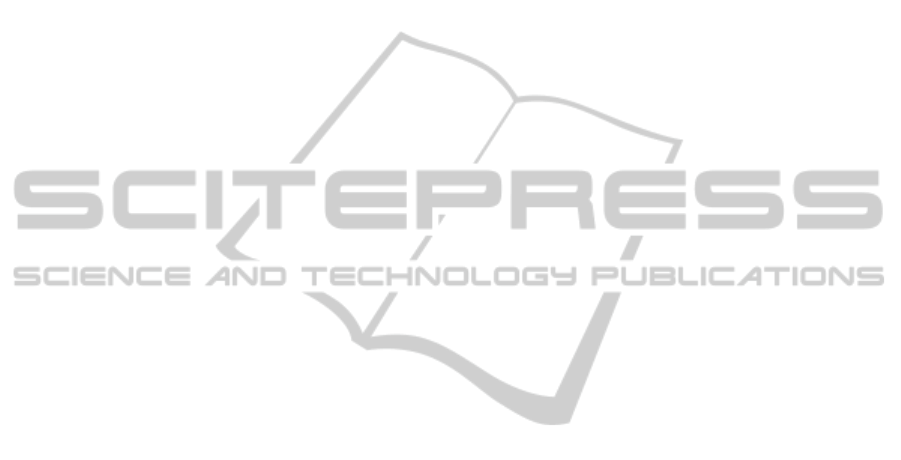
2003)(Bakshy et al., 2011), the authors conclude that
there exist some influential nodes that foster diffusion
of innovation throughout the network. They show
this by presenting different probabilistic models that
allow estimating the node activation from the signals
received by other users. In (Aral and Walker, 2012),
the authors show that influential individuals tend
not to be susceptible to influence from others, while
susceptible individuals tend not to be influential.
Our work is different and adds to the works above.
Our interests are not in studying the adoption or dif-
fusion processes per se in social networks. The focus
of our work is on identifying the most appropriate en-
try points to deploy in order to initiate product adop-
tion and diffusion. In particular, in social networks,
we aim to mine the underlying network structure
and utilise characteristics of individual nodes such as
influencing (referent) power to determine the most
promising entry points for seeding the innovation.
The contribution of this work is in providing a mech-
anism able to identify suitable entry points for the in-
novation in the social network in order to achieve a
more efficient early adoption, in terms of cost, rate of
adoption and subsequent benefits to the firm introduc-
ing it. Moreover, since the mechanism needs a global
view of the social network it must be deployed by the
network owner; that is, social network firms (Face-
book Inc., Twitter Inc, etc.) might offer the use of
the mechanism as a service for other companies inter-
ested in advertising and spreading their services and
products.
6 CONCLUSIONS
In this paper, we have presented a mechanism to se-
lect suitable entry points in a social network to intro-
duce innovation, so fostering its acceptance and its
diffusion. For that purpose we have used the under-
lying structure of the network, as well as the power
some users exercise on others. We have empirically
validated the theoretical approach with a set of exper-
iments over a Facebook dataset and two examples of
random networks. As future work we intend to study
an extension of the mechanism in order to deal with
dynamic social networks, in which structural proper-
ties change quickly due to the evolution of the links
in the network. Another interesting avenue for future
work is to build a macro model for using the mecha-
nism in an inter-network environment; i.e. to be able
to use the mechanism to estimate the centrality and
the power of users in different networks. This may be
of relevance especially when new users connect to a
network.
ACKNOWLEDGEMENTS
This work has been partially supported by the Spanish
Ministry of Economy and Competitiveness through
grants CSD2007-00022 ("Agreement Technologies",
CONSOLIDER-INGENIO2010), TIN2012-36586-
C03-02 ("iHAS") as well as by the Autonomous
Region of Madrid through grant P2013/ICE-3019
("MOSI-AGIL-CM", co-funded by EU Structural
Funds FSE and FEDER").
REFERENCES
Aral, S. and Walker, D. (2012). Identifying Influential and
Susceptible Members of Social Networks. Science,
337(6092):337–341.
Bakshy, E., Hofman, J. M., Mason, W. A., and Watts, D. J.
(2011). Everyone’s an influencer: Quantifying influ-
ence on twitter. In Proceedings of the Fourth ACM In-
ternational Conference on Web Search and Data Min-
ing, pages 65–74, New York, NY, USA. ACM.
Bass, F. (1969). A new product growth model for consumer
durables. Management Science, 15.
Calantone, R. J., Cavusgil, S., and Zhao, Y. (2002). Learn-
ing orientation, firm innovation capability, and firm
performance. Industrial Marketing Management,
31(6):515 – 524.
Deroïan, F. (2002). Formation of social networks and dif-
fusion of innovations. Research Policy, 31(5):835 –
846.
Fasli, M. (2006). On the relationship between roles and
power: preliminary report. In Proceedings of the
2006 ACM symposium on Applied computing, SAC
’06, pages 313–318, New York, NY, USA. ACM.
Freeman, L. C. (1977). A Set of Measures of Centrality
Based on Betweenness. Sociometry, 40(1):35–41.
Goldenberg, J., Han, S., Lehmann, D. R., and Hong, J. W.
(2009). The role of hubs in the adoption process. Jour-
nal of Marketing, 73(2):1–13.
Jackson, M. O. (2008). Social and Economic Networks.
Princeton University Press, Princeton, NJ, USA.
Kempe, D., Kleinberg, J., and Tardos, E. (2003). Maximiz-
ing the spread of influence through a social network.
In Proceedings of the Ninth ACM SIGKDD Interna-
tional Conference on Knowledge Discovery and Data
Mining, pages 137–146, New York, USA. ACM.
López-Pintado, D. (2008). Diffusion in complex social net-
works. Games and Economic Behavior, 62(2):573 –
590.
Luu, D. M., Lim, E.-P., Hoang, T.-A., and Chua, F. C. T.
(2012). Modeling diffusion in social networks using
network properties. In Proceedings of the 6th ICWSM.
McAuley, J. J. and Leskovec, J. (2012). Learning to dis-
cover social circles in ego networks. In 26th Annual
Conference on Neural Information Processing Sys-
tems, pages 548–556.
EntryPointMatters-EffectiveIntroductionofInnovationinSocialNetworks
25