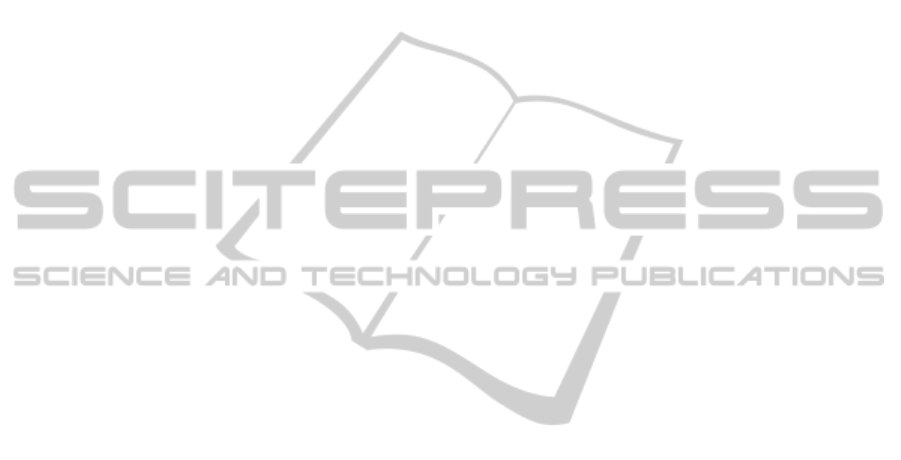
tion; (3) relevance of the partitioning method to guide
an FLTM-based GWAS pinpointing regions with sig-
nificantly associated SNPs. The CAST
bin
clustering
method was shown slightly different from CAST
real
and DBSCAN, from the clustering viewpoint. How-
ever, this difference was not reflected by a difference
in GWASs’ performances. Therefore, to the initial
question ”Which clustering method should be cho-
sen”, the answer for the Crohn’s disease WTCCC data
set relative to chromosome 2 would rather prioritize
easiness in tuning parameters. In our experiments so
far, the FLTM learning algorithm seems robust to the
choice of the clustering method, provided that the in-
trinsic parameters of the latter are appropriately set.
Further works include extending the current analysis
to other chromosomes, for the WTCCC data set, as
well as to other diseases, and extending our analysis
to other clustering methods.
It was the first time that the FLTM learning algo-
rithm was run on real GWAS data. It is questionable
whether the present study should be complemented by
intensive experiments run on simulated GWAS data
sets. Given the high processing times required as soon
as GWASs are addressed, and the recurring question
of generating sufficiently realistic GWAS data, a less
systematic approach, encompassing more diseases,
seems wholly relevant.
Finally, to return to the multilocus aspect of the
type of GWAS addressed here, one of our next tasks
is to compare the FLTM-based GWAS strategy with
the few other scalable multilocus approaches existing,
including BEAGLE (Browning and Browning, 2007).
ACKNOWLEDGEMENTS
The project SAMOGWAS (Specific Advanced MOd-
els for Genome Wide Association Studies) is sup-
ported by the French National Research Agency
(Agence Nationale de la Recherche, ANR). The au-
thors are also grateful to the Wellcome Trust Case
Control Consortium for providing the GWAS data
used in this study.
REFERENCES
Abel, H. and Thomas, A. (2011). Accuracy and Com-
putational Efficiency of a Graphical Modeling Ap-
proach to Linkage Disequilibrium Estimation. Statis-
tical Applications in Genetics and Molecular Biology,
10(1):Article 5.
Ackerman, M. and Ben-David, S. (2009). Clusterability: a
Theoretical Study. In Dyk, D. and Welling, M., ed-
itors, Twelfth International Conference on Artificial
Intelligence and Statistics (AISTATS09), Journal of
Machine Learning Research, Proceedings Track, vol-
ume 5, pages 1–8.
Balding, D. (2006). A Tutorial on Statistical Methods for
Population Association Studies. Nature Reviews Ge-
netics, 7(10):781–791.
Barrett, J., Hansoul, S., Nicolae, et al. (2008). Genome-
wide Association Defines more than 30 Distinct Sus-
ceptibility Loci for Crohn’s Disease. Nature Genetics,
40(8):955–962.
Ben-Dor, A., Shamir, R., and Yakhini, Z. (1999). Clus-
tering Gene Expression Patterns. In Third Annual In-
ternational Conference on Research in Computational
Molecular Biology (RECOMB99), pages 33–42.
Browning, B. and Browning, S. (2007). Efficient Multilo-
cus Association Testing for Whole Genome Associ-
ation Studies Using Localized Haplotype Clustering.
Genetic Epidemiology, 31:365–375.
Cahill, J. (2002). Error-Tolerant Clustering of Gene Mi-
croarray Data. Bachelors Honors Thesis, Boston Col-
lege, Massachusetts.
Ester, M., Kriegel, H.-P., Sander, J., and Xu, X. (1996). A
Density-Based Algorithm for Discovering Clusters in
Large Spatial Databases with Noise. In Second In-
ternational Conference on Knowledge Discovery and
Data Mining (KDD96), pages 226–231.
Fowlkes, E. and Mallows, C. (1983). A Method for Com-
paring Two Hierarchical Clusterings. Journal of the
American Statistical Association, 78(383):553–569.
Gabriel, S., Schaffner, S., Moore, J., et al. (2002). The
Structure of Haplotype Blocks in the Human Genome.
Science, 296(5576):2225–2229.
Gibbs, R., Belmont, J., Hardenbol, P., et al. (2003). The In-
ternational HapMap Project. Nature, 426(6968):789–
796.
Hubert, L. and Arabie, P. (1985). Comparing Partitions.
Journal of Classification, 2(1):193–218.
Meila, M. (2005). Comparing Clusterings: an Axiomatic
View. In Twenty-second International Conference on
Machine Learning (CML05), ACM, pages 577–584.
Mirkin, B. (1998). Mathematical Classification and Clus-
tering: from How to What and Why. Classification,
Data Analysis, and Data Highways, 690:172–181.
Mourad, R., Sinoquet, C., and Leray, P. (2011). A Hierar-
chical Bayesian Network Approach for Linkage Dise-
quilibrium Modeling and Data-dimensionality Reduc-
tion prior to Genome-wide Association Studies. BMC
Bioinformatics, 12:16+.
Pritchard, J. and Przeworski, M. (2001). Linkage Disequi-
librium in Humans: Models and Data. The American
Journal of Human Genetics, 69(1):1–14.
Purcell, S., Neale, B., Todd-Brown, K., et al. (2007).
PLINK: a Toolset for Whole-genome Association and
Population-based Linkage Analysis. The American
Journal of Human Genetics, 81(3):559–575.
Rand, W. (1971). Objective Criteria for the Evaluation of
Clustering Methods. Journal of the American Statisti-
cal Association, 66(336):846–850.
ModelingGeneticalDatawithForestsofLatentTreesforApplicationsinAssociationGeneticsataLargeScale-Which
ClusteringMethodshouldBeChosen?
15