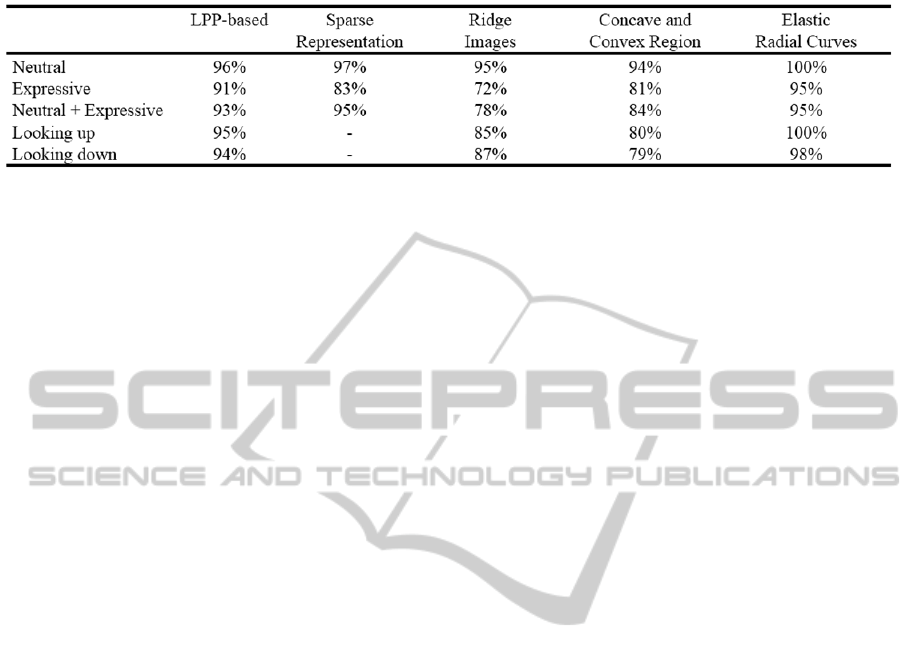
Table 2: Recognition comparison on Gavab database.
7 CONCLUSIONS
An effective 3-D shape matching scheme for pose
and expression-invariant face recognition has been
presented in this paper. The key contribution of the
proposed work is to use isometric embedding shape
representation and statistical modelling techniques to
achieve accurate dense point correspondences and
generate appropriate shapes for new 3-D face data.
From the experimental results on the Gavab and BU-
3DFE database, it can be concluded that the LPP-
based approach offers a recognition rate that can be
as high as nearly 100% and is more expression-
invariant compared with the existing benchmark
approaches. The research will be extended further by
taking into consideration more practical factors. One
possible extension for the work is to evaluate the
ability of the proposed algorithm using more
databases that are produced by different devices
operated under various acquisition environments.
The missing data problem can also be introduced
and dealt with by modifying the shape matching
scheme. Finally, more sophisticated pattern
recognition methods can be applied to increase the
overall performance of the proposed method.
ACKNOWLEDGEMENTS
The work presented in this paper was supported by
the Engineering and Physical Sciences Research
Council (Grant numbers EP/D077540/1 and
EP/H024913/1) and the EU FP7 Project
SEMEOTICONS.
REFERENCES
Berretti, S., Bimbo, A., Pala, P., 2006. 3D face recognition
by modelling the arrangement of concave and convex
regions. Adaptive Multimedia Retrieval, 108-118.
Bronstein, A., Bronstein, M., Kimmel, R., 2005. Three-
dimensional face recognition. International Journal of
Computer Vision, 64(1), 5-30.
Bronstein, A., Bronstein, M., Kimmel, R., 2007.
Expression-invariant representation of faces. IEEE
Transactions on Image Processing, 16(1), 188-197.
Bronstein, M., Bronstein, A., Kimmel, R., Yavneh, I.,
(2006). Multigrid multidimensional scaling.
Numerical Linear Algebra with Applications, 13, 149-
171.
Chang, K., Bowyer, K., Flynn, P., 2003. Multi-modal 2D
and 3D biometrics for face recognition. Proceeding of
IEEE Workshop Analysis and Modelling of Face and
Gestures, 187-194.
Chang, K., Bowyer, K., Flynn, P., 2006. Multiple nose
region matching for 3-D face recognition under
varying facial expression. IEEE Transactions on
Pattern Analysis and Machine Intelligence, 28(10),
1695-1700.
Chellappa, R., Wilson, C., Sirohey, S., 1995. Human and
machine recognition of faces: a survey. Proceeding of
IEEE, 83(5), 705-740.
Chua, C., Han, F., Ho, Y., 2002. 3-D Human face
recognition using point signature. Proceeding of
International Conference Automatic Face and Gesture
Recognition, 233-238.
Drira, H., Amor, B., Mohammed, D., Srivastava, A., 2010.
Pose and expression-invariant 3-D face recognition
using elastic radial curves. British Machine Vision
Conference.
Elad, A., Kimmel, R., 2003. On bending invariant
signatures for surfaces. IEEE Transactions on Pattern
Analysis and Machine Intelligence, 25(10), 1285-
1295.
Hastie, T., Tibshirani, R., Friedman, J., 2001. The
elements of statistical learning. Springer Series in
Statistic, Second Edition, Springer.
Hajati, F., Raie, A., Gao, Y., 2012. 2.5D face recognition
using patch geodesic moments. Pattern Recognition,
45(2012), 969-982.
He, X., Yan, S., Hu, Y., Niyogi, P., Zhang, H., 2005. Face
recognition using Laplacianfaces. IEEE Transactions
on Pattern Analysis and Machine Intelligence. 27(3),
328-340.
Huang, D., Ardabilian, M., Wang, Y., Chen, L., 2012. 3-D
face recognition using eLBP-based facial description
and local feature hybrid matching. IEEE Transactions
3-DShapeMatchingforFaceAnalysisandRecognition
51