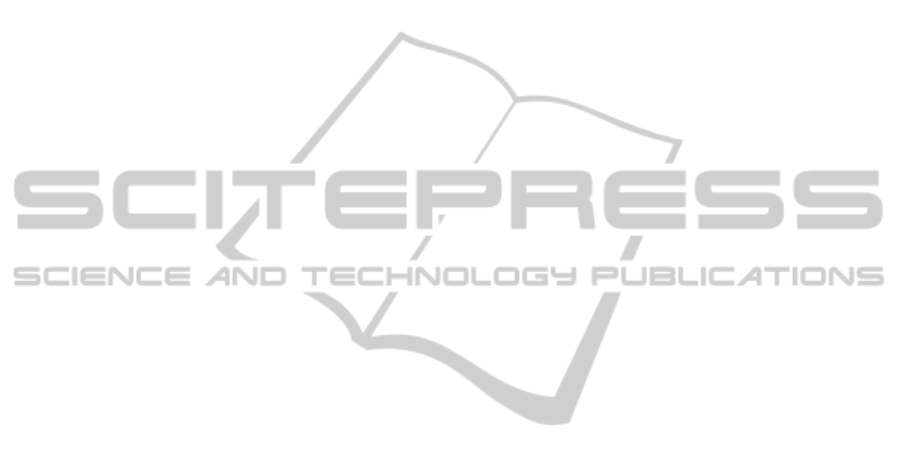
7 CONCLUSION AND RELATED
WORK
This paper tackled the question of how to measure
inconsistency of a knowledge base, how to compare
knowledge bases in terms of inconsistency and infor-
mation and how to measure distance between knowl-
edge bases. Given an inconsistent knowledge base,
our goal is to decrease its inconsistency and/or find
the closest consistent knowledge base.
Namely, we proposed to use the operation ϕ
del(α
i
)
as a basic operation for decreasing inconsistency of a
knowledge base; its advantage is that it yields a mini-
mal change of a knowledge base. We defined a partial
order on the set of knowledge bases and showed
its link with existing inconsistency and information
measures. We also introduced operations Σ
del(α
i
)
and
showed that they define the closest consistent knowl-
edge bases according to . Furthermore, we defined
a class of metrics that can be used to measure dis-
tances between two knowledge bases as well as the
distance from a knowledge base to the closest con-
sistent knowledge base. We formally showed that
our class of metrics agrees with an intuitive distance.
Namely, whatever the value of parameter p, deleting
a co-atom that appears in the least number of formu-
lae in a knowledge base is the minimal change (in the
sense of distance measured by d
p
) required to obtain
a consistent knowledge base. Also, we proved that
those metrics are compatible with , which means
that the distance between a knowledge base Σ and
consistency decreases whenever our minimal weak-
ening is applied to Σ.
The closest related paper is the work by Grant
and Hunter discussed in the introduction (Grant and
Hunter, 2011b). We are inspired by that paper but we
use only one operation instead of three. To be more
precise, Grant and Hunter proposed three classes of
operations, but given a concrete formula, say ϕ = x ∨y
in a concrete knowledge base Σ, they do not say how
to practically weaken ϕ. Our paper offers the answers
to this question. Also, we compare stepwise inconsis-
tency resolution and one-step approach where we go
directly to the closest consistent knowledge base.
There is another work that might seem very close
to the second part of our paper (Grant and Hunter,
2013). But indeed, there is a huge difference, since
they introduce metrics to measure distances between
models, which is not the case in our paper. We mea-
sure the distance between knowledge bases using a
variation of p-norms, applied on symmetric differ-
ence of models of formulae of two knowledge bases.
There is also related work on inconsistency
measurement for probabilistic logics (Picado-Mui
˜
no,
2011; Thimm, 2009), where p-norms are used to
calculate measure of inconsistency of a probabilistic
knowledge base. But unlike in our paper, in those pa-
pers the p-norms are used to determine the distance
between all the functions (which are not probability
measures) satisfying the (inconsistent) set of proba-
bility requirements from the knowledge base, and the
closest probability measure.
ACKNOWLEDGEMENTS
Dragan Doder was supported by the National Re-
search Fund (FNR) of Luxembourg through project
PRIMAT. The authors would like to thank S
´
ebastien
Konieczny for his help.
REFERENCES
Doder, D., Raskovic, M., Markovic, Z., and Ognjanovic,
Z. (2010). Measures of inconsistency and de-
faults. International Journal of Approximate Reason-
ing, 51(7):832–845.
Gabbay, D. M. and Hunter, A. (1991). Making incon-
sistency respectable: a logical framework for incon-
sistency in reasoning. In Fundamentals of Artificial
Intelligence Research, International Workshop (FAIR
’91), volume 535 of Lecture Notes in Computer Sci-
ence, pages 19–32. Springer.
Gabbay, D. M. and Hunter, A. (1993). Making incon-
sistency respectable: Part 2 - meta-level handling
of inconsistency. In Symbolic and Quantitative Ap-
proaches to Reasoning and Uncertainty, European
Conference (ECSQARU’93), volume 747 of Lecture
Notes in Computer Science, pages 129–136. Springer.
Grant, J. and Hunter, A. (2006). Measuring inconsistency in
knowledgebases. J. Intell. Inf. Syst., 27(2):159–184.
Grant, J. and Hunter, A. (2011a). Measuring consistency
gain and information loss in stepwise inconsistency
resolution. In Proceedings of the 7th European Con-
ference on Symbolic and Quantitative Approaches to
Reasoning with Uncertainty (ECSQARU’11), pages
362–373.
Grant, J. and Hunter, A. (2011b). Measuring the good and
the bad in inconsistent information. In Proceedings of
the 22nd International Joint Conference on Artificial
Intelligence (IJCAI’11), pages 2632–2637.
Grant, J. and Hunter, A. (2013). Distance-based measures
of inconsistency. In 12th Europen Conference on Sym-
bolic and Quantitative Approaches to Reasoning with
Uncertainty (ECSQARU’13), volume 7958 of Lecture
Notes in Computer Science, pages 230–241. Springer.
Hunter, A. and Konieczny, S. (2005). Approaches to
measuring inconsistent information. In Inconsistency
Tolerance [result from a Dagstuhl seminar], volume
3300 of Lecture Notes in Computer Science, pages
191–236. Springer.
ICAART2015-InternationalConferenceonAgentsandArtificialIntelligence
36