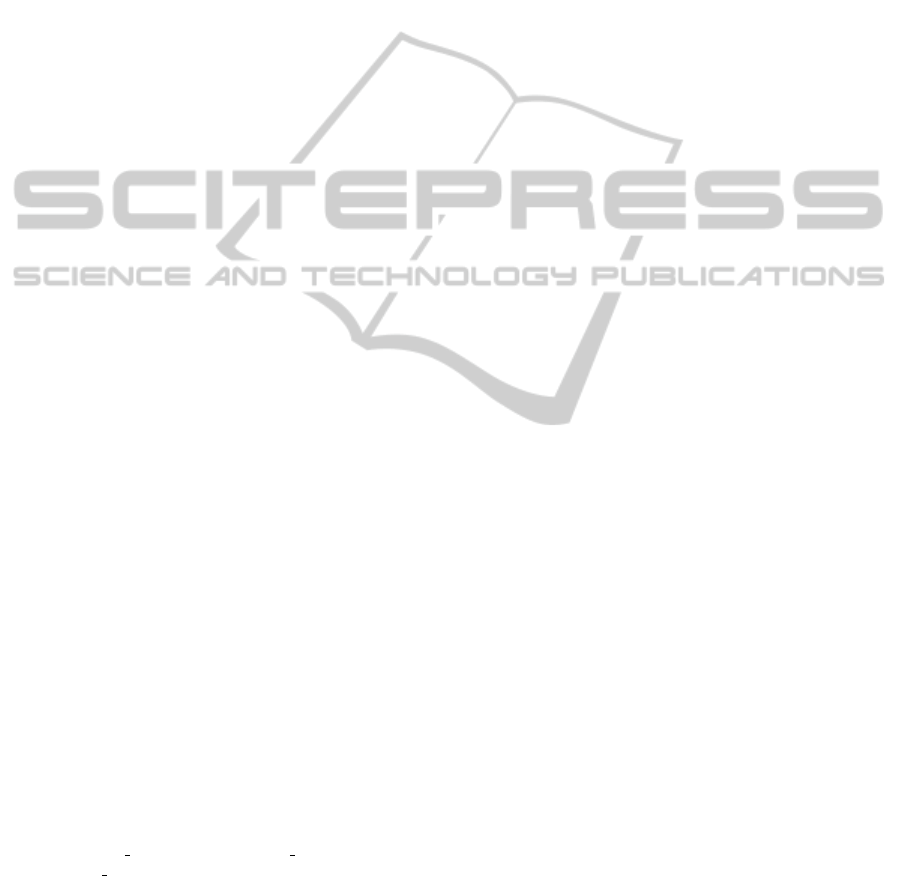
forming real-time detection of the region of interest
of the target skin lesion. In this work we also present
a methodology to automatically segment skin lesions
from dermatological images acquired via mobile de-
vices. The method was applied in 80 smartphone-
acquired images, achieving a mean Jaccard index
result of 81%, mean True Detection Rate of 96%
and mean Accuracy around 98%, confirming the
adequacy of the suggested automatic segmentation
methodology.
In order to expand this study in the near future,
we consider that is important to have a testing dataset
with more skin lesion images acquired via mobile de-
vices, manually segmented by different specialists in
the area and also investigate if the methodology is ro-
bust for different brands of mobile devices.
Above all, it is our goal to develop a mobile ap-
plication easily accessible for the general population,
with the aim of raise awareness and help both patients
and doctors in the early diagnosis of skin cancers.
ACKNOWLEDGEMENTS
This work was done under the scope of the project
“SMARTSKINS: A Novel Framework for Super-
vised Mobile Assessment and Risk Triage of Skin
Lesion via Non-invasive Screening” with reference
PTDC/BBB-BMD/3088/2012 financially supported
by Fundac¸
˜
ao para a Ci
ˆ
encia e a Tecnologia in Por-
tugal.
REFERENCES
Cavalcanti, P., Scharcanski, J., Di Persia, L., and Milone,
D. (2011). An ICA-based method for the segmenta-
tion of pigmented skin lesions in macroscopic images.
In 2011 Annual International Conference of the IEEE
Engineering in Medicine and Biology Society,EMBC,
pages 5993–5996.
Cavalcanti, P., Yari, Y., and Scharcanski, J. (2010). Pig-
mented skin lesion segmentation on macroscopic im-
ages. In 25th International Conference of Image and
Vision Computing New Zealand, pages 1–7.
Fraunhofer, P. A. (2014). Melanoma detection,
internal project. http://www.fraunhofer.pt/
en/fraunhofer aicos/projects/internal research/
melanoma detection.html.
Fraz, M. M., Remagnino, P., Hoppe, A., Uyyanonvara, B.,
Rudnicka, A. R., Owen, C. G., and Barman, S. A.
(2012). Blood vessel segmentation methodologies in
retinal images–a survey. Computer methods and pro-
grams in biomedicine, 108(1):407–433.
Ivanovici, M. and Stoica, D. (2012). Color diffusion model
for active contours-an application to skin lesion seg-
mentation. In 2012 Annual International Conference
of the IEEE, Engineering in Medicine and Biology So-
ciety (EMBC), pages 5347–5350. IEEE.
Jaccard, P. (1912). The distribution of the flora in the alpine
zone. 1. New phytologist, 11(2):37–50.
Mahmoud, M. and Al-Jumaily, A. (2011). Segmentation
of skin cancer images based on gradient vector flow
(GVF) snake. In 2011 International Conference on
Mechatronics and Automation (ICMA), pages 216–
220.
Manousaki, A. G., Manios, A. G., Tsompanaki, E. I.,
Panayiotides, J. G., Tsiftsis, D. D., Kostaki, A. K.,
and Tosca, A. D. (2006). A simple digital image
processing system to aid in melanoma diagnosis in
an everyday melanocytic skin lesion unit. a prelimi-
nary report. International Journal of Dermatology,
45(4):402–410.
Otsu, N. (1979). A threshold selection method from gray-
level histograms. IEEE Transactions on Systems, Man
and Cybernetics, 9(1):62–66.
Rosado, L., Castro, R., Ferreira, L., and Ferreira, M. (2012).
Extraction of ABCD rule features from skin lesions
images with smartphone. Studies in health technology
and informatics, 177:242–247.
Silveira, M., Nascimento, J. C., Marques, J. S., Marc¸al,
A. R., Mendonc¸a, T., Yamauchi, S., Maeda, J., and
Rozeira, J. (2009). Comparison of segmentation meth-
ods for melanoma diagnosis in dermoscopy images.
IEEE Journal of Selected Topics in Signal Processing,
3(1):35–45.
Tabatabaie, K., Esteki, A., and Toossi, P. (2009). Extraction
of skin lesion texture features based on independent
component analysis. Skin research and technology,
15(4):433–439.
HEALTHINF2015-InternationalConferenceonHealthInformatics
250