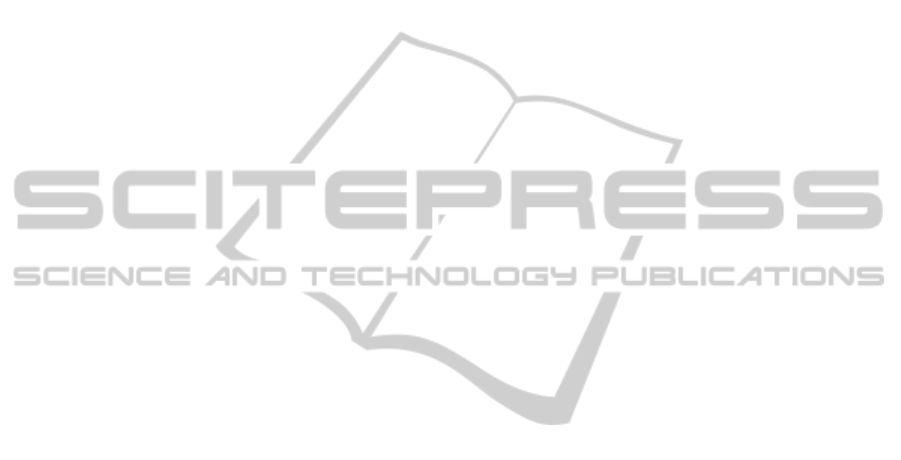
is considered as a physician expert in the treatment
of a single disease, and is represented by an agent
with hierarchical planning capabilities. The result is
obtained through the coordination of all the agents,
and respects the recommendations of each guideline.
Riaño et al. represent guidelines as sets of
clinical actions that are modelled into an ontology
(López-Vallverdú et al. 2013). To combine two
treatments, first they are unified in a unique
treatment and then a set of “combination rules” is
applied to detect and avoid possible interactions. A
model-based automatic merge of CIGs is then
purposed in (Riaño and Collado 2013), through the
definition of a combining operator. Jafarpour and
Abidi (Jafarpour and Abidi 2013) use semantic-web
rules and an ontology for the merging criteria. Given
these, an Execution Engine dynamically merges
several CIGs according to merge criteria. GLINDA
proposes a wide ontology of cross-guideline
interactions (http://glinda-project.stanford.edu/guide
lineinteractionontology.html). We recently proposed
an original approach, supporting user-driven and
interactive interaction detection over different levels
of abstractions (Piovesan et al. 2014).
However, although interactions can only occur in
time, to the best of our knowledge no previous
approach to the treatment of interactions (and
comorbidities) has already provided facilities to
address the temporal dimension. This is the goal of
the approach in this paper, in which we proposed a
general approach, suitable in different situations
(e.g., either in case a specific comorbid patient is
considered, or in case “abstract” possible
interactions between CIGs are taken into account),
and providing a wide range of facilities to user-
physicians.
Temporal issues are pervasive in the CIG context
and many previous approaches have faced some of
them (see, e.g., the survey in (Terenziani et al.
2008)). In particular, in the Asbru (Shahar et al.
1998) and in the GLARE (Anselma et al. 2006)
projects, rich representation formalisms have been
proposed to cope with temporal constraints in the
CIGs, and in GLARE correct and complete temporal
constraint propagation algorithms have been
proposed to reason with them and to merge them
with the time of execution of actions on specific
patients (Anselma et al. 2006). However, to the best
of our knowledge, no other approach to CIGs has
explicitly addressed the treatment of time and
temporal constraints for the detection of CIG
interactions. In this sense, we believe that our
approach, besides being innovative, is somehow
complementary with respect to several other
approaches in the literature, so that an integration
with them can be devised as a future work (e.g., with
Riaño’s methodology to merge CIGs (Riaño and
Collado 2013)).
We are currently developing a prototypical
implementation to demonstrate our approach, based
on GLARE. In our short-term future work, we aim at
extending our approach to cope also with cases not
covered in Table 1. In our long-term future work, we
will attempt to support physicians also in the
interaction solving, and, finally, in merging multiple
guidelines in the treatment of a specific patient.
ACKNOWLEDGEMENTS
The work described in paper was partially supported
by Compagnia di San Paolo, in the Ginseng project.
REFERENCES
Allen, J.F., 1983. Maintaining knowledge about temporal
intervals. Communications of the ACM, 26(11),
pp.832–843.
Anselma, L. et al., 2006. Towards a comprehensive
treatment of repetitions, periodicity and temporal
constraints in clinical guidelines. Artificial Intelligence
in Medicine, 38(2), pp.171–195.
Brusoni, V. et al., 1997. Later: managing temporal
information efficiently. IEEE Expert, 12(4), pp.56–64.
Dechter, R., Meiri, I. and Pearl, J., 1991. Temporal
Constraint Networks. Artif. Intell., 49(1-3), pp.61–95.
Fridsma, D.B., 2001. Special Issue on Workflow
Management and Clinical Guidelines. Journal of the
American Medical Informatics Association, 22(1).
Gordon, C. and Christensen, J.P. eds., 1995. Health
telematics for clinical guidelines and protocols,
Amsterdam, Netherlands: IOS Press.
Horvitz, E., 1999. Uncertainty, Action, and Interaction: In
Pursuit of Mixed-Initiative Computing. IEEE
Intelligent Systems, 14(5), pp.17–20.
Jafarpour, B. and Abidi, S.S.R., 2013. Merging Disease-
Specific Clinical Guidelines to Handle Comorbidities
in a Clinical Decision Support Setting. In AIME. pp.
28–32.
López-Vallverdú, J.A., Riaño, D. and Collado, A., 2013.
Rule-Based Combination of Comorbid Treatments for
Chronic Diseases Applied to Hypertension, Diabetes
Mellitus and Heart Failure. In R. Lenz et al., eds.
Process Support and Knowledge Representation in
Health Care. Lecture Notes in Computer Science.
Springer Berlin Heidelberg, pp. 30–41.
Michalowski, M. et al., 2013. Using Constraint Logic
Programming to Implement Iterative Actions and
Numerical Measures during Mitigation of
Concurrently Applied Clinical Practice Guidelines. In
TemporalDetectionofGuidelineInteractions
49