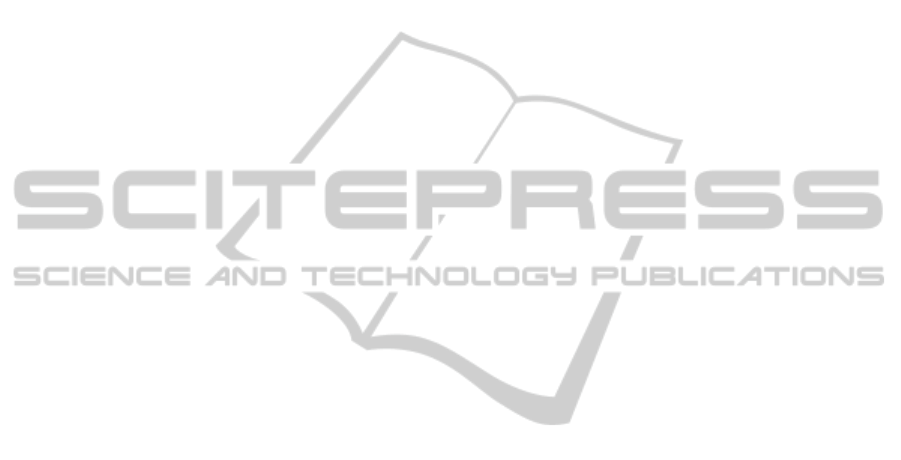
Alzheimer’s disease’ Behav Neurol; vol. 24, no. 2, pp.
149-158.
Grimaldi LM, Casadei VM, Ferri C, Veglia F, Licastro F,
Annoni G, Biunno I, De Bellis G, Sorbi S, Mariani C,
Canal N, Griffin WS, Franceschi M. 2000
‘Association of early-onset Alzheimer's disease with
an interleukin-1alpha gene polymorphism’ Ann
Neurol; vol. 47, no. 3, pp. 361-365.
Grossi E, Mancini A and Buscema M. 2007 ‘International
experience on the use of artificial neural networks in
gastroenterology’ Dig Liver Dis; vol. 39, no. 3, pp.
278-285.
Hamsten A and Eriksson P. 2008 ‘Identifying the
susceptibility genes for coronary artery disease: from
hyperbole through doubt to cautious optimism’ J
Intern Med; vol. 263, no. 5, pp. 538–552.
Ianni M, Callegari S, Rizzo A, Pastori P, Moruzzi P,
Corradi D, Porcellini E, Campo G, Ferrari R, Ferrario
MM, Bitonte S, Carbone I, Licastro F. 2012 ‘Pro-
inflammatory genetic profile and familiarity of acute
myocardial infarction’ Immun Ageing; vol. 9, no. 1,
pp. 14.
Juárez-Herrera Ú, Jerjes-Sánchez C, RENASICA II
Investigators. 2013 ‘Risk factors, therapeutic
approaches, and in-hospital outcomes in Mexicans
with ST-elevation acute myocardial infarction: the
RENASICA II multicenter registry’ Clin Cardiol; vol.
36, no. 5, pp. 241-248.
Kullo IJ and Ding K. 2007 ‘Mechanisms of disease: The
genetic basis of coronary heart disease’ Nat Clin Pract
Cardiovasc Med; vol. 4, no. 10, pp. 558–569.
Lahner E, Intraligi M, Buscema M, Centanni M, Vannella
L, Grossi E, Annibale B. 2008 ‘Artificial Neural
Networks in the Recognition of the Presence of
Thyroid Disease in Patients with Atrophic Body
Gastritis’ World J Gastroenterol; vol. 14, no. 4, pp.
563-568.
Latorra D, Campbell K, Wolter A, Hurley JM. 2003
‘Enhanced allele-specific PCR discrimination in SNP
genotyping using 3' locked nucleic acid (LNA)
primers’ Hum Mutat; vol. 22, no. 1, pp. 79-85.
Levi F, Lucchini F, Negri E, La Vecchia C. 2002 ‘Trends
in mortality from cardiovascular and cerebrovascular
diseases in Europe and other areas of the world’
Heart; vol. 88, no. 22, pp. 119–124.
Li K, Monni S, Hüsing A, Wendt A, Kneisel J, Groß ML,
Kaaks R. 2014 ‘Primary preventive potential of major
lifestyle risk factors for acute myocardial infarction in
men: an analysis of the EPIC-Heidelberg cohort’ Eur J
Epidemiol; vol. 29, no. 1, pp. 27-34.
