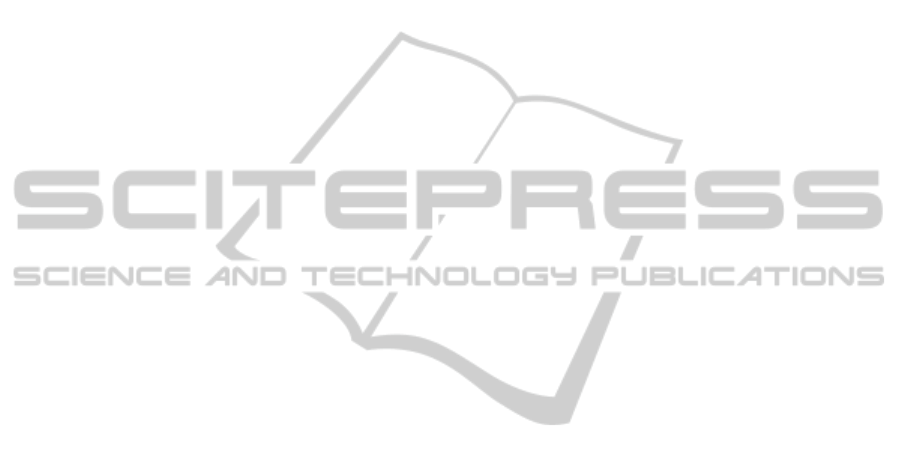
5 CONCLUSIONS
In this paper we proposed two exploration approaches
based on the query reformulation and the Nearest
Neighbor paradigms. The main goal attained by the
proposed mechanisms is to be able to explore the fea-
ture space around the images labeled as being rel-
evant by the user, thus following the user’s explo-
rative behavior during a session of image search into
a visual database. Reported results show that the
proposed approach succeeded in showing the user a
greater number of new relevant images during the first
few iterations, in comparison with other techniques
either based on “classification”, or “exploration” ap-
proaches. We believe that the effectiveness of CBIR
systems strongly depends on its adaptive behavior in
response to relevance feedback. Accordingly, fur-
ther experiments aimed at testing the system with real
users are needed in order to assess the effectiveness
of the proposed approach compared to other state-of-
the-art relevance feedback mechanisms.
ACKNOWLEDGEMENTS
We would like to thank Dr. M. Arevalillo-Herrez
and Dr. F. J. Ferri for providing us with the details
of the results reported in (Arevalillo- Herra ez and
Ferri, 2013). This work has been partly supported
by the Regional Administration of Sardinia (RAS),
Italy, within the project Advanced and secure sharing
of multimedia data over social networks in the future
Internet (CRP 17555, L.R. 7/2007, Bando 2009).
REFERENCES
Arevalillo-Herr
´
aez, M. and Ferri, F. J. (2010). Interactive
image retrieval using smoothed nearest neighbor esti-
mates. In Hancock et al. ed., SSPR/SPR, LNCS 6218,
pp 708–717. Springer.
Arevalillo-Herr
´
aez, M. and Ferri, F. J. (2013). An improved
distance-based relevance feedback strategy for image
retrieval. Image Vision Comput., 31(10):704–713.
Arevalillo-Herr
´
aez, M., Ferri, F. J., and Domingo, J. (2010).
A naive relevance feedback model for content-based
image retrieval using multiple similarity measures.
Pattern Recognition, 43(3):619–629.
Boiman, O., Shechtman, E., and Irani, M. (2008). In de-
fense of nearest-neighbor based image classification.
In CVPR 2008. IEEE Computer Society.
Chen, Y., Zhou, X. S., and Huang, T. (2001). One-class
svm for learning in image retrieval. In ICIP 2001,
volume 1, pp 34 –37.
Cohn, D. A., Atlas, L. E., and Ladner, R. E. (1994). Im-
proving generalization with active learning. Machine
Learning, 15(2):201–221.
Datta, R., Joshi, D., Li, J., and Wang, J. Z. (2008). Image
retrieval: Ideas, influences, and trends of the new age.
ACM Computing Surveys, 40(2):1–60.
Ding, C. H. Q. and He, X. (2004). K-nearest-neighbor con-
sistency in data clustering: incorporating local infor-
mation into global optimization. In Haddad et al. ed.,
SAC 2004, pp 584–589. ACM.
Garc
´
ıa, S., Herrera, F., and Shawe-taylor, J. An extension
on “statistical comparisons of classifiers over multiple
data sets” for all pairwise comparisons. Journal of
Machine Learning Research, pp 2677–2694.
Giacinto, G. (2007). A nearest-neighbor approach to rele-
vance feedback in content based image retrieval. In
CIVR ’07, pp 456–463, New York, NY, USA. ACM.
Giacinto, G. and Roli, F. (2004a). Bayesian relevance
feedback for content-based image retrieval. Pattern
Recognition, 37(7):1499–1508.
Giacinto, G. and Roli, F. (2004b). Nearest-prototype rele-
vance feedback for content based image retrieval. In
ICPR 2004, pp 989–992.
Hoi, S. C. H., Jin, R., Zhu, J., and Lyu, M. R. (2009).
Semisupervised svm batch mode active learning with
applications to image retrieval. ACM Trans. Inf. Syst.,
27(3):16:1–16:29.
Laaksonen, J., Koskela, M., and Oja, E. (2002). PicSOM-
self-organizing image retrieval with MPEG-7 content
descriptors. IEEE Transactions on Neural Networks,
13(4):841–853.
Lew, M. S., Sebe, N., Djeraba, C., and Jain, R. (2006).
Content-based multimedia information retrieval: State
of the art and challenges. ACM Trans. Multimedia
Comput. Commun. Appl., 2(1):1–19.
Lindenbaum, M., Markovitch, S., and Rusakov, D. (2004).
Selective sampling for nearest neighbor classifiers.
Machine Learning, 54(2):125–152.
Pavlidis, T. (2008). Limitations of content-based image re-
trieval. Technical report, Stony Brook University.
Piras, L., Giacinto, G., and Paredes, R. (2012). Enhanc-
ing image retrieval by an exploration-exploitation ap-
proach. In Perner, P. ed., MLDM 2012, LNCS 7376,
pp 355–365. Springer.
Rao, Y., Mundur, P., and Yesha, Y. (2006). Fuzzy svm en-
sembles for relevance feedback in image retrieval. In
Sundaram et al. ed., CIVR 2006, LNCS 4071, pp 350–
359. Springer.
Rocchio, J. J. (1971). Relevance feedback in information re-
trieval. In Salton, G. ed., The SMART Retrieval System
- Experiments in Automatic Document Processing, pp
313–323. Prentice Hall, Englewood, Cliffs, New Jer-
sey.
Rui, Y., Huang, T. S., and Mehrotra, S. (1997). Content-
Based image retrieval with relevance feedback in
MARS. In International Conference on Image Pro-
cessing Proceedings, pp 815–818.
Sivic, J. and Zisserman, A. (2008). Efficient visual search
for objects in videos. Proceedings of the IEEE,
96(4):548 –566.
ICPRAM2015-InternationalConferenceonPatternRecognitionApplicationsandMethods
188