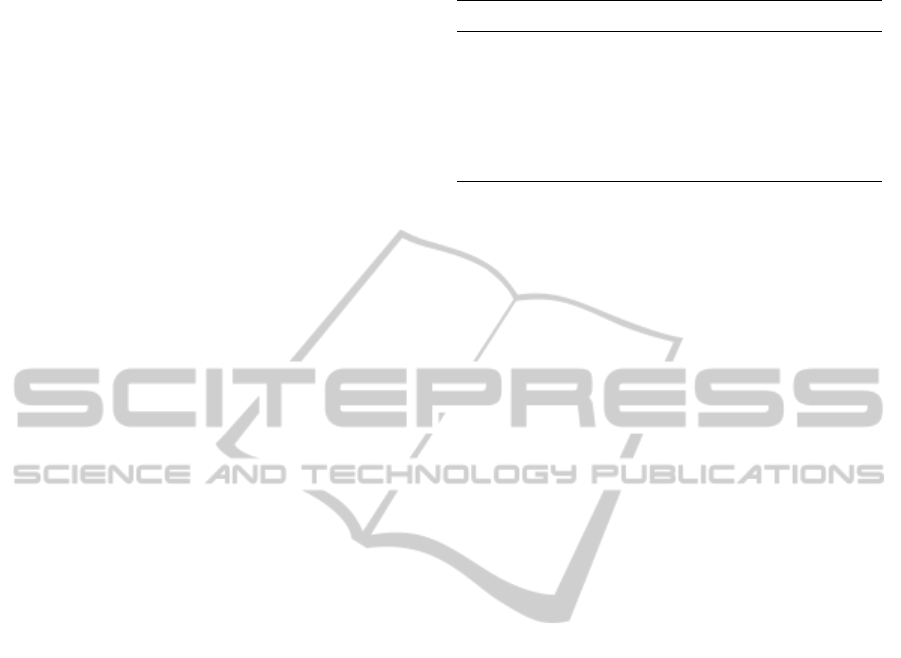
artifacts from measured data and recognize when beat
detection is impaired or not possible. Validation of the
method on ballistocardiogram measurements of 12
healthy subjects showed 4.7% average beat-detection
error rate. The presented algorithm lends itself to ap-
plication on a multitude of almost periodic signals,
especially in cardiology.
Currently, the algorithm at first adapts itself to a
specific BCG signal, as described in Section 2. It
is likely that alterations of the system (e.g., changed
body position) will trigger the likelihood-based arti-
fact detection routine (see Section 2) and a new sys-
tem identification step will become necessary. How-
ever, in the present dataset, subjects were laying still
and this issue was not observed.
A further complication, which was also not ob-
served in the current dataset, may arise when strong
heart-rate variability occurs. The window-based ap-
proach may either select a window where no beat
is present or a window with two beats. In the first
case, a false-positive beat will be detected, while in
the other case, the algorithm will miss a beat and
make a false-negative error. This issue could be ad-
dressed by considering the relative likelihood value
of a beat estimate to the other indices with large like-
lihood values and deciding if two beats are present or
none. Also schemes which run the window-based de-
tection schemes multiple times and each time includ-
ing the currently detected beats and models on heart-
rate variability might mitigate these type of errors.
Blind system identification i.e., estimation of the
state-space model solely on BCG measurements,
would facilitate applicability of the current method.
As a consequence, the resulting method would not
require ECG measurement equipment and would be
able to adapt to varying external factors (e.g., sleeping
position). Moreover, investigating on the relation be-
tween the estimated input magnitude and the instan-
taneous cardiac output, which is a key biomarker for
various cardiovascular diseases, seems to be a promis-
ing direction for future research.
A further enhancement can be achieved by multi-
plying the hypothesis likelihood with a Gaussian win-
dow, centered around the assumed input, serving as
a prior on the heart beat position. This refinement
significantly improves error rates, when the BCG sig-
nal is hardly contaminated by movement artifacts. On
the other hand when the signal contains several move-
ment artifacts, it is better not to assume any prior on
the beat position to avoid error propagation. To deal
with a general BCG signal, possibly heavily contam-
inated with artifacts, the Gaussian window was omit-
ted in the performance evaluation in subsection 4.
Table 1: Performance metrics.
Metric Unit Value
Signal duration min 10
Coverage 6-σ labeling % 95
Coverage likelihood drop labeling % 90
HB detection error rate % 4.7
HB interval error ms 33.1
ACKNOWLEDGEMENTS
The authors would like to thank Nour Zalmai for valu-
able feedback concerning the sparse-input learning al-
gorithm. Furthermore we highly appreciated the valu-
able discussions with Dr. med. Reto Wildhaber re-
garding the cardiovascular system.
REFERENCES
Alihanka, J., Vaahtoranta, K., and Saarikivi, I. (1981). A
new method for long-term monitoring of the ballisto-
cardiogram, heart rate, and respiration. Am J Physiol,
240(5):R384–92.
Bruser, C., Stadlthanner, K., de Waele, S., and Leonhardt,
S. (2011). Adaptive beat-to-beat heart rate estimation
in ballistocardiograms. IEEE Transactions on Infor-
mation Technology in Biomedicine,, 15(5):778–786.
Cathcart, R. T., Field, W. W., and Richards Jr, D. W. (1953).
Comparison of cardiac output determined by the bal-
listocardiograph (nickerson apparatus) and by the di-
rect fick method. Journal of Clinical Investigation,
32(1):5.
Friedrich, D., Aubert, X., Fuhr, H., and Brauers, A.
(2010). Heart rate estimation on a beat-to-beat ba-
sis via ballistocardiography-a hybrid approach. In En-
gineering in Medicine and Biology Society (EMBC),
2010 Annual International Conference of the IEEE,
pages 4048–4051. IEEE.
Inan, O., Etemadi, M., Paloma, A., Giovangrandi, L., and
Kovacs, G. (2009). Non-invasive cardiac output trend-
ing during exercise recovery on a bathroom-scale-
based ballistocardiograph. Physiological measure-
ment, 30(3):261.
Kschischang, F. R., Frey, B. J., and Loeliger, H.-A. (2001).
Factor graphs and the sum-product algorithm. In-
formation Theory, IEEE Transactions on, 47(2):498–
519.
Kurumaddali, B., Marimuthu, G., Venkatesh, S. M., Suresh,
R., Syam, B., and Suresh, V. (2014). Cardiac output
measurement using ballistocardiogram. In The 15th
International Conference on Biomedical Engineering,
pages 861–864. Springer.
Ljung, L. (1998). System identification. Springer.
Loeliger, H.-A., Dauwels, J., Hu, J., Korl, S., Ping, L., and
Kschischang, F. R. (2007). The factor graph approach
BIOSIGNALS2015-InternationalConferenceonBio-inspiredSystemsandSignalProcessing
28