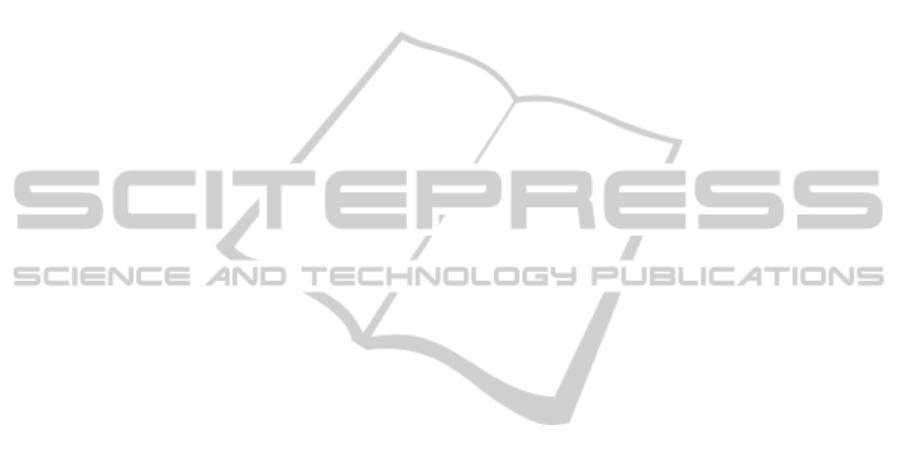
dered in order to remove irrelevant features. They are
applied to the features extracted using Co-occurrence
Features with distance d = 3, since this method, as
it was commented, provides the best performance.
After feature selection, process described in Section
2.3.2 is repeated: SVM is trained and a 10-fold cross-
validation is done. Table 4 shows accuracy obtained
by each method (INTERACT - INT, correlation-based
feature selection - CFS and consistency-based filter -
Cons), as well as the number of features selected by
each one.
As it was commented previously, Co-occurrence
Features with distance d = 3 provides the best rate
(93.97%). For this descriptor, 28 features are consi-
dered. Results after applying feature selection, pre-
sented in Table 4, show that CFS has the most aggres-
sive behaviour since it retains 32.14% of the features,
but at the expense of a slight decrease in the accuracy
of the classifier, while Cons and INTERACT retain
around the 50% of the initial features with no degra-
dation in performance. Thus, results obtained with
Co-occurrence Features (d = 3) can be remained but
with a significant reduction in the number of features.
Note that a reduction in the number of feature implies
a decrease in the time needed to compute the descrip-
tor. This time, which is not a matter of study in this
paper, could become a bottle neck when applying a
segmentation process in which a great amount of win-
dows have to be analyzed, and herein also lies the re-
levance of using feature selection.
5 CONCLUSIONS AND FUTURE
WORK
In this work, an automatic characterization of the
choroid layer in EDI OCT retinal images based on
textural information has been presented. The me-
thod consists in locating the region of interest through
an active contour-based segmentation and then, ex-
tracting texture descriptors to perform a classification
process with the purpose of discriminating between
regions belonging to the choroid from those corres-
ponding to other areas. Different texture descriptors
have been considered for the pattern recognition task
in order to perform a comparative study and deter-
mine the best one to represent this layer. Results show
that the choroid can be identified in a very effective
way using Co-ocurrences Features, surpassing pre-
vious works (Danesh et al., 2014). Feature selec-
tion has been applied, allowing to eliminate the irre-
levant/redundant features with no degradation in the
accuracy of the performance. Therefore, texture in-
formation extracted with this method can be used to
characterize this layer in a robust way.
As future work, a deeper study of textural infor-
mation with different descriptors could be done, as
well as more classifiers can be considered. Besides
that, this information must be included in a methodo-
logy that allows to tackle the accurate segmentation
of the lower choroid boundary.
ACKNOWLEDGEMENTS
This paper has been partly funded by Instituto de
Salud Carlos III under PI14/02161 project and the
Secretar
´
ıa de Estado de Investigaci
´
on, Desarrollo e
Innovaci
´
on of the Spanish Government (A. Gonz
´
alez-
L
´
opez acknowledges its support under FPI Grant Pro-
gram). The authors would like to thank Hospital do
Barbanza, Ribeira (Spain) for providing images.
REFERENCES
Alonso-Caneiro, D., Read, S. A., and Collins,
M. J. (2013). Automatic segmentation of
choroidal thickness in optical coherence tomog-
raphy. Biomed. Opt. Express, 4(12):2795–2812.
Besag, J. (1974). Spatial interaction and the statistical
analysis of lattice systems. Journal of the Royal
Statistical Society. Series B, 36:192–236.
Bill, A., Sperber, G., and Ujiie, K. (1983). Physiology
of the choroidal vascular bed. Int. Ophthalmol.,
6(2):101–107.
Bol
´
on-Canedo, V., Snchez-Maroo, N., and Alonso-
Betanzos, A. (2013). A review of feature selec-
tion methods on synthetic data. Knowl. Inf. Syst.,
34(3):483–519.
C¸ esmeli, E. and Wang, D. L. (2001). Texture segmen-
tation using gaussian-markov random fields and
neural oscillator networks. IEEE Transactions
on Neural Networks, 12(2):394–404.
Danesh, H., Kafieh, R., Rabbani, H., and Hajizadeh,
F. (2014). Segmentation of choroidal boundary
in enhanced depth imaging octs using a multires-
olution texture based modeling in graph cuts.
Computational and Mathematical Methods in
Medicine, 2014(479268).
Dash, M. and Liu, H. (2003). Consistency-based
search in feature selection. Artificial Intelli-
gence, 151(1-2):155–176.
Daubechies, I. (1992). Ten Lectures on Wavelets.
Society for Industrial and Applied Mathematics,
Philadelphia, PA, USA.
ChoroidCharacterizationinEDIOCTRetinalImagesBasedonTextureAnalysis
275