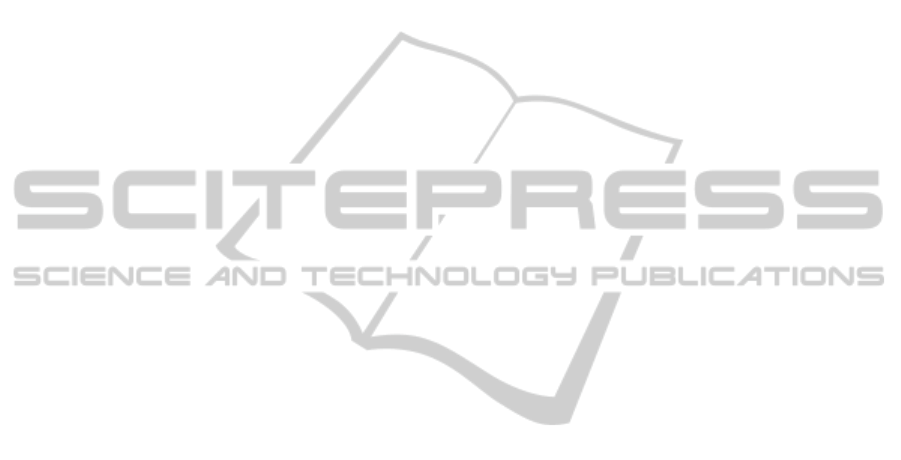
which including three processes, i.e. preprocessing,
getting MA candidates, and screening MAs. The PC
model is used to get MAs candidates. The obtained
MAs candidates are very near to the true MAs,
which give the good basis for next processing. Then,
the irrelevant information, such as the vessel
fragments, is removed by constructing directional
cross-section profiles. This approach is invariant to
image contrast and brightness, which needs no
enhancement processing. The experiments results on
50 images provided by ROC website show that this
method can accurately detect microaneurysms in
color fundus images.
ACKNOWLEDGEMENTS
This work was supported by the National Nature
Science Foundation of China (NSFC) under grant
No. 61102150 and the Tianjin Science and
Technology Supporting Projection under grant No.
13ZCZDGX02100.
REFERENCES
Spencer, T., Olson, J. A., McHardy, K. C., et al, 1996. An
image-processing strategy for the segmentation and
quantification of microaneurysms in fluorescein
angiograms of the ocular fundus [J]. Computers and
Biomedical Research, 29(4): 284-302.
Hipwell, J. H., Strachant, F., Olson, J. A., et al, 2000.
Automated detection of microaneurysms in digital red-
free photographs: A diabetic retinopathy screening
tool [J]. Diabetic Medicine, 17(8): 588-594.
Fleming, A. D., Philip, S., Goatman, K. A., et al, 2006.
Automated microaneurysm detection using local
contrast normalization and local vessel detection [J].
IEEE Transactions on Medical Imaging, 25(9):1223-
1232.
Walter, T., Massin, P., Erginay, A., et al, 2007. Automatic
detection of microaneurysms in color fundus images
[J]. Medical Image Analysis, 11(6): 555-566.
Sinthanayothin, C., Boyce, J. F., Williamson, T. H., et al,
2002. Automated detection of diabetic retinopathy on
digital fundus images [J]. Diabetic Medicine,
19(2):105-112.
Niemeijer, M., Ginneken, B., Staal, J., et al, 2005.
Automatic detection of red lesions in digital color
fundus photographs [J]. IEEE Transactions on
Medical Imaging, 24(5): 584-592.
Zhang, B., Karray, F., Zhang, L., et al, 2010.
Microaneurysm (MA) detection via sparse
representation classifier with MA and Non-MA
dictionary learning [C]. In IEEE International
Conference on Pattern Recognition, 277-280.
Quellec, G., Lamard, M., Josselin, P. M., et al, 2008.
Optimal wavelet transform for the detection of
microaneurysms in retina photographs [J]. IEEE
Transactions on Medical Imaging, 27(9): 1230-1241.
Hatanaka, Y., Inoue, T., Okumura, S., et al, 2012.
Automated microaneurysm detection method based on
double-ring filter and feature analysis in retinal fundus
images[C]. In 25th International Symposium on
Computer-Based Medical Systems. Rome, 1-4.
Morrone, M. C., Owens, R. A., 1987. Feature detection
from local energy [J]. Pattern Recognition Letters,
6(5): 303-313.
Xiao, Z. T., Hou, Z. X., 2004. Phase based feature detector
consistent with human visual system characteristics
[J]. Pattern Recognition Letters, 25(10): 1115-1121.
Kovesi, P., 1999. Image features from phase congruency
[J]. Journal of Computer Vision Research, 1(3): 1-26.
Lazar, I., Hajdu, A., 2013. Retinal Microaneurysm
detection through local rotating cross-section profile
analysis [J]. IEEE Transactions on Medical Imaging,
32(2): 400-407.
Retinopathy Online Challenge, 2008. http://roc.health-
care.uiowa.edu/.
Gao, W. W., Shen, J. X., Wang, Y. L., 2012. Efficient and
automated detection of microaneurysms from non-
dilated fundus images [J]. Chinese Journal of
Biomedical Engineering, 31(6):839-845.
UsingPhaseCongruencyModelforMicroaneurysmsDetectioninFundusImage
163