Licastro F, Chiappelli M, Porcellini E, Campo G,
Buscema M, Grossi E, Garoia F, Ferrari R. 2010
‘Gene-gene and gene - clinical factors interaction in
acute myocardial infarction: a new detailed risk chart’
Current Pharmaceutical Des; vol. 16, no. 7, pp. 783–
788.
Licastro F, Chiappelli M, Caldarera CM, Porcellini E,
Carbone I, Caruso C, Lio D, Corder EH. 2011
‘Sharing pathogenetic mechanisms between acute
myocardial infarction and Alzheimer disease as shown
by partially overlapping of gene variant profiles’ J Alz
Dis; vol. 23, no. 3, pp. 421-431.
Lisboa PJC. 2002 ‘A review of evidence of health benefit
from artificial neural networks in medical
intervention’ Neural Netw; vol. 15, no. 1, pp. 11-39.
Mortensen MB, Sivesgaard K, Jensen HK, Comuth W,
Kanstrup H, Gotzsche O, May O, Bertelsen J, Falk E.
2013 ‘Traditional SCORE-based health check fails to
identify individuals who develop acute myocardial
infarction’ Dan Med J; vol. 60, no. 5, A4629.
Pace F, Riegler G, de Leone A, Pace M, Cestari R,
Dominici P, Grossi E, EMERGE Study Group. 2010
‘Is It Possible to Clinically Differentiate Erosive from
Non erosive Reflux Disease Patients? A Study Using
an Artificial Neural Networks-Assisted Algorithm’
Eur J Gastroenterol Hepatol; vol. 22, no. 10, pp.
1163-1168.
Packard RR and Libby P. 2008 ‘Inflammation in
atherosclerosis: from vascular biology to biomarker
discovery and risk prediction’ Clin Chem; vol. 54, no.
1, pp. 24–38.
Pamukcu B, Lip GY, Devitt A, Griffiths H, Shantsila E.
2010 ‘The role of monocytes in atherosclerotic
coronary artery disease’ Ann Med; vol. 42, no. 6, pp.
394–403.
Patel RS and Ye S. 2011 ‘Genetic determinants of
coronary heart disease: new discoveries and insights
from genome-wide association studies’ Heart; vol. 97,
no. 18, pp. 1463–1473.
Penco S, Grossi E, Cheng S, Intraligi M, Maurelli G,
Patrosso MC, Marocchi A, Buscema M. 2005
‘Assessment of the Role of Genetic Polymorphism in
Venous Thrombosis Through Artificial Neural
Networks’ Ann Hum Genet; vol. 69, no. 6; pp. 693-
706.
Penco S, Buscema M, Patrosso MC, Marocchi A, Grossi
E. 2008 ‘New application of intelligent agents in
sporadic amyotrophic lateral sclerosis identifies
unexpected specific genetic background’ BMC
Bioinformatics; vol. 9, pp. 254.
Ravaglia G, Forti P, Maioli F, Orlanducci P, Sacchetti L,
Flisi E, Dalmonte E, Martignani A, Cucinotta D,
Cavalli G. 2001 ‘Conselice study: a population based
survey of brain aging in a muncipality of the Emilia
Romagna region: (A.U.S.L. Ravenna). Design and
methods’ Arch Gerontol Geriatr Suppl; vol. 7, pp.
313-324.
Ridker PM, Rifai N, Pfeffer M, Sacks F, Lepage S,
Braunwald E. 2000 ‘Elevation of tumor necrosis
factor-alpha and increased risk of recurrent coronary
events after myocardial infarction’ Circulation; vol.
101, no. 18, pp. 2149–2153.
Rotondano G, Cipolletta L, Grossi E, Koch M, Intraligi M,
Buscema M, Marmo R; Italian Registry on Upper
Gastrointestinal Bleeding (Progetto Nazionale
Emorragie Digestive). 2011 ‘Artificial Neural
Networks Accurately Predict Mortality in Patients
with Non variceal Upper GI Bleeding’ Gastrointest
Endoscop; vol. 73, no. 2, pp. 218-226.
Rumelhart DE and McClelland JL. 1982 ‘An interactive
HEALTHINF2015-InternationalConferenceonHealthInformatics
258