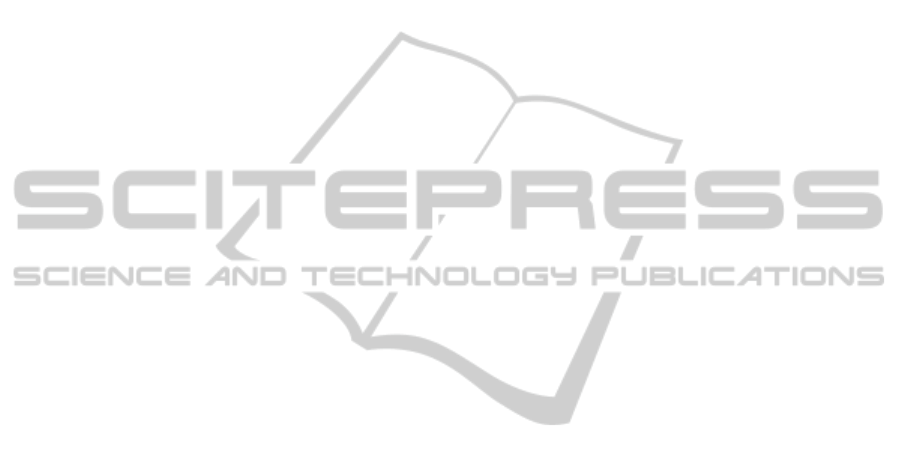
normalization would have bought the feature to a
subject-specific level, which is an important step in a
discrimination method (Vaseghi, 2008).
A limitation of the present validation consists in
the use of the the Oxford scale as validation criteria
for a correct discrimination. The Oxford scale
describes a subject’s ability to contact maximally the
PFM and was assed once. Each subject conducted 4
MVC protocols on 2 different days. Since the
outcome of each protocol has not been rated
separately, unsuccessful completion of the protocols
for healthy subjects may have occurred. The
histogram of the central frequency estimated by the
proposed approach (see Figure 3) shows that healthy
subjects have a very compact cluster. In contrast,
weak PFM subjects have a histogram with a long tail
into the high frequency domain (> 60Hz).
Discrimination errors are due to a misclassification
of weak PFM subjects from this marginal tail of the
histogram as healthy subjects. Thus, the limitation
related to the Oxford scale should not have an
influence on the performance of the proposed
algorithm presented herein.
5 CONCLUSIONS
Wavelet decomposition together with AR-modeling
provides a method for discrimination between
healthy and post-partum subjects with weak PFM
capabilities that outperforms classical FFT-based
methods.
REFERENCES
Akay, M., 1995. Wavelets in biomedical engineering.
Annals of Biomedical Engineering, pp. 531-542.
Auchincloss, C. C. & McLean, L., 2009. The reliability of
surface EMG recorded from the plevic floor muscles.
Journal of Neuroscience Methods, pp. 85-96.
Beck, T. et al., 2007. Does the frequency content of the
surface mechanomyographic signal reflect motor unit
firing rates? A brief review. J Electromyogr Kinesiol.,
pp. 1-13.
Bø, K. & Finckenhagen, H., 2001. Vaginal palpation of
pelvic floor muscle strength: inter-test reproducibility
and comparison between palpation and vaginal
squeeze. Acta Obstet Gynecol Scand., pp. 883-887.
Bø, K. & Sherburn, M., 2005. Evaluation of female plevic
floor muscle function and strength. PHYS THER., pp.
269-282.
Carlo, J., De Luca, L. & Gilmore, D., 2010. Filtering the
surface EMG signal: Movement artifact and baseline
contamination. Journal of Biomechanics, p. 1573–
1579.
Croce, R., Miller, K., Chamberlin, J. & Filipovic, D., kein
Datum Wavelet analysis of Quadriceps power spectra
and amplitude under varying levels of contraction
intensity and velocity. ‘Accepted Article’, doi:
10.1002/mus.24230.
Guyton, A. C. & Hall, J. E., 2011. Textbook of Medical
Physiology. Philadelphia: Elsevier.
Hermens, H. J., Freriks, B., Disselhorst-Klug, C. & Rau,
G., 2000. Developement of recommendations for
SEMG sensors and sensor placement procedures. J
Electromyogr Kinesiol. , pp. 361-74.
Lauper, M. et al., 2009. Plevic floor stimulation: what are
the good virbrations?. Neurourol Urodyn., pp. 405-10.
Shishido, K. et al., 2008. Influence of pelvic floor muscle
contraction on the profile of vaginal closure pressure
in continent and stress urinary incontinent women. J.
Urol., p. 1917–22.
Tscharner, V., 2000. Intensity analysis in time frequency
space of surface myoelectric signals by wavelets of
specified resolution. Journal of Electromyography and
Kinesiology, pp. 433-445.
Tscharner, V., Geopfert, B. & Nigg, B., 2003. Changes in
EMG signals for the muscle tibialis anterior while
running barefoot or with shoes resolved by non-
linearly scaled wavelets. Journal of Biomechanics, pp.
1169-76.
Vaseghi, S. V., 2008. Advanced Digital Signal Processing
and Noise Reduction. s.l.:JOHN WILEY & SONS,
LTD.
Vetter, R., 1999. Extraction of Efficient and Characteristic
Features of Multidimensional Time Series, Lausanne:
PhD Thesis, Swiss Federal Institute of
Technology.Vetter, R. et al., 1998. Subband modeling
of the human neuro-cardiovascular system: new
insights into cardiovascular regulation. Ann. Biomed.
Eng., pp. 293-307.
DiscriminationofHealthyandPost-partumSubjectsusingWaveletFilterbankandAuto-regressiveModelling
137