedings for 2011 International Research
Conference And Colloquium, Contemporary Research
Issues and Challenges in Emerging Economies.
J. M. Harris and N. Blisard, 2002, Food -Con-sumption
Patterns among Elderly Age Groups.
N. Habib, S. Inam, S. Batool, S. Naheed and S. Siddiqui,
2013, Nutritional Pattern and its Impact on the Health:
A Case Study of Tehsil Kot Addu, Punjab, Pakistan,
International Journal of Humanities and Social Science,
Vol. 3 No. 10,Special Issue, May 2013.
Harris Polls, 2010, http://www.harrisinteractive.com
/NewsRoom/ HarrisPolls/tabid/447/ctl/
ReadCustom%20Default/mid/1508/ArticleId/614/Defa
ult.aspx.
RecSysWiki, 2012, http://recsyswiki.com/wiki/ Grocery_
shopping_datasets.
US Census, 2000, http://www.census.gov/ces/
dataproducts/ demographicdata.html.
http://www.publichealth.lacounty.gov.
http://www.cancer-rates.info/ca/index.php.
http://sandiegohealth.org/disease/diabetes/
diabetes2001.pdf.
http://www.cdph.ca.gov/pubsforms/Pubs/
OHIRmentalhealthCareCA2001.pdf.
http://www.healthysf.org/bdi/determinants/ hypertension/
http://www.sdcounty.ca.gov/hhsa/programs/
http://adai.washington.edu/pubs/ infobriefs/ ADAI-IB-
2004- 06.pdf.
HEALTHINF2015-InternationalConferenceonHealthInformatics
244