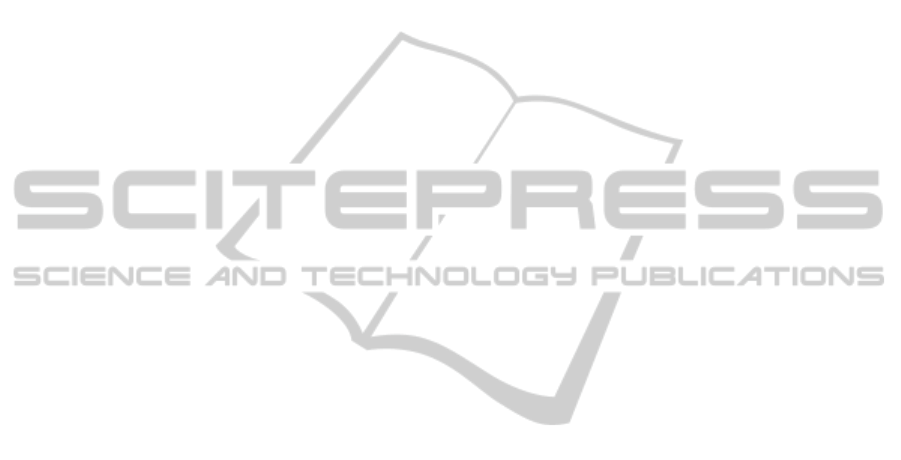
mative depending on the descriptors probability dis-
tribution.
The results obtained encourage further work in
this direction. Concatenation is a very simple and fast
(practically zero cost) method of combination, but it
does not make any distinction between the different
representations involved, even if they perform badly
with certain kind of images. A deeper research on
ensemble methods could prove to be fruitful.
Other way to look the results is that there are sev-
eral families of descriptors that can contribute with
rich information, but the a specific sampling method
detects only a few of these families. To identify
these sets of complementary descriptors and to de-
velop methods to extract them is another rich field of
research.
ACKNOWLEDGEMENTS
This work was supported by the following research
and fellowship grants: Fondecyt 1110854, DGIP-
UTFSM, MECESUP and CONICYT. The work of C.
Moraga was partially supported by the Foundation for
the Advance of Soft Computing, Mieres, Spain, and
by the CICYT Spain, under project TIN 2011-29827-
C02-01.
REFERENCES
Arandjelovi´c, R. (2012). Three things everyone should
know to improve object retrieval. In Proc. CVPR,
pages 2911–2918.
Arandjelovi´c, R. and Zisserman, A. (2013). All about
VLAD. In Proc. CVPR, pages 1578–1585.
Delhumeau, J., Gosselin, P.-H., J´egou, H., and P´erez, P.
(2013). Revisiting the VLAD image representation.
In Proc. ACM Int. Conf. on Multimedia, pages 653–
656.
Douze, M., Ramisa, A., and Schmid, C. (2011). Combin-
ing attributes and Fisher vectors for efficient image re-
trieval. In Proc. CVPR, pages 745–752.
Gong, Y., Lazebnik, S., Gordo, A., and Perronnin, F.
(2013). Iterative quantization: A procrustean ap-
proach to learning binary codes for large-scale image
retrieval. Pattern Analysis and Machine Intelligence,
35(12):2916–2929.
Gordo, A., Rodriguez-Serrano, J. A., Perronnin, F., and Val-
veny, E. (2012). Leveraging category-level labels for
instance-level image retrieval. In Proc. CVPR, pages
3045–3052.
Huiskes, M. J. and Lew, M. S. (2008). The MIR Flickr
retrieval evaluation. In Proc. ACM Int. Conf. on Mul-
timedia Information Retrieval, pages 39–43.
Jaakkola, T. S. and Haussler, D. (1999). Exploiting gen-
erative models in discriminative classifiers. In Proc.
Conf. on Advances in Neural Information Processing
Systems II, pages 487–493.
J´egou, H. and Chum, O. (2012). Negative evidences and
co-occurrences in image retrieval: the benefit of PCA
and whitening. In Proc. ECCV, pages 774–787.
J´egou, H., Douze, M., and Schmid, C. (2008). Hamming
embedding and weak geometric consistency for large
scale image search. In Proc. ECCV, volume I, pages
304–317.
J´egou, H., Douze, M., and Schmid, C. (2011). Prod-
uct quantization for nearest neighbor search. Pattern
Analysis and Machine Intelligence, 33(1):117–128.
J´egou, H., Perronnin, F., Douze, M., S´anchez, J., P´erez, P.,
and Schmid, C. (2012). Aggregating local image de-
scriptors into compact codes. Pattern Analysis and
Machine Intelligence, pages 1704–1716.
Lowe, D. G. (2004). Distinctive image features from scale-
invariant keypoints. International Journal of Com-
puter Vision, 60(2):91–110.
Manning, C. D., Raghavan, P., and Schtze, H. (2008). In-
troduction to Information Retrieval. Cambridge Uni-
versity Press, New York.
Nister, D. and Stewenius, H. (2006). Scalable recognition
with a vocabulary tree. In Proc. CVPR, pages 2161–
2168.
Perronnin, F. and Dance, C. R. (2007). Fisher kernels on
visual vocabularies for image categorization. In Proc.
CVPR, pages 1–8.
Perronnin, F., Liu, Y., Snchez, J., and Poirier, H. (2010a).
Large-scale image retrieval with compressed Fisher
vectors. In Proc. CVPR, pages 3384–3391.
Perronnin, F., S´anchez, J., and Mensink, T. (2010b). Im-
proving the Fisher kernel for large-scale image classi-
fication. In Proc. ECCV, pages 143–156.
Philbin, J., Chum, O., Isard, M., Sivic, J., and Zisserman, A.
(2007). Object retrieval with large vocabularies and
fast spatial matching. In Proc. CVPR, pages 1–8.
S´anchez, J., Perronnin, F., Mensink, T., and Verbeek, J.
(2013). Image classification with the Fisher vector:
Theory and practice. International Journal of Com-
puter Vision, 105(3):222–245.
Shahbaz Khan, F., Anwer, R., van de Weijer, J., Bagdanov,
A., Vanrell, M., and Lopez, A. (2012). Color attributes
for object detection. In Proc. CVPR, pages 3306–
3313.
Tuytelaars, T. and Mikolajczyk, K. (2008). Local invariant
feature detectors: A survey. Foundations and Trends
in Computer Graphics and Vision, 3(3):177–280.
Wengert, C., Douze, M., and J´egou, H. (2011). Bag-of-
colors for improved image search. In ACM Multime-
dia, pages 1437–1440.
Zhang, S., Yang, M., Cour, T., Yu, K., and Metaxas, D.
(2012). Query specific fusion for image retrieval. In
Proc. ECCV, pages 660–673.
Zheng, L., Wang, S., Zhou, W., and Tian, Q. (2014). Bayes
merging of multiple vocabularies for scalable image
retrieval. In Proc. CVPR, pages 1963–1970.
CombiningFisherVectorsinImageRetrievalUsingDifferentSamplingTechniques
135