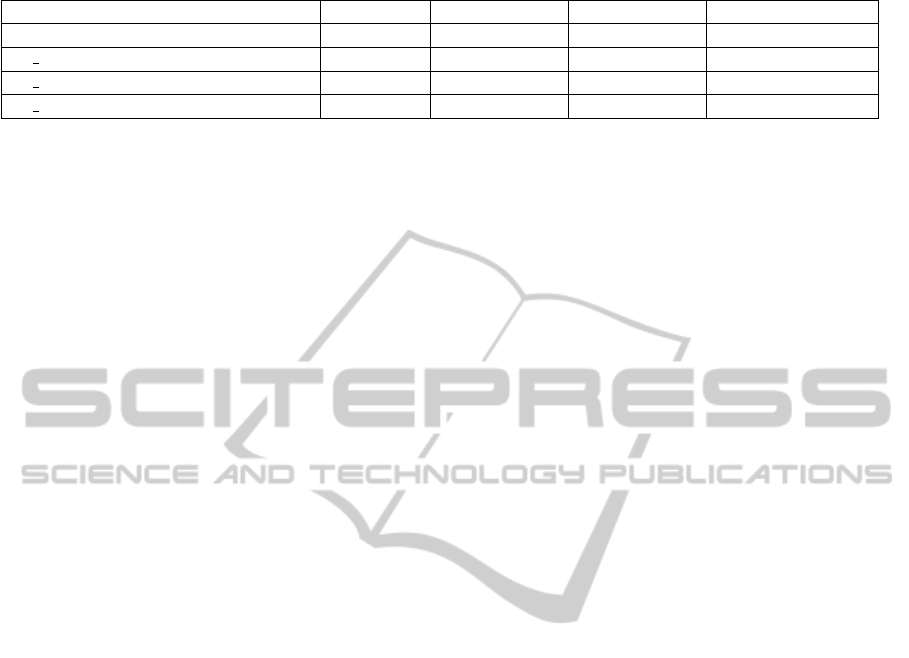
Table 2: Entity matching rates.
Recall (%) Precision (%) Fmeasure (%) Runtime (sec/doc)
EROCS (Chakaravarthy et al., 2006) 67.58 54,09 60,09 69.5
M EROCS
1
(+OCR error tolerance) 71,05 53,89 61,29 70.8
M EROCS
2
(+filtering) 71,05 54,77 61,86 6.2
M EROCS
3
(+entity resolution) 73,36 69,58 71,43 4,4
by segments in OCRed document. The extensions on
term matching and segment restructure of EROCS are
proven effective for OCRed documents which have
altered content and structure. A filtering step based
on data labeling reduces the runtime from 70.8 sec
to 6.2 sec per document. The pre-processing step of
entity resolution on the database improves the match-
ing rates with 2.31 points for the recall and 14.81
points for the precision and it decreases the runtime
with about 1.8 seconds by document. The results on a
dataset of 500 documents are promising and achieve
about 73% for recall and about 70% for precision.
The future work is to solve the problem of non-
contiguity of elements composing an entity. In case
of incomplete entity, we will choose from distant la-
beled elements those they complete correctly the en-
tity. The choice will be focused on the elements in-
creasing the matching score. Furthermore, we will
plan the use of other datasets, limited in this study to
supplier entities, in order to enlarge the field search
to all elements (close and distant) with more complex
spatial relations. The idea is to integrate the physical
and logical structures of the document and to exploit
them in the element searching. Another prospect is to
apply other methods for OCR matching and correc-
tion. A dictionary that maintains spell variations of
fields, such as abbreviations and character variations,
will be used.
ACKNOWLEDGEMENT
We would like to thank our collaborator ITESOFT for
providing real word data (images, OCRed documents
and database) for test.
REFERENCES
Bilenko, M. (2006). Adaptive blocking: Learning to scale
up record linkage. In Proceedings of the 6th IEEE
International Conference on Data Mining, pages 87–
96.
Bilenko, M., Mooney, R., Cohen, W., Ravikumar, P., and
Fienberg, S. (2003). Adaptive name matching in
information integration. IEEE Intelligent Systems,
18(5):16–23.
Chakaravarthy, V. T., Gupta, H., Roy, P., and Mohania, M.
(2006). Efficiently linking text documents with rele-
vant structured information. In International Confer-
ence on Very Large Data Bases, pages 667–678.
Cohen, W. W., Ravikumar, P., and Fienberg, S. E. (2003).
A comparison of string distance metrics for name-
matching tasks. In Proceedings of IJCAI-03 Workshop
on Information Integration, pages 73–78.
Fellegi, I. P. and Sunter, A. B. (1969). A theory for record
linkage. Journal of the American Statistical Associa-
tion, 64(328):1183–1210.
Hashemi, R. R., Ford, C., Bansal, A., Sieloff, S. D., and Tal-
burt, J. R. (2003). Building semantic-rich patterns for
extracting features from events of an on-line newspa-
per. In Proceedings of the IADIS International Con-
ference WWW/Internet, pages 627–634.
Laishram, J. and Kaur, D. (2013). Named entity recognition
in Manipuri: a hybrid approach. In The International
Conference of the German Society for Computational
Linguistics and Language Technology, volume 8105,
pages 104–110.
Lee, M.-L., Ling, T. W., and Low, W. L. (2000). Intelli-
clean: a knowledge-based intelligent data cleaner. In
ACM SIGKDD Conference on Knowledge Discovery
and Data Mining, pages 290–294.
Pereda, R. and Taghva, K. (2011). Fuzzy information ex-
traction on OCR text. In ITNG, pages 543–546.
Taghva, K., Beckley, R., and Coombs, J. S. (2006). The
effects of OCR error on the extraction of private infor-
mation. In Document Analysis Systems, pages 348–
357.
Wu, N., Talburt, J., Heien, C., Pippenger, N., Chiang, C.-
C., Pierce, E., Gulley, E., and Moore, J. (2007). A
method for entity identification in open source docu-
ments with partially redacted attributes. J. Comput.
Small Coll., 22(5):138–144.
Zhang, X., Zou, J., Le, D. X., and Thoma, G. R. (2010).
Investigator name recognition from medical journal
articles: a comparative study of svm and structural
svm. In Document Analysis Systems, ACM Interna-
tional Conference Proceeding Series, pages 121–128.
ICPRAM2015-InternationalConferenceonPatternRecognitionApplicationsandMethods
